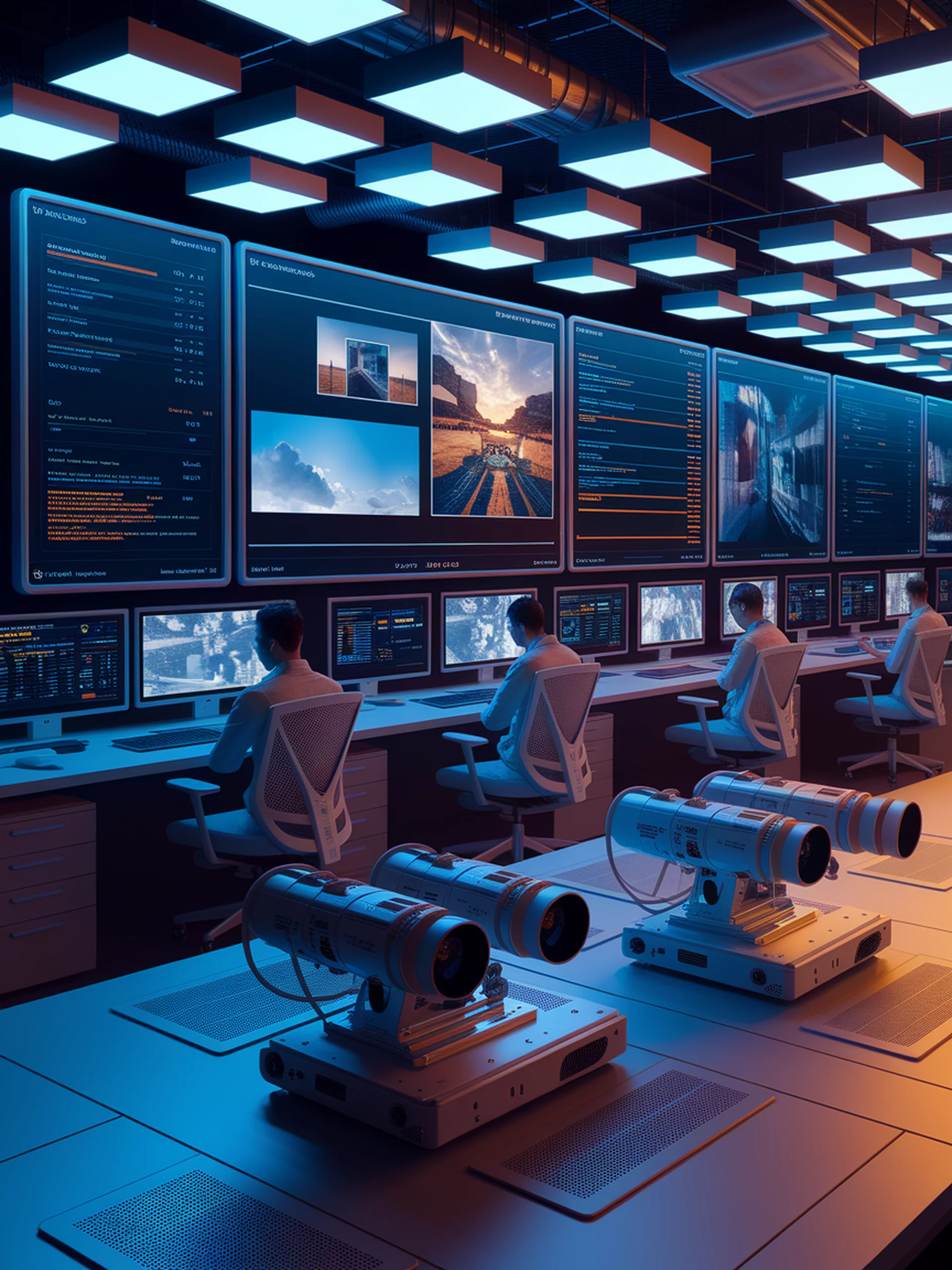
Leveraging MLLMs for Image Safety
A human-free approach to detecting unsafe visual content
This research introduces a framework using Multimodal Large Language Models to judge image safety without requiring human-labeled data.
- Proposes a novel MLLM-as-a-Judge approach that evaluates image safety across diverse safety policies
- Demonstrates performance comparable to models trained with human-labeled datasets
- Creates a comprehensive evaluation benchmark for image safety judgment
- Enables adaptable, configurable safety filtering without extensive retraining
This innovation addresses critical security needs for content moderation systems and AI image generators, providing an efficient, scalable method to identify potentially harmful visual content without human exposure to unsafe materials.