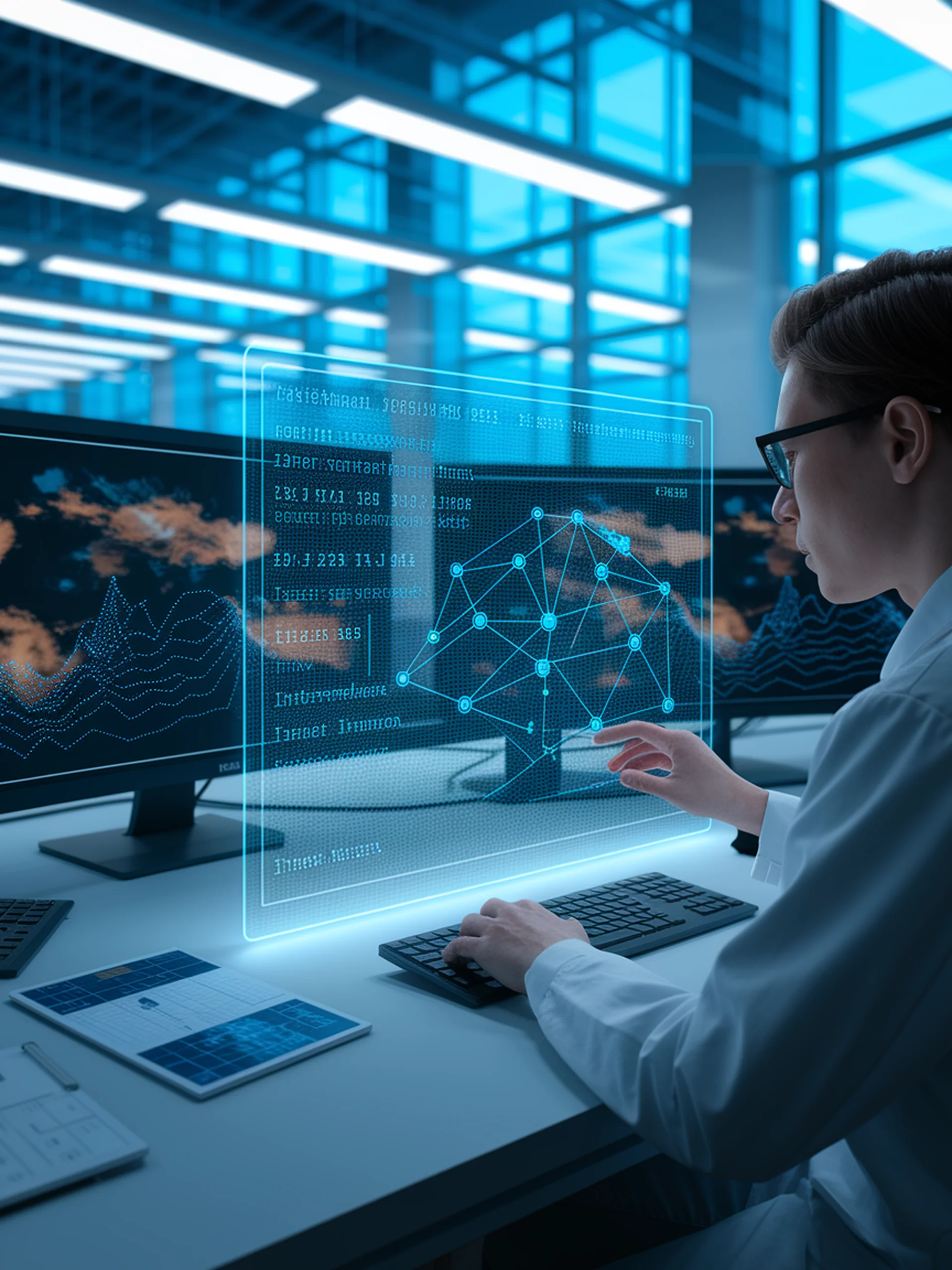
Predicting MLLM Reliability Under Shifting Conditions
A New Information-Theoretic Framework for Quantifying MLLM Risks
This research introduces the first formal framework to quantify the risks of Multimodal Large Language Models (MLLMs) when faced with distribution shifts.
- Proposes an information-theoretic approach to measure how MLLMs perform when test data differs from training data
- Establishes a mathematical foundation for predicting MLLM failure scenarios
- Addresses the critical need for safety guarantees before MLLMs can be widely deployed
- Enables more reliable risk assessment in real-world applications with unpredictable inputs
For security professionals, this framework offers a systematic method to evaluate MLLM vulnerabilities before deployment in critical systems, potentially preventing costly failures or security breaches.
Understanding Multimodal LLMs Under Distribution Shifts: An Information-Theoretic Approach