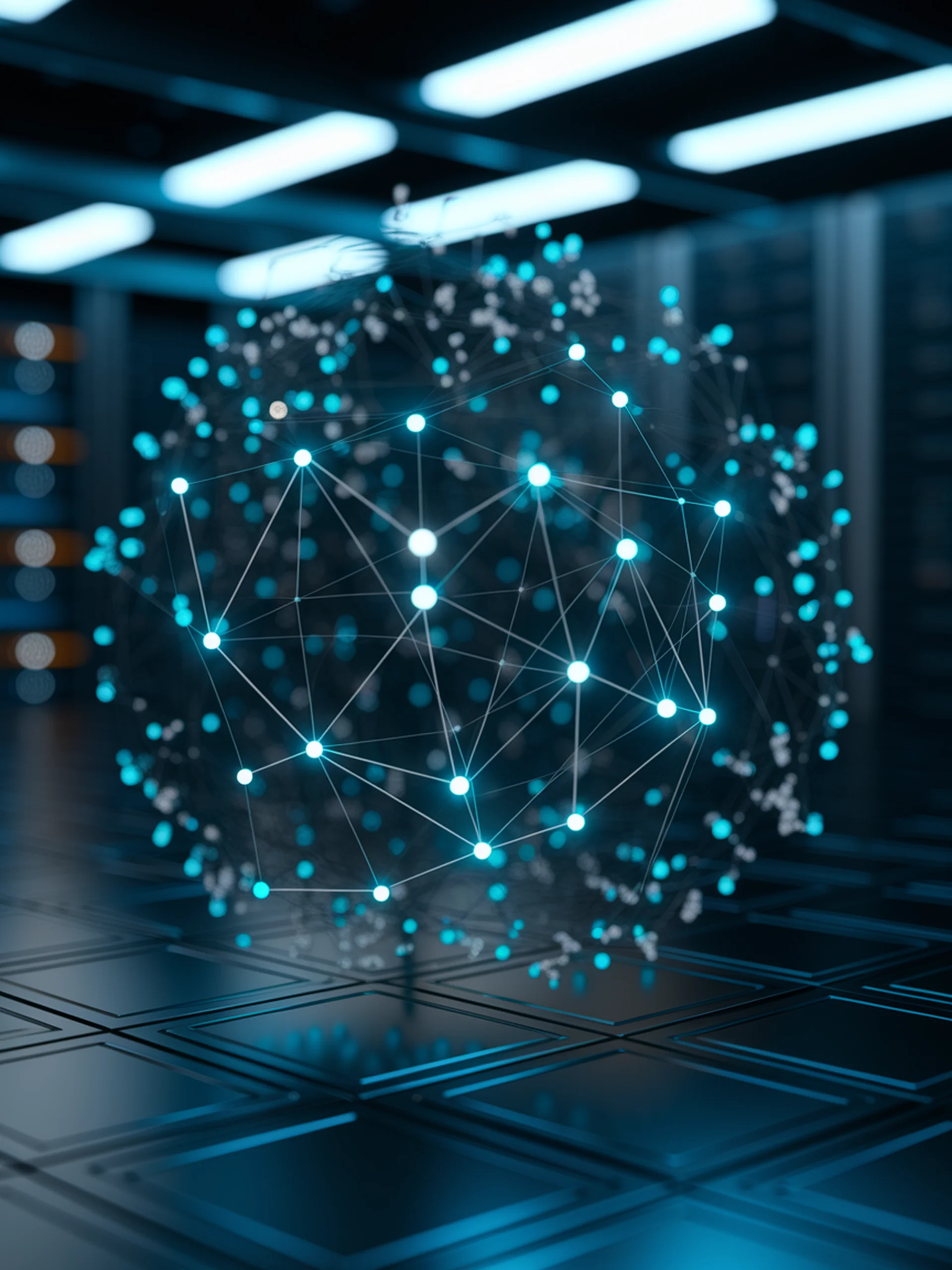
Behavioral Control of LLMs at Runtime
Tuning Model Behavior Without Additional Training
The Mixture-of-Tunable-Experts (MoTE) approach allows for precise control over LLM behavior during inference without requiring retraining or fine-tuning.
- Uses functional Token Resonance Imaging (fTRI) to analyze neural activation patterns in DeepSeek-R1
- Enables on-demand modification of model responses and refusal behaviors
- Provides granular control over model outputs by manipulating expert weighting
- Creates more predictable and customizable AI systems with existing models
From a security perspective, this research offers a significant advancement in controlling potentially harmful outputs and tailoring refusal rates for sensitive content, enhancing model safety without compromising capability.
Mixture of Tunable Experts -- Behavior Modification of DeepSeek-R1 at Inference Time