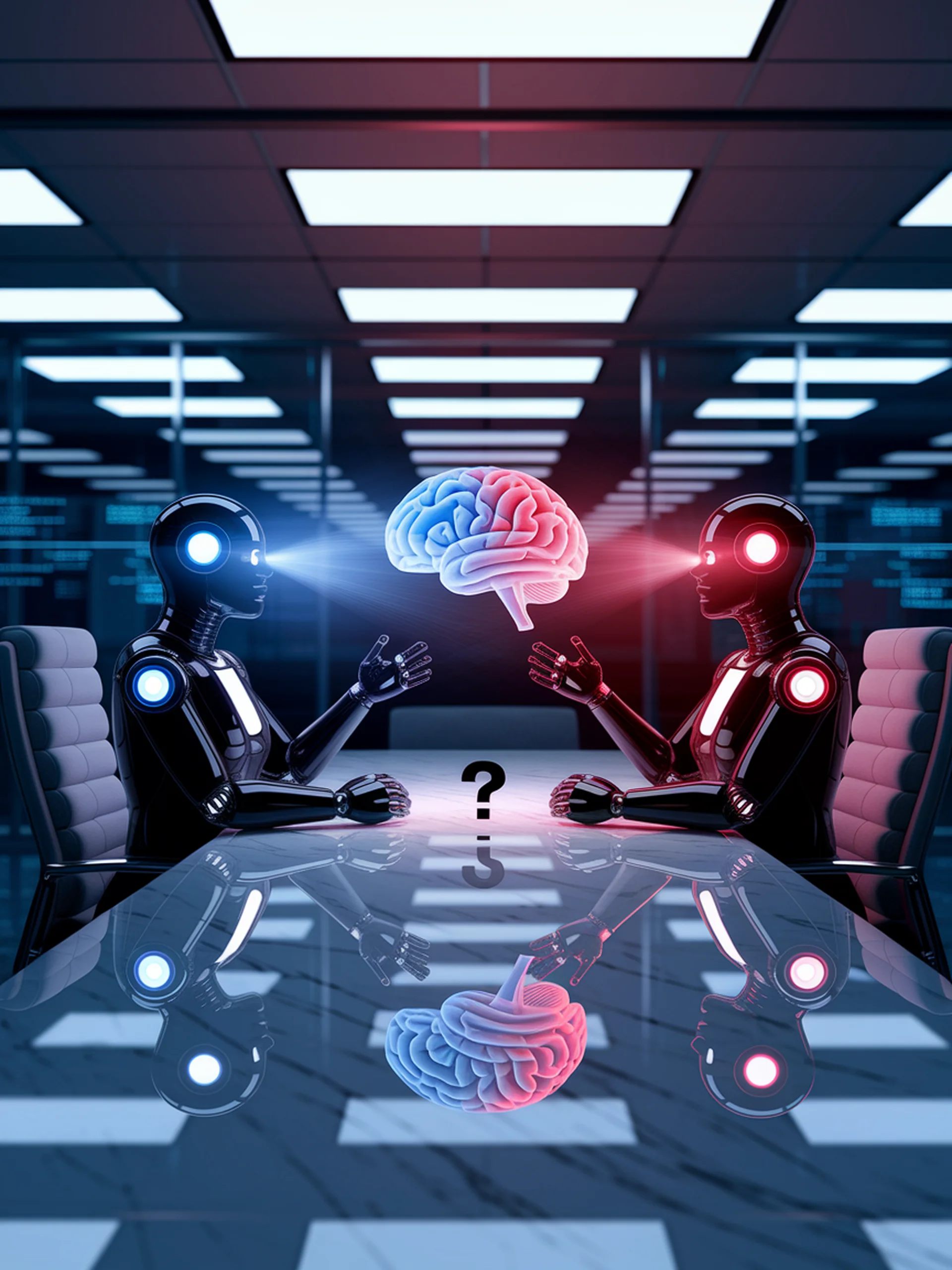
Truth vs. Persuasion in AI Debates
How LLMs can be persuaded to endorse falsehoods despite knowing better
This research introduces a new metric to measure how easily AI systems can be persuaded to accept falsehoods in multi-agent debates, even when they know the correct answer.
Key Findings:
- LLMs serving as judges can be persuaded to endorse false information despite having factual knowledge
- The researchers developed Confidence-Weighted Persuasion Override Rate (CW-POR) to quantify this vulnerability
- Different LLM architectures show varying levels of susceptibility to persuasion
- Even advanced models can be swayed by confident, persuasive falsehoods
Security Implications: This vulnerability creates significant risks for AI safety and security, as bad actors could potentially manipulate AI systems to confidently spread misinformation or make harmful decisions based on false premises.