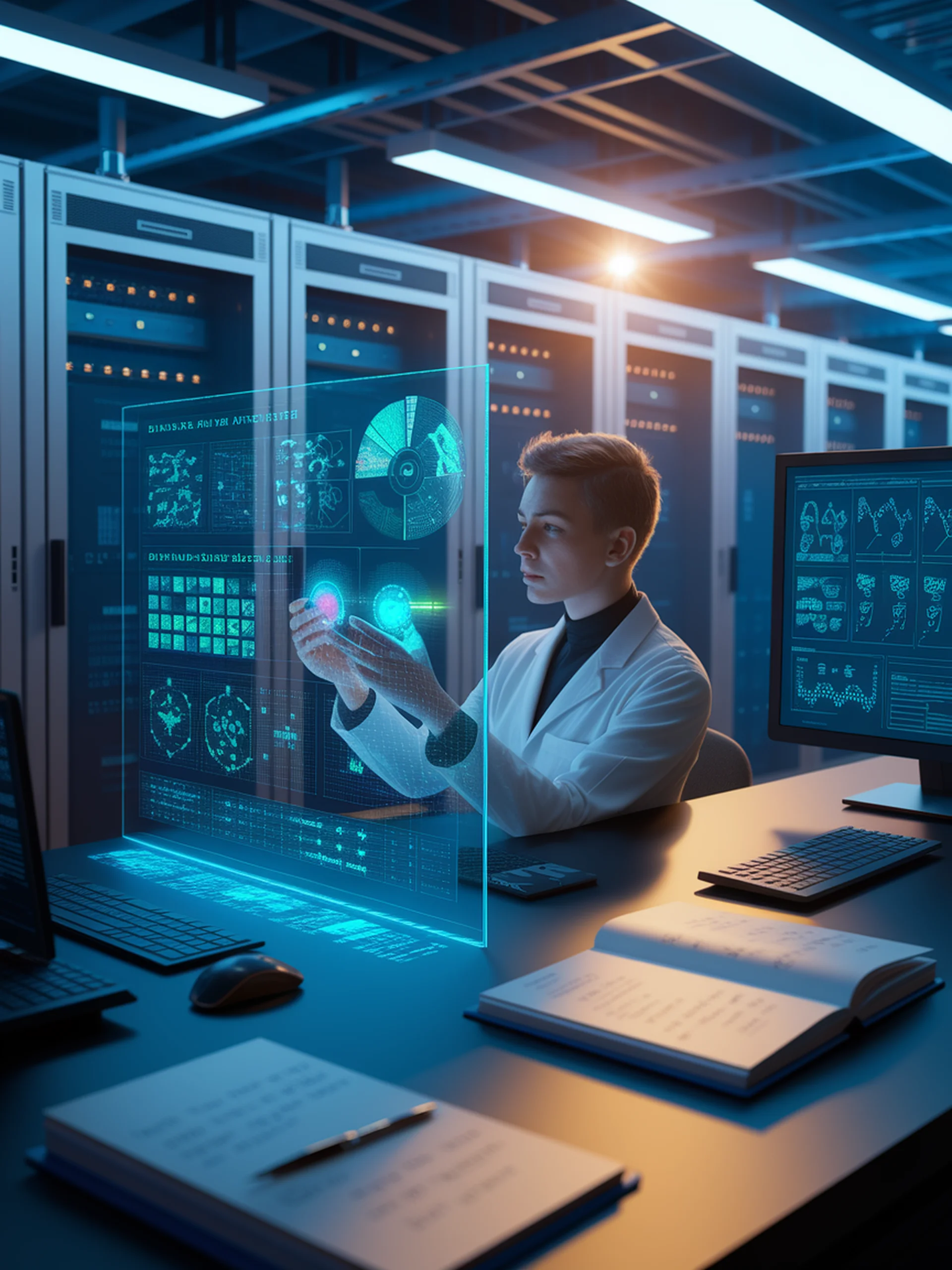
Detecting LLM Training Data
A New Method for Transparency in AI Models
This research introduces a calibrated detection method for determining whether specific text was used to train large language models, addressing critical transparency needs in AI.
- Proposes a novel divergence-based calibration approach that improves upon existing Min-K% detection methods
- Addresses the challenge of undisclosed training data in commercial LLMs
- Enhances security assessment possibilities through better identification of training materials
- Enables more thorough ethical evaluation of large language models
Why it matters: As AI models grow more powerful, understanding their training data becomes essential for security audits, copyright compliance, and ensuring ethical AI deployment.
Pretraining Data Detection for Large Language Models: A Divergence-based Calibration Method