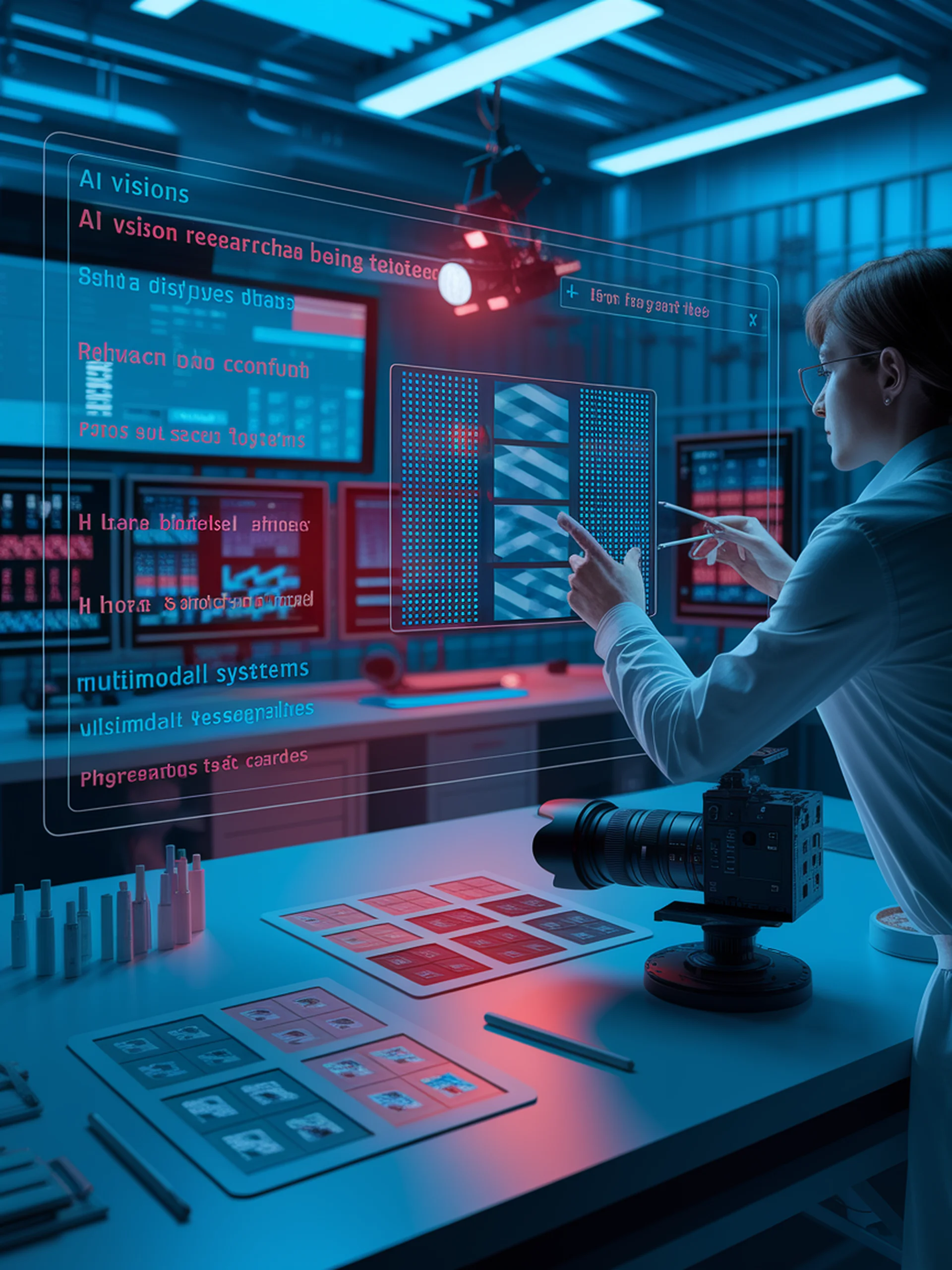
The SCAM Dataset: Exposing Visual-Text Vulnerabilities
Largest real-world dataset for evaluating multimodal model security
This research introduces the SCAM dataset to test how vulnerable multimodal AI models are to misleading text embedded in images.
- Largest diverse collection of 1,162 real-world typographic attack images
- Spans hundreds of object categories with various attack words
- Reveals significant security vulnerabilities in current foundation models
- Provides a benchmark for improving AI systems against visual-textual manipulation
This work is crucial for security as it exposes how easily multimodal models can be deceived by malicious text in images, helping developers build more robust systems that can resist real-world manipulation attempts.
SCAM: A Real-World Typographic Robustness Evaluation for Multimodal Foundation Models