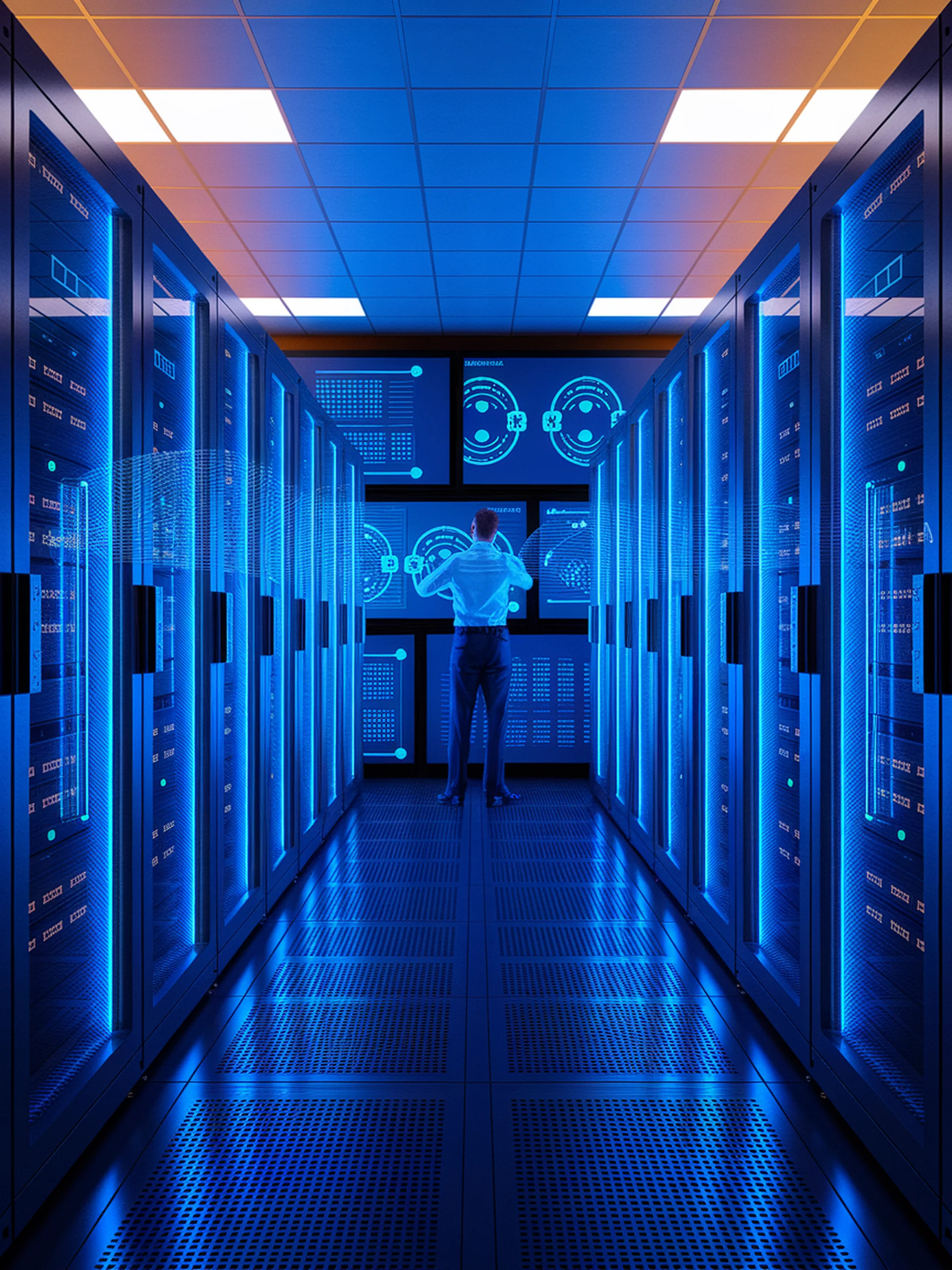
Safeguarding Privacy in the LLM Era
A comprehensive analysis of privacy threats and protection strategies
This research provides a critical examination of privacy vulnerabilities in Large Language Models and evaluates current protection mechanisms, particularly for sensitive domains like healthcare.
- Privacy Threats: Identifies key vulnerabilities in LLMs trained on internet-sourced datasets
- Protection Mechanisms: Analyzes effectiveness of anonymization, differential privacy, and machine unlearning
- Healthcare Focus: Highlights specific privacy concerns in medical applications
- Security Framework: Offers a structured approach to understanding and mitigating LLM privacy risks
The findings are crucial for security professionals developing responsible AI systems that balance innovation with robust privacy protection in an increasingly AI-driven world.
Preserving Privacy in Large Language Models: A Survey on Current Threats and Solutions