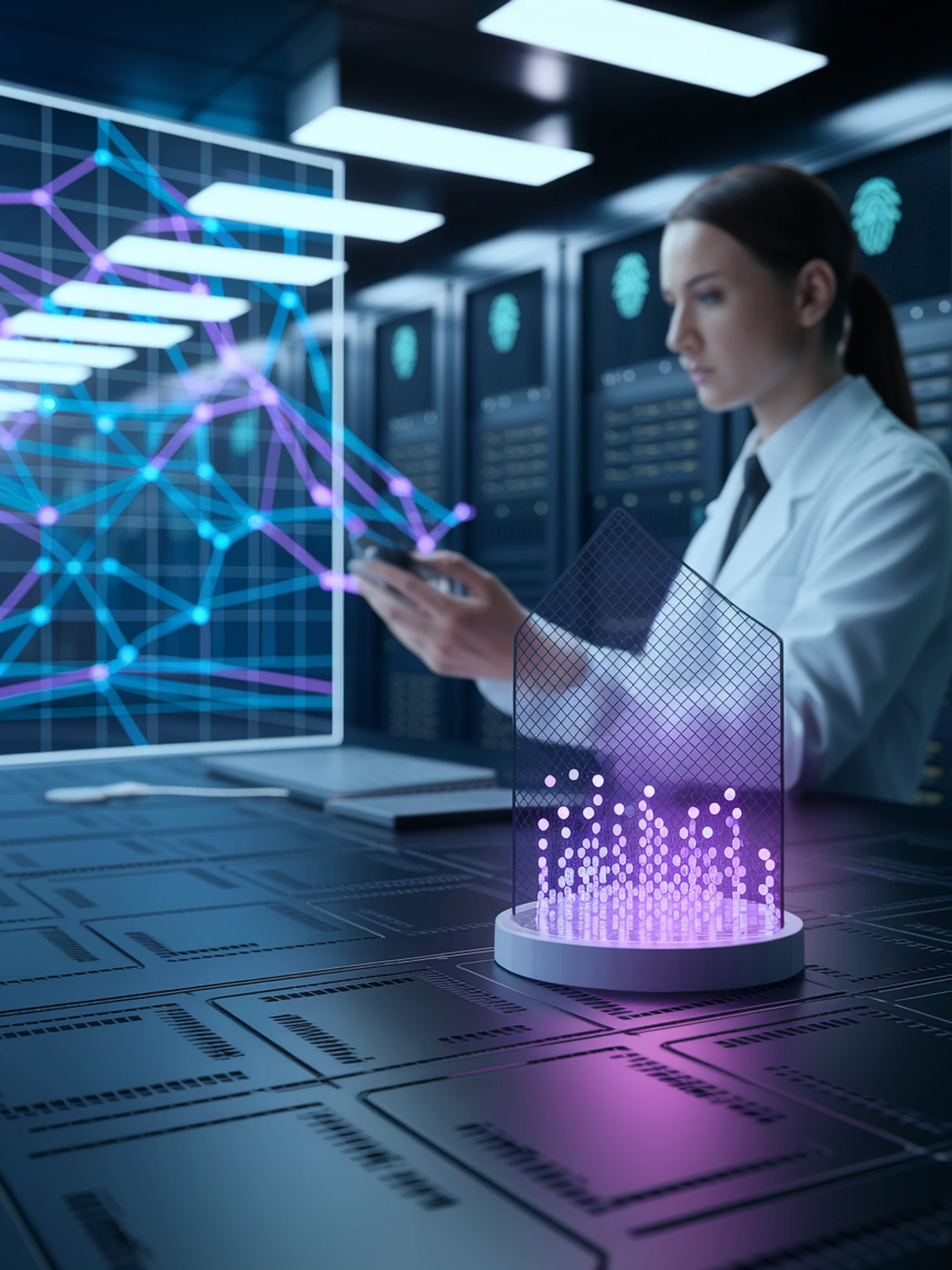
Privacy-Preserving ML for Similarity-Based Models
New DP-SGD approach for contrastive learning in LLMs
This research advances differential privacy for large language models and computer vision systems that rely on similarity-based training objectives like contrastive learning.
- Addresses a critical gap in privacy protection for models using non-decomposable objective functions
- Develops a novel DP-SGD variant specifically designed for similarity and contrastive losses
- Enables privacy-preserving training while maintaining model performance
- Particularly important for unsupervised pre-training in LLMs where standard DP approaches fall short
As privacy regulations tighten globally, this work provides a practical solution for organizations developing LLMs and vision models that must protect user data while leveraging powerful contrastive learning techniques.
Differentially Private Optimization for Non-Decomposable Objective Functions