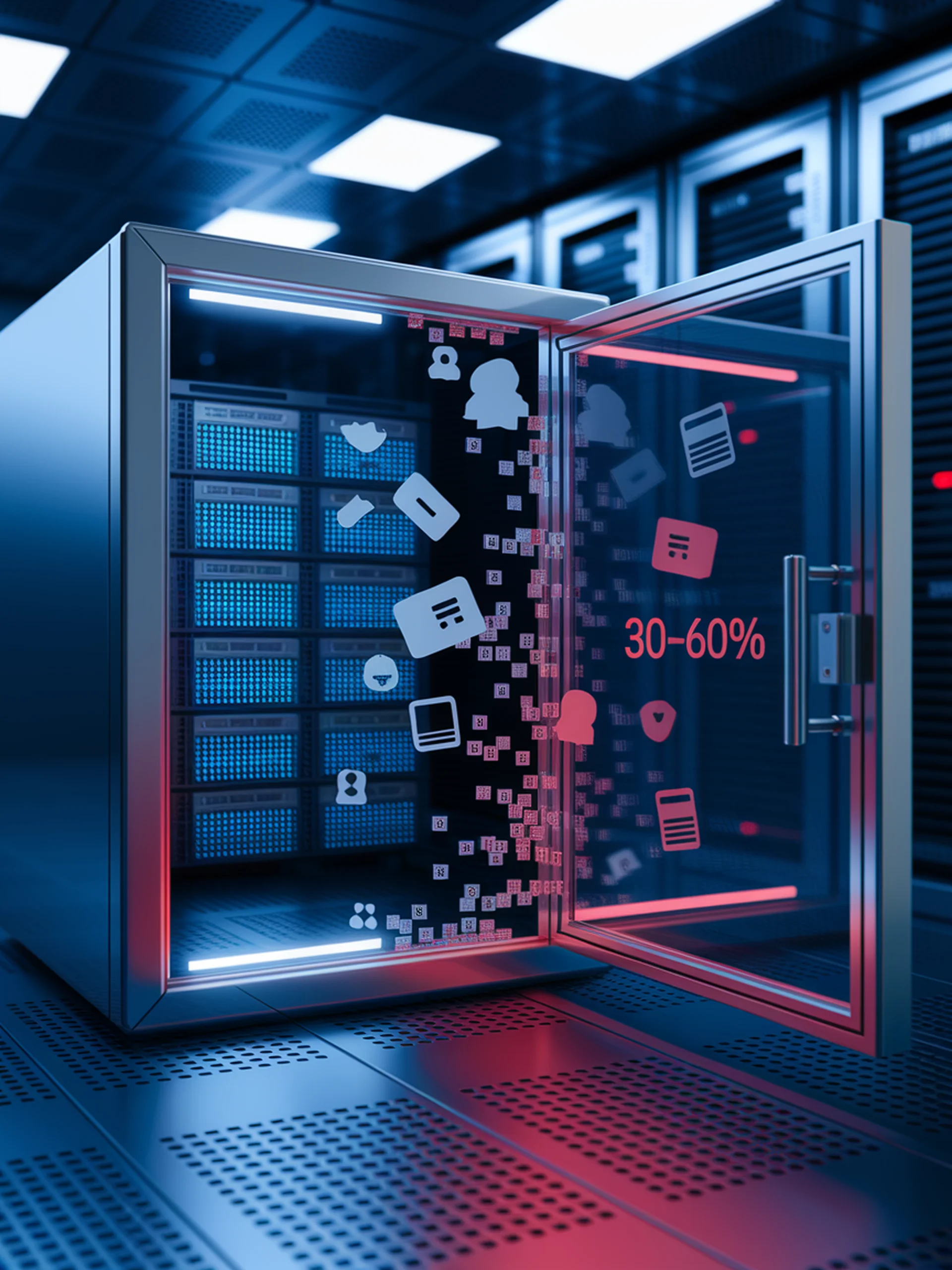
The False Privacy of Synthetic Data
Why generated data doesn't solve LLM privacy concerns
This research reveals that using synthetic data for fine-tuning LLMs fails to address privacy risks, as generated data can retain and leak personal information.
- 30-60% of synthetic data samples contained Personal Identifiable Information (PII) from the original dataset
- Fine-tuned models showed vulnerability to Membership Inference Attacks regardless of using synthetic data
- The more capable the generating LLM, the higher risk of privacy leakage in synthetic data
These findings challenge the security assumption that synthetic data provides adequate privacy protection when training language models, requiring organizations to implement additional safeguards even when using generated datasets.