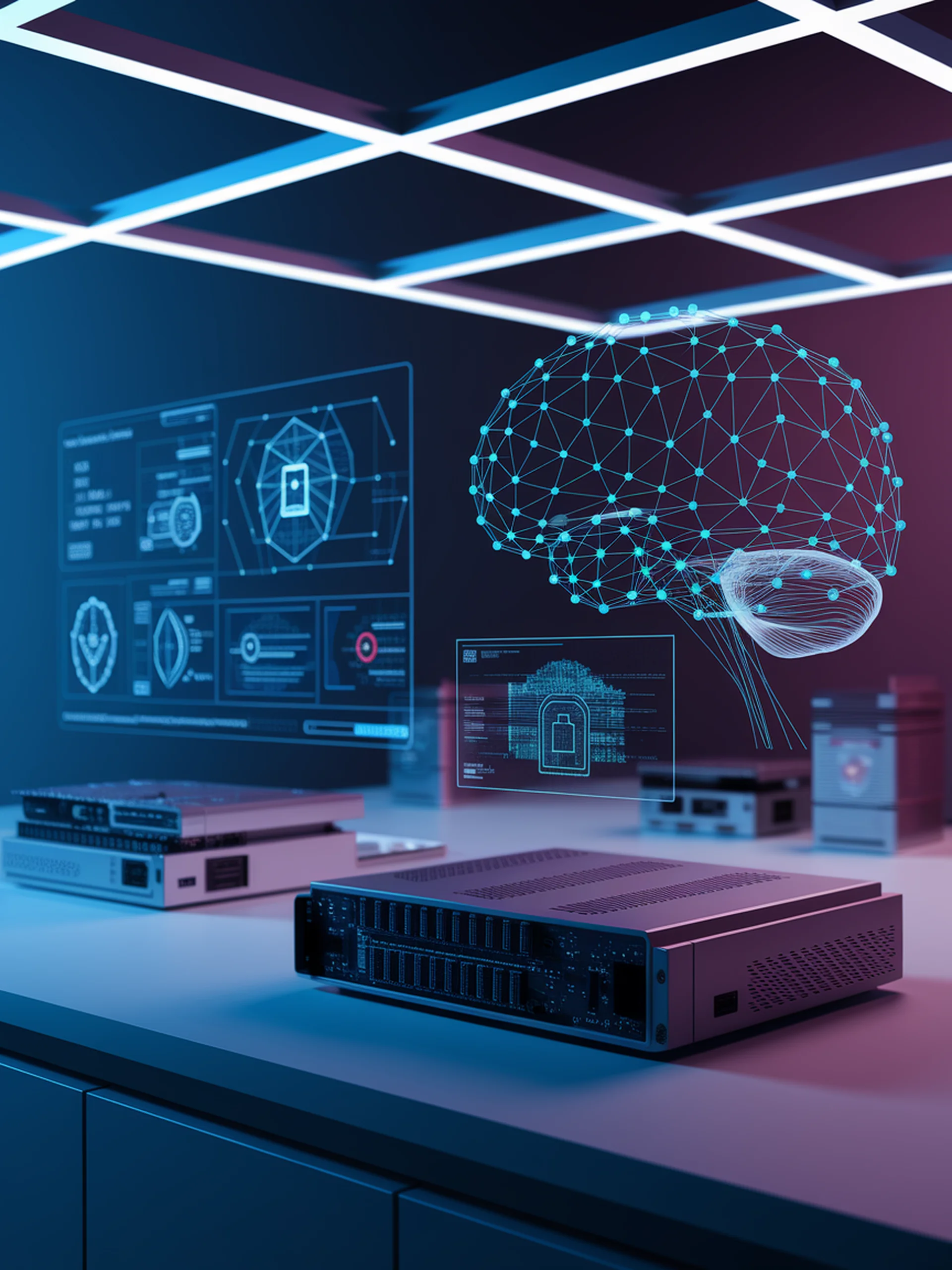
Rethinking Safety Alignment in Multi-modal AI
Building safer models without malicious training data
This research challenges the conventional approach to safety alignment in multi-modal large language models (MLLMs) by demonstrating effective protection against harmful outputs without using curated malicious data.
- Key innovation: A novel safety alignment approach using only benign data and augmentation techniques
- Improved security: Effectively defends against vision-domain attacks like typographic manipulation
- Practical advantage: Reduces the need for potentially harmful datasets in the alignment process
- Broader impact: Addresses the critical alignment gap in MLLMs when processing multi-modal inputs
For security professionals, this research offers a path to develop safer AI systems with reduced risk of exposure to harmful content during the training process, while maintaining strong defense capabilities against potential attacks.
Do We Really Need Curated Malicious Data for Safety Alignment in Multi-modal Large Language Models?