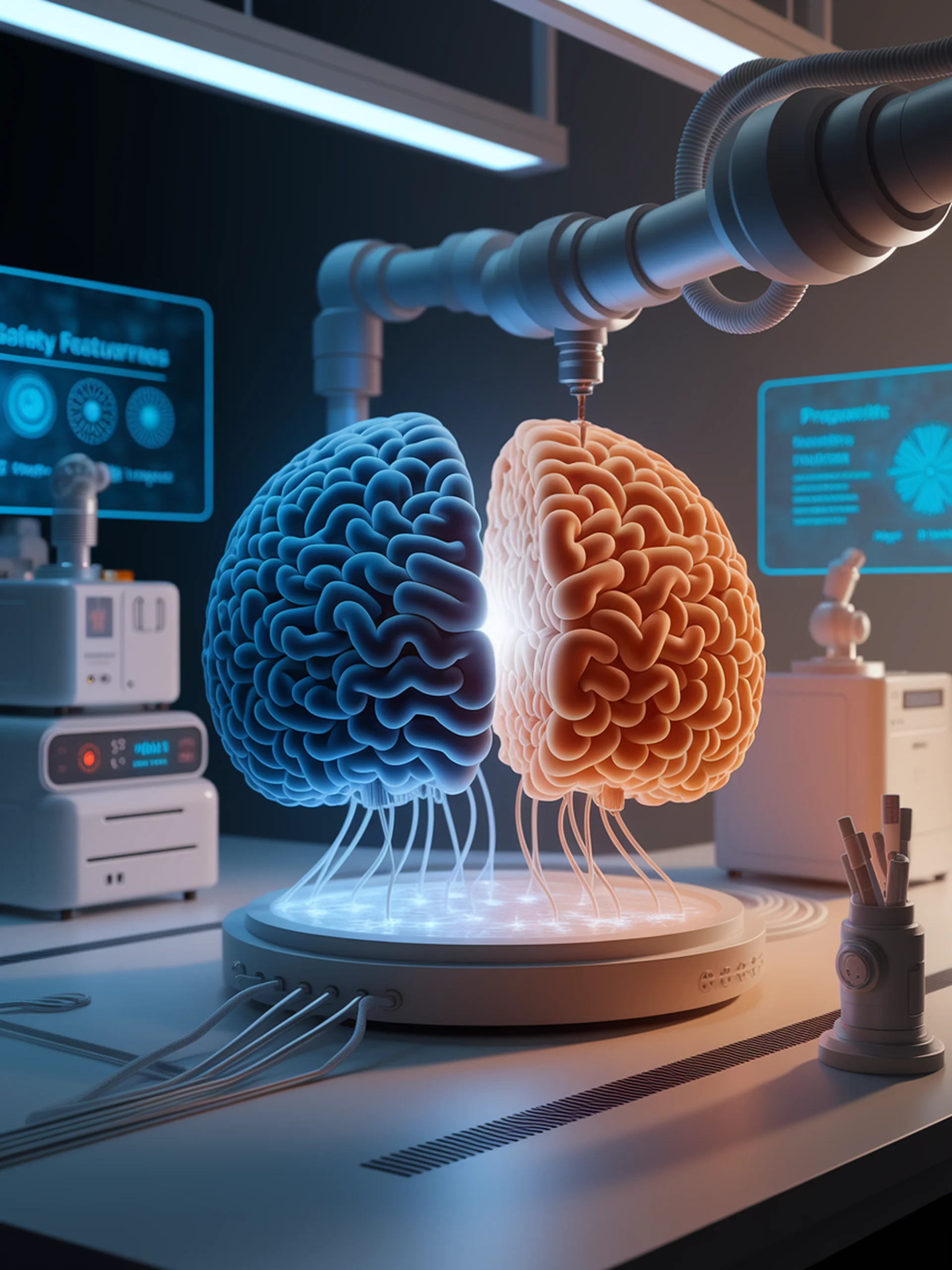
Safer Model Merging for LLMs
Resolving Safety-Utility Conflicts in LLM Integration
LED-Merging is a novel technique that resolves the critical safety-utility trade-off when combining multiple fine-tuned language models, preserving both specialized capabilities and safety guardrails.
- Addresses neuron misidentification and cross-task interference problems that cause safety degradation in traditional model merging
- Uses innovative location-election-disjoint approach to intelligently select and integrate neurons across models
- Achieves 41% reduction in harmful responses while maintaining or improving performance on utility tasks
- Requires zero additional training, offering a computationally efficient solution for LLM deployment
This research is particularly valuable for security teams working with LLMs, as it enables the integration of specialized capabilities without compromising safety guardrails that prevent harmful outputs.
LED-Merging: Mitigating Safety-Utility Conflicts in Model Merging with Location-Election-Disjoint