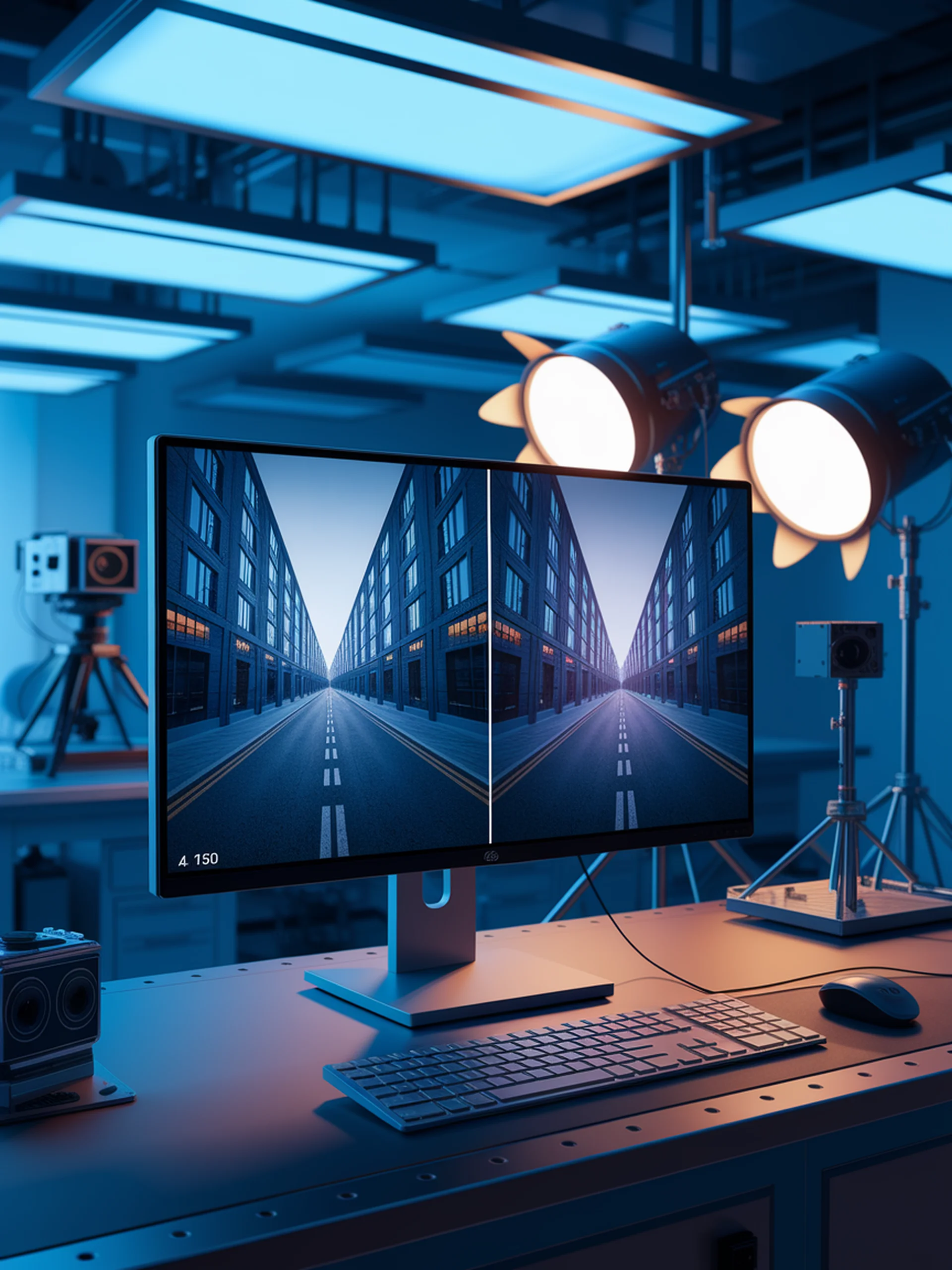
Illumination Vulnerabilities in AI Vision
How lighting changes can deceive vision-language models
Researchers developed the Illumination Transformation Attack (ITA), the first framework to systematically assess how Vision-Language Models (VLMs) respond to lighting changes.
- ITA reveals significant vulnerabilities in VLMs when processing images under different lighting conditions
- These vulnerabilities can be exploited to manipulate model outputs, creating security risks
- The research demonstrates how seemingly natural environmental changes can dramatically reduce model performance
- Exposes a critical gap in the robustness testing of visual AI systems
For security professionals, this research highlights the urgent need to develop lighting-robust vision systems before deploying them in safety-critical applications like autonomous vehicles or security surveillance.