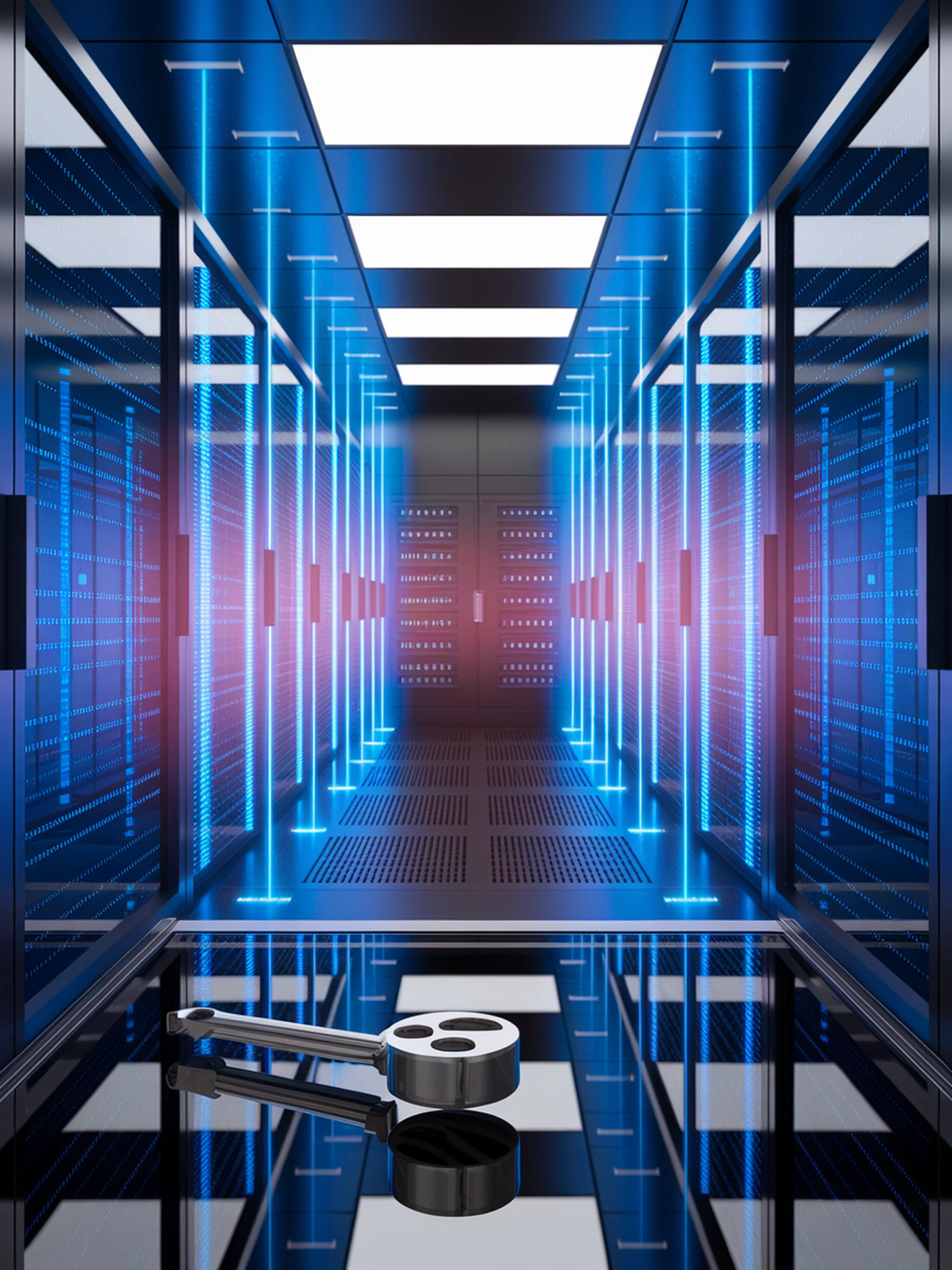
Securing LLMs: Privacy Without Compromise
A practical framework for private interactions with black-box language models
InferDPT introduces the first practical privacy-preserving framework for black-box Large Language Models, balancing security and usability.
- Implements differential privacy techniques specifically for text-based LLM interactions
- Prevents unauthorized data leakage while maintaining model utility
- Offers a practical solution with manageable computation and communication costs
- Demonstrates effective privacy protection rates against various attack vectors
This research addresses critical security concerns for organizations deploying LLMs, enabling confident adoption while protecting sensitive information and ensuring regulatory compliance.
InferDPT: Privacy-Preserving Inference for Black-box Large Language Model