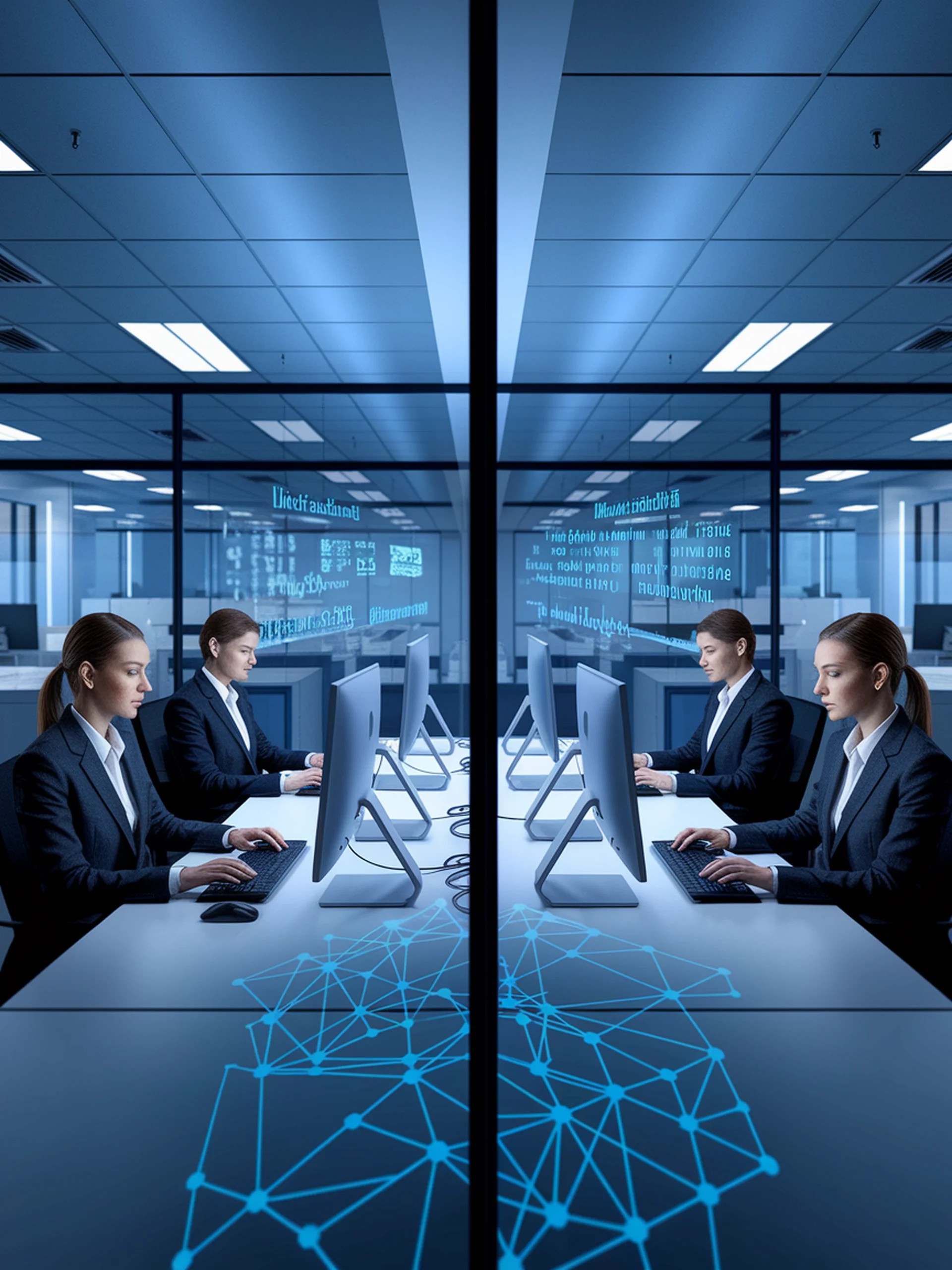
Secure LLM Fine-Tuning Without Data Sharing
Personalized federated learning for heterogeneous data environments
FedAMoLE enables collaborative fine-tuning of Large Language Models while preserving data privacy through innovative federated learning architecture.
- Addresses the challenge of training LLMs on sensitive instruction data that cannot be publicly shared
- Deploys heterogeneous model architectures tailored to each client's data characteristics
- Achieves better performance than uniform model approaches while maintaining privacy
- Enables scalable, secure collaboration across organizations with varying data volumes and formats
This research advances security in AI by allowing organizations to benefit from collective model improvement without exposing sensitive information—critical for privacy-compliant AI development in regulated industries.
Personalized Federated Fine-Tuning for LLMs via Data-Driven Heterogeneous Model Architectures