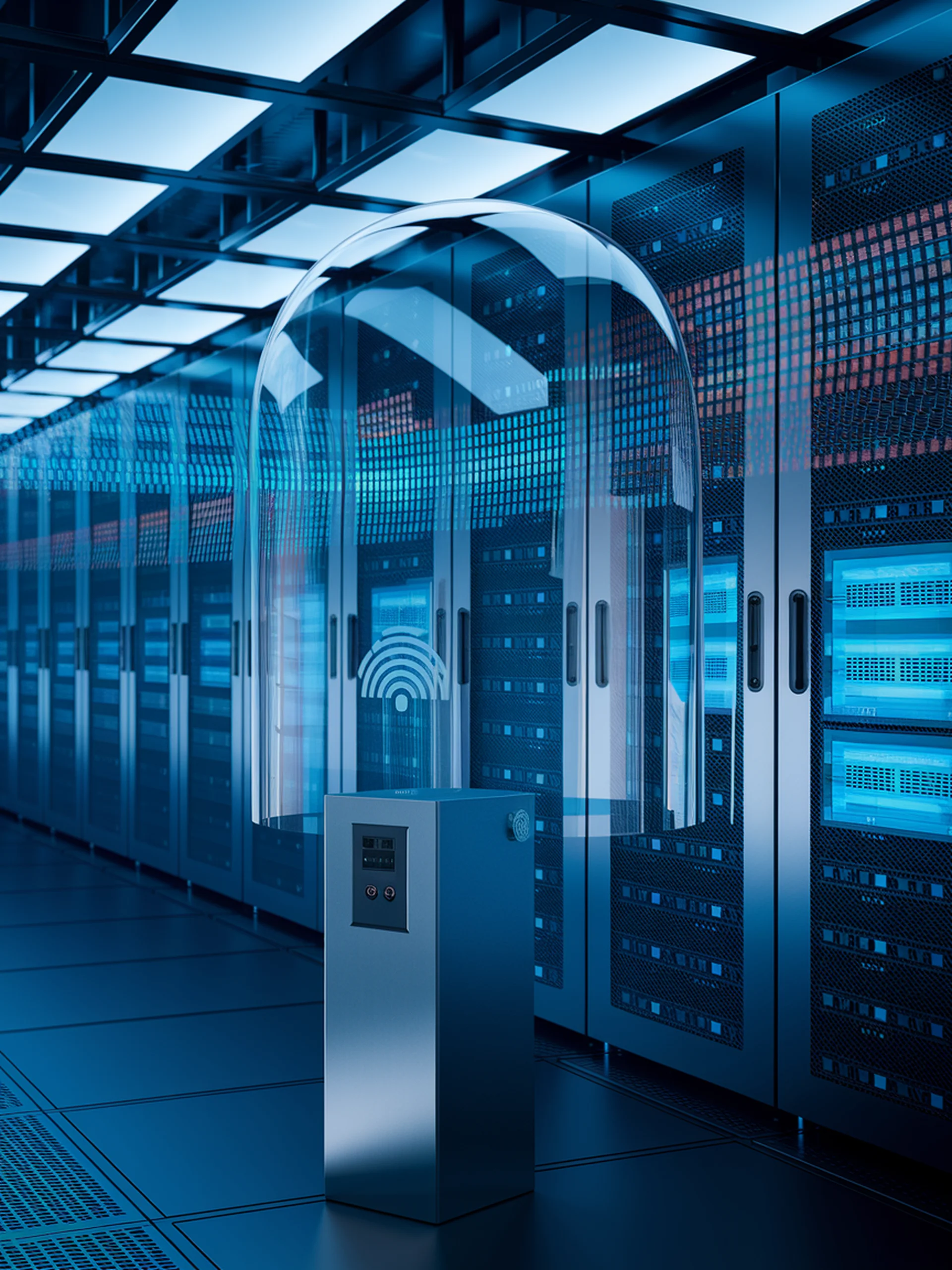
Protecting Privacy in LLM Fine-tuning
Understanding vulnerabilities and defenses for sensitive data protection
This research provides a comprehensive analysis of privacy risks during the fine-tuning stage of Large Language Models, highlighting both attack vectors and defense mechanisms.
- Vulnerability identification: Maps out key privacy threats including membership inference, data extraction, and backdoor attacks
- Defense evaluation: Assesses protective measures like differential privacy and secure computing techniques
- Security framework: Establishes a systematic approach for evaluating and enhancing privacy in LLM fine-tuning processes
- Future directions: Outlines emerging challenges and research opportunities in LLM privacy protection
Critical for organizations deploying custom LLMs in security-sensitive environments where protecting confidential information is paramount.
Privacy in Fine-tuning Large Language Models: Attacks, Defenses, and Future Directions