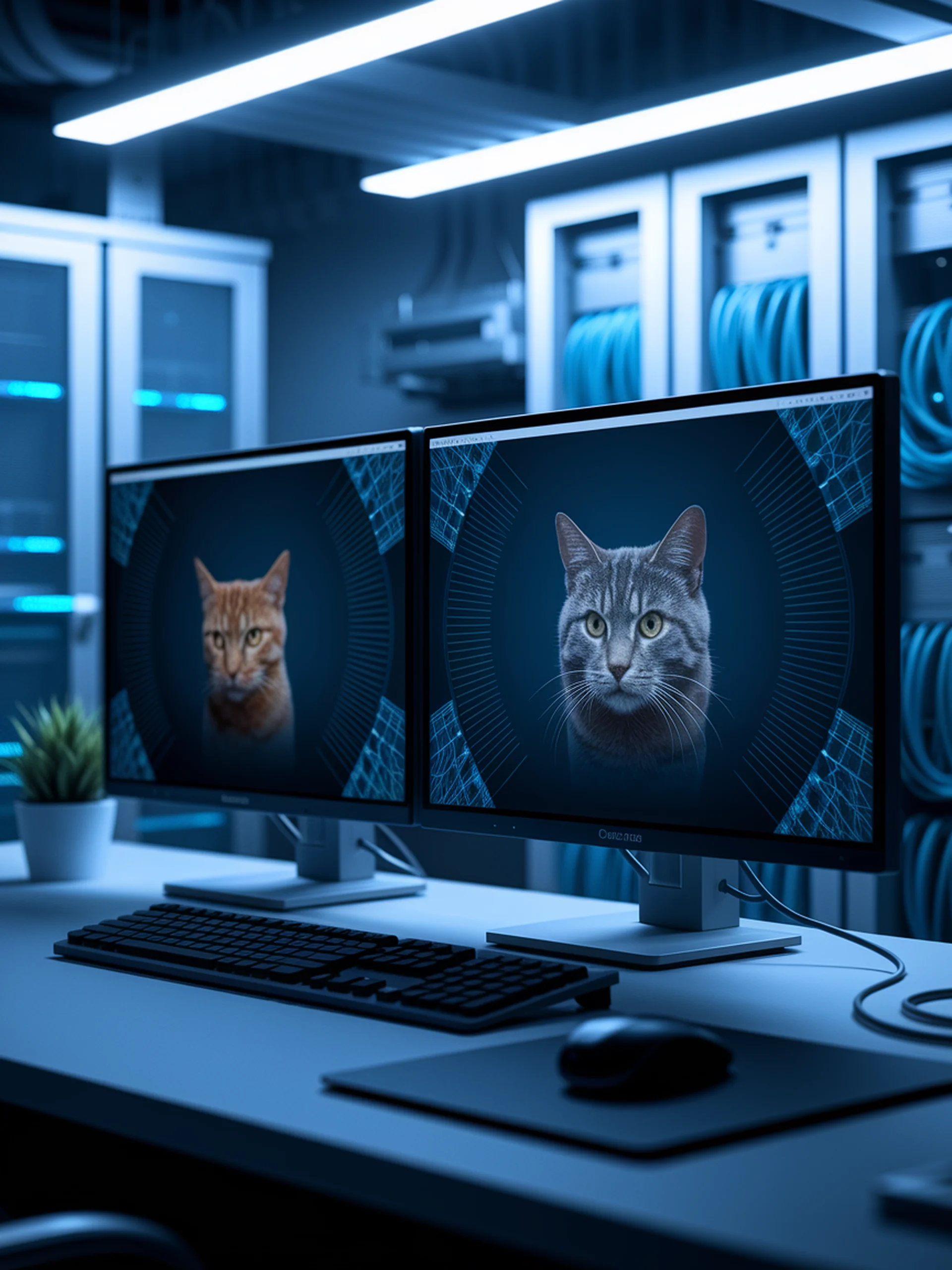
The Gaslighting Vulnerability in Multimodal AI
How negation arguments can trick advanced vision-language models
This research reveals a critical security vulnerability in Multimodal Large Language Models (MLLMs) that allows attackers to manipulate model responses through simple negation techniques.
- Models frequently accept contradictions to their initially correct answers when faced with negation arguments
- Researchers developed GaslightingBench, a specialized benchmark to evaluate model robustness
- Even state-of-the-art MLLMs show significant performance drops when challenged with negation
- These findings highlight urgent security concerns for multimodal AI systems in high-stakes applications
For security professionals, this research emphasizes the need for more robust defenses against conversational manipulation in multimodal systems before deployment in critical environments.
Original Paper: Calling a Spade a Heart: Gaslighting Multimodal Large Language Models via Negation