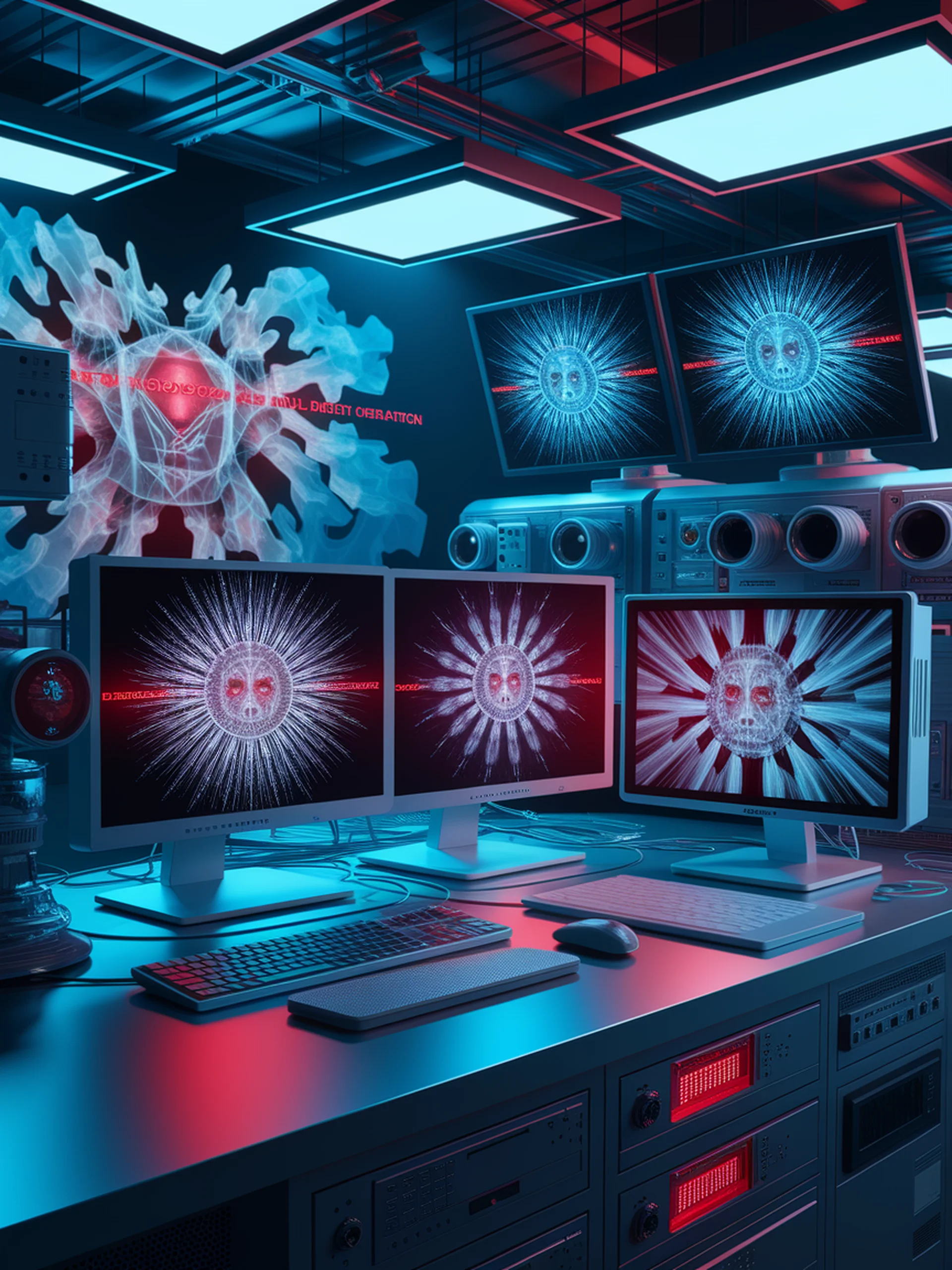
Exploiting Visual Distractions in MLLMs
How complexity of visuals can bypass AI safety guardrails
This research reveals critical security vulnerabilities in Multimodal Large Language Models through a novel attack framework that exploits how MLLMs process complex visual inputs.
- Discovered that image complexity rather than content is the key factor in successful jailbreaking attempts
- Developed the CS-DJ framework that systematically exploits these vulnerabilities to bypass safety mechanisms
- Demonstrated effectiveness across multiple commercial MLLMs through extensive testing
- Highlights urgent need for robust multimodal safety measures as these models become more widely deployed
This work matters because it exposes fundamental design weaknesses in how current MLLMs handle the interplay between visual and text inputs, creating potential risks for commercial applications where users could bypass content moderation.
Distraction is All You Need for Multimodal Large Language Model Jailbreaking