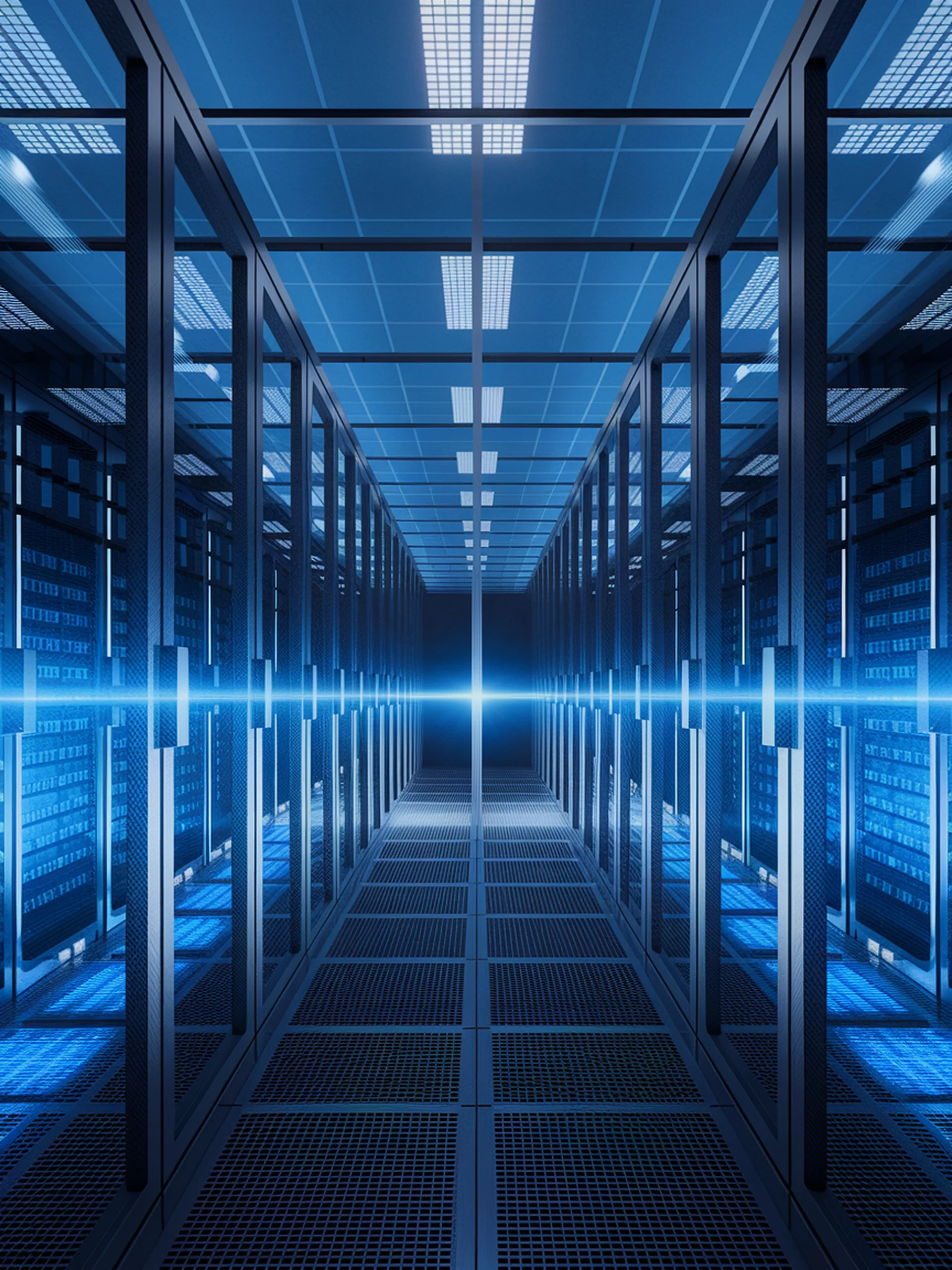
Protecting User Data in Cloud LLMs
Innovative approach to secure prompts without compromising performance
This research introduces a novel framework for securing sensitive user prompts when using cloud-based large language models, while maintaining model confidentiality and output quality.
- Secure Partitioned Decoding (SPD) confines user prompts to trusted execution environments
- Uses confidential virtual machines (CVMs) to isolate sensitive data from cloud providers
- Incorporates cryptographic prompt obfuscation for additional security layers
- Provides security without significant performance degradation
This matters for security professionals because it addresses the critical trade-off between leveraging powerful cloud LLMs and protecting sensitive or proprietary user data, enabling more secure AI implementations in regulated industries.
Confidential Prompting: Protecting User Prompts from Cloud LLM Providers