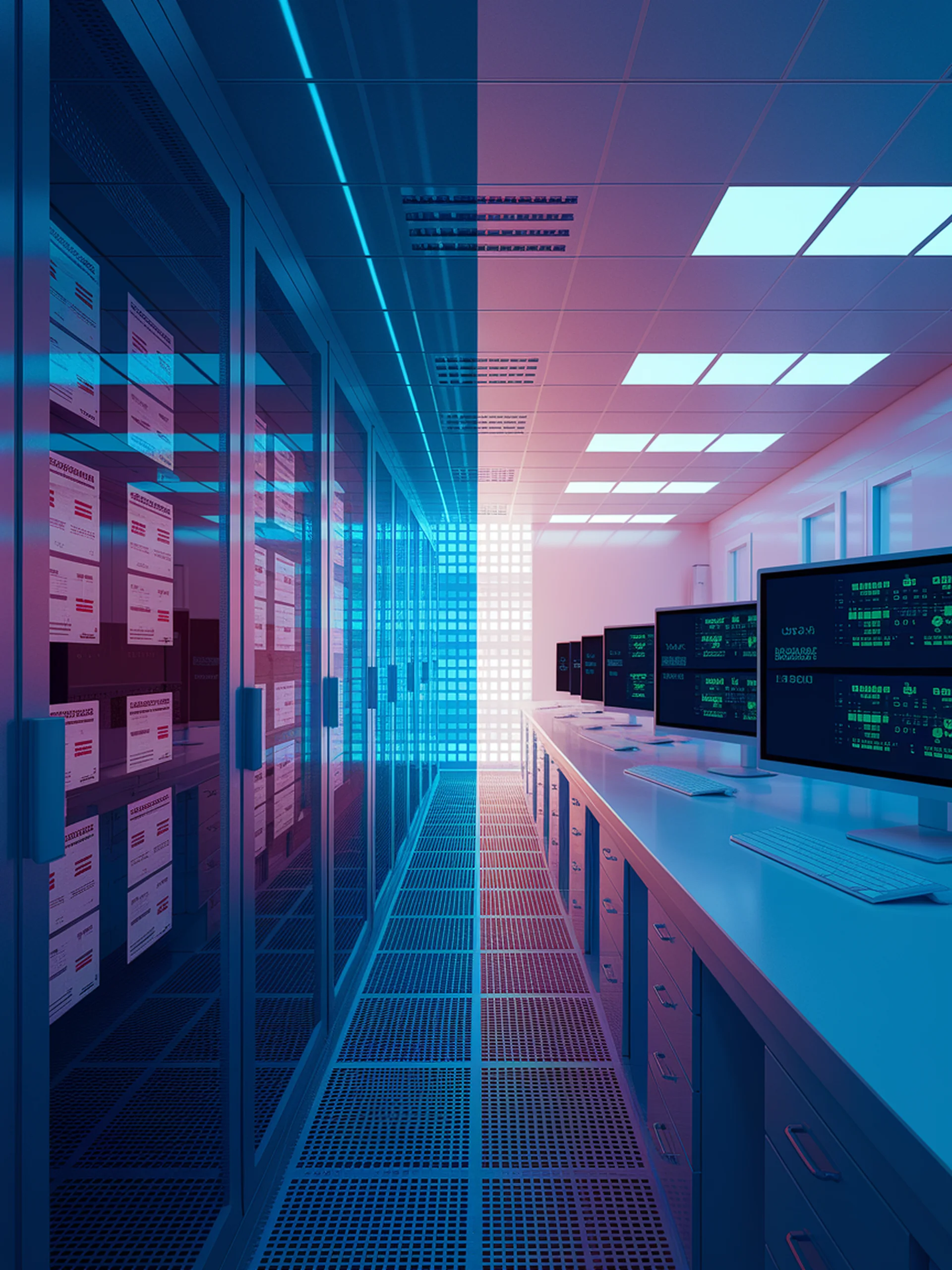
Beyond De-identification: The Promise of Synthetic Medical Data
Comparing Privacy Protection Methods for Clinical Notes
This research evaluates how synthetic clinical notes generated by AI models compare to traditional de-identification methods for protecting patient privacy while maintaining data utility.
- De-identification alone proved insufficient for privacy protection
- Synthetic data offers enhanced privacy safeguards with comparable utility
- Large language models show promise in generating realistic clinical notes
- Both approaches have trade-offs between privacy protection and data utility
Why This Matters: Healthcare organizations need better solutions for sharing sensitive clinical data while meeting privacy regulations. Synthetic data generation provides a viable alternative that balances privacy concerns with research needs without exposing actual patient information.
De-identification is not enough: a comparison between de-identified and synthetic clinical notes