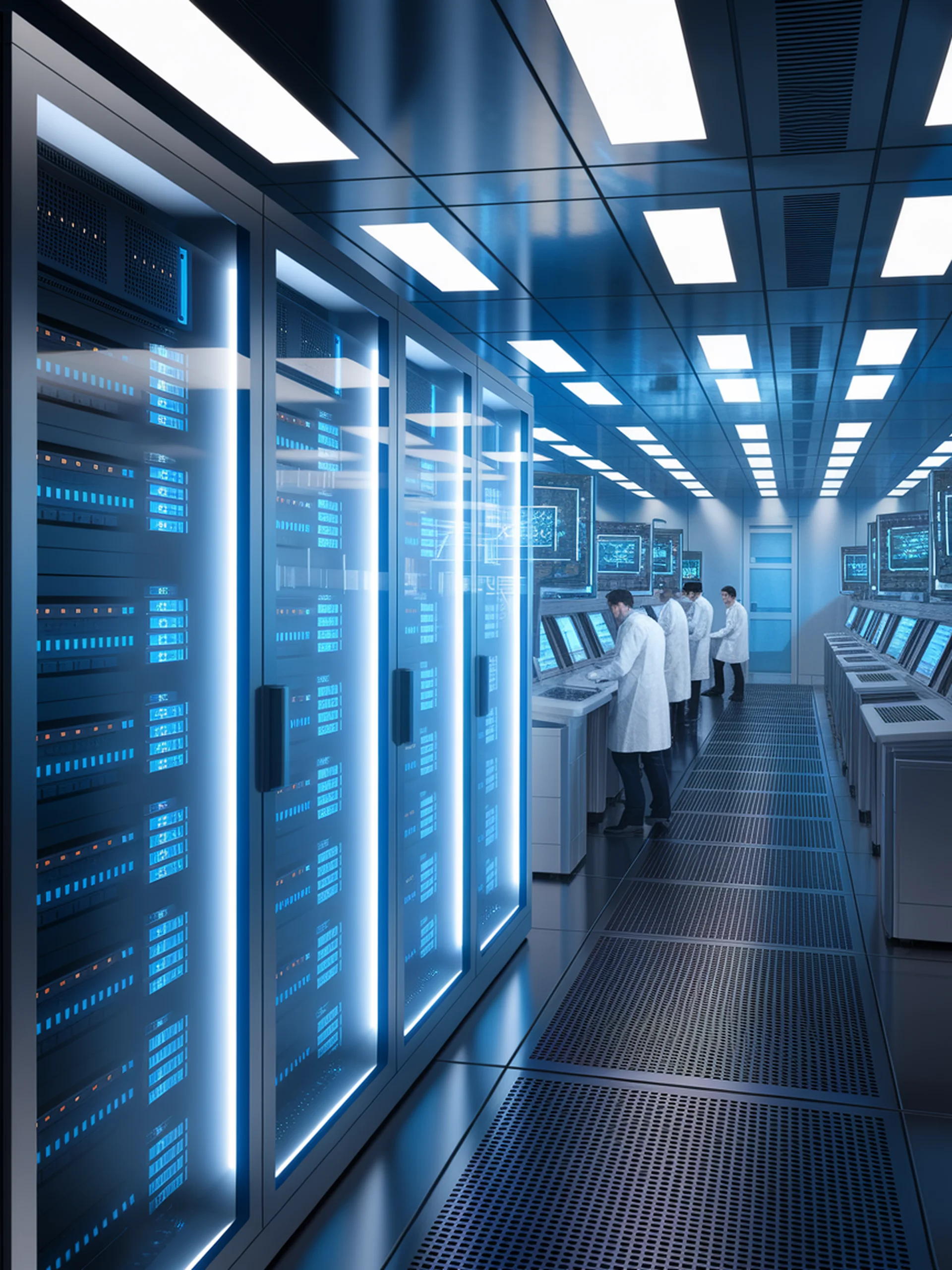
Securing LLMs with Encrypted Computation
A novel architecture for privacy-preserving language models
This research introduces an encryption-friendly LLM architecture that enables language models to process sensitive data while maintaining privacy through homomorphic encryption.
- Redesigned transformer architecture optimized for encrypted computations
- Achieves privacy-preserving inference without significant performance loss
- Balances security and computational efficiency for practical deployment
- Addresses critical privacy concerns in personalized AI interactions
This innovation represents a breakthrough for secure AI in sensitive domains like healthcare, finance, and legal services where data privacy is paramount while still leveraging powerful language models.