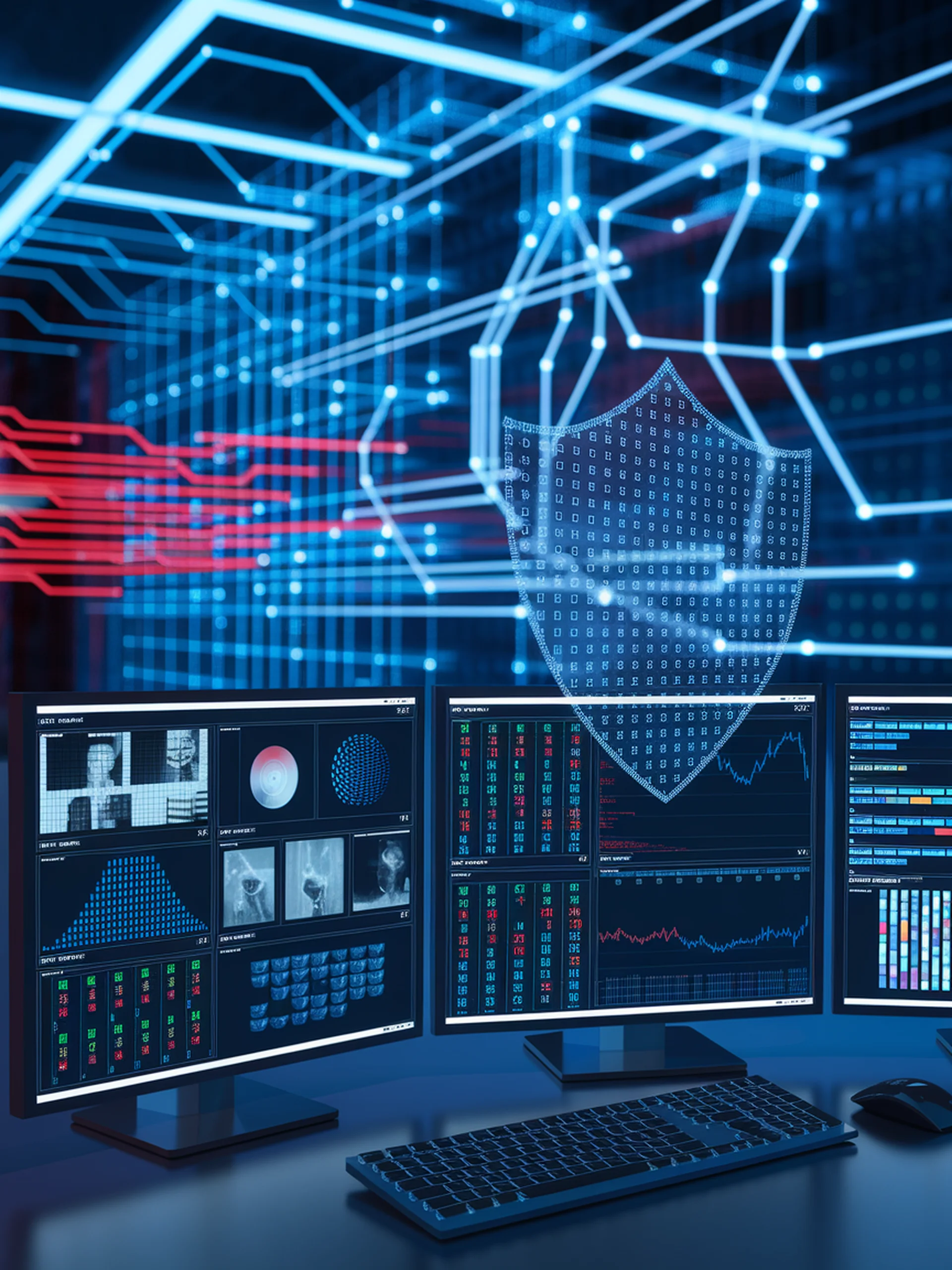
Defending Multimodal LLMs Against Adversarial Attacks
Understanding vulnerabilities across text, image, audio, and video modalities
This survey examines the security vulnerabilities of Multimodal Large Language Models (MLLMs) and systematically analyzes adversarial attack techniques that can compromise their reliability.
- Cross-modal vulnerabilities create unique attack surfaces not present in unimodal systems
- Adversarial manipulations can affect model outputs through subtle modifications to images, text, audio, or video inputs
- Existing defense mechanisms are often insufficient against sophisticated multimodal attacks
- Standardized evaluation frameworks are needed to properly assess MLLM robustness
As MLLMs increasingly power critical applications across industries, understanding these security risks is essential for developing robust AI systems that can be safely deployed in real-world environments.
Survey of Adversarial Robustness in Multimodal Large Language Models