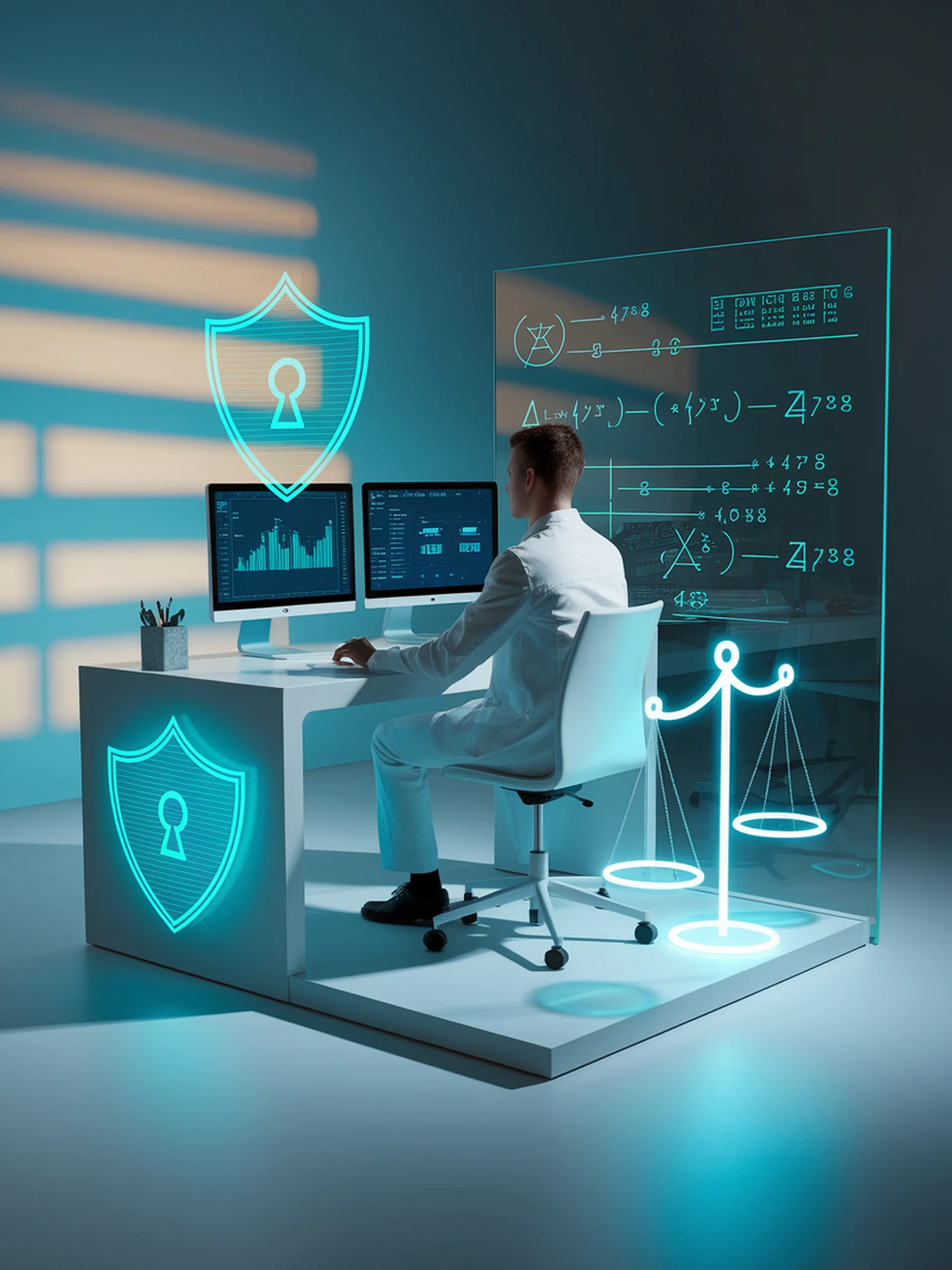
Balancing Privacy & Data Selection in Machine Learning
A novel approach to privacy-preserving active learning
This research presents a breakthrough framework that combines active learning and differential privacy to effectively select training data while preserving privacy.
- Introduces DP-BADGE, a new algorithm that maintains performance while providing differential privacy guarantees
- Demonstrates that traditional active learning methods significantly degrade when privacy constraints are applied
- Achieves up to 57.1% reduction in privacy cost compared to baseline methods
- Provides comprehensive analysis across various privacy budgets and datasets
This work addresses crucial security challenges in privacy-sensitive domains like healthcare by enabling efficient data utilization without compromising individual privacy—essential for organizations handling sensitive data in regulatory environments.
Differentially Private Active Learning: Balancing Effective Data Selection and Privacy