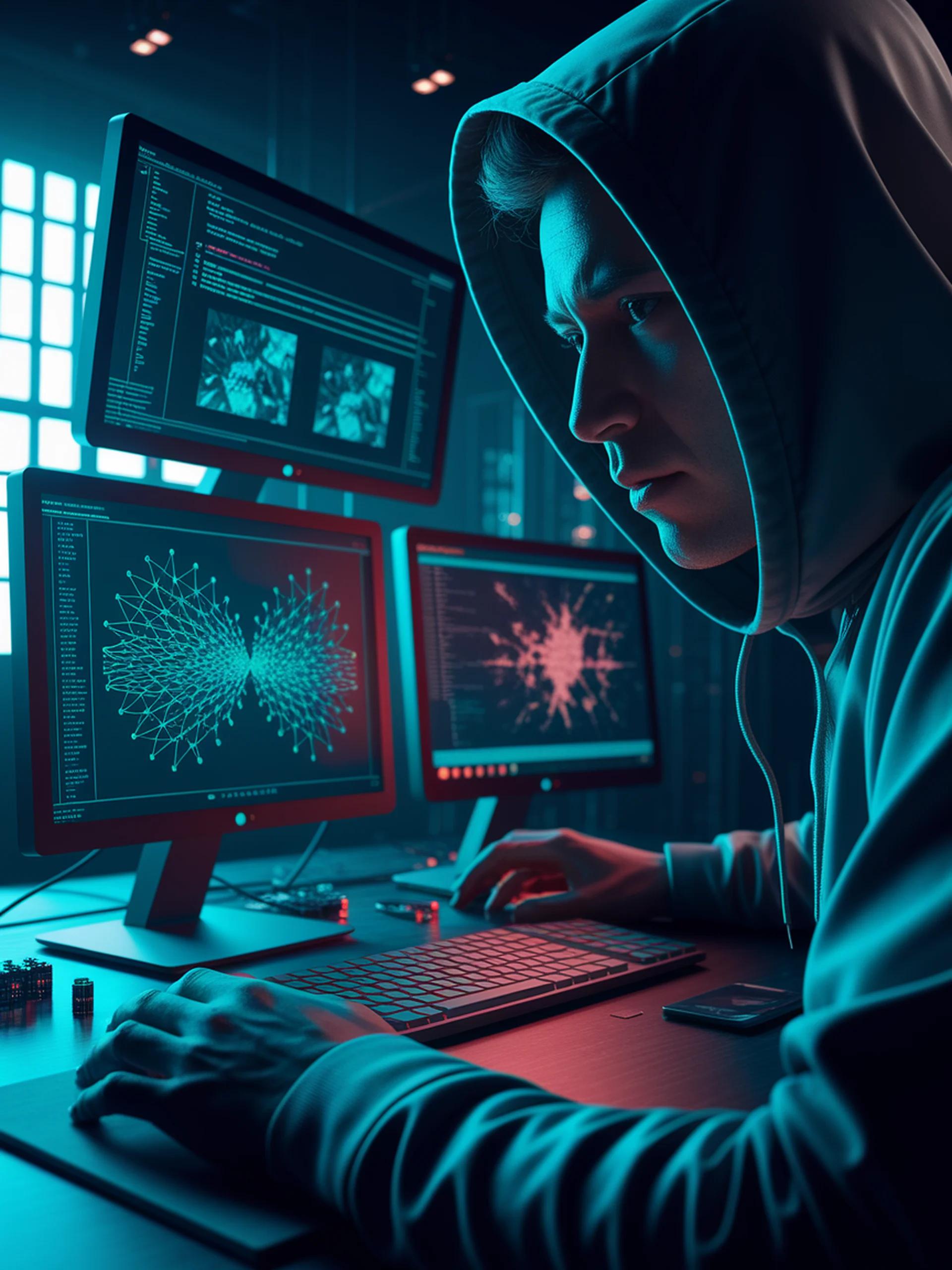
Exploiting the Blind Spots of MLLMs
A Dynamic Approach to Transfer Adversarial Attacks Across Models
This research introduces a novel attack method that significantly improves the transferability of adversarial examples between different Multimodal Large Language Models (MLLMs).
- Proposes Dynamic Vision-Language Alignment Attack (DVLA) that achieves up to 3.17× higher transfer success rates compared to previous methods
- Focuses on exploiting the vision-language alignment process rather than just vision-specific perturbations
- Reveals fundamental vulnerabilities in how MLLMs connect visual and language components
- Demonstrates attack effectiveness across popular models including LLaVA, MiniGPT-4, and InstructBLIP
This research highlights critical security concerns as MLLMs are increasingly deployed in production environments, showing that even models with different architectures share exploitable weaknesses.
Improving Adversarial Transferability in MLLMs via Dynamic Vision-Language Alignment Attack