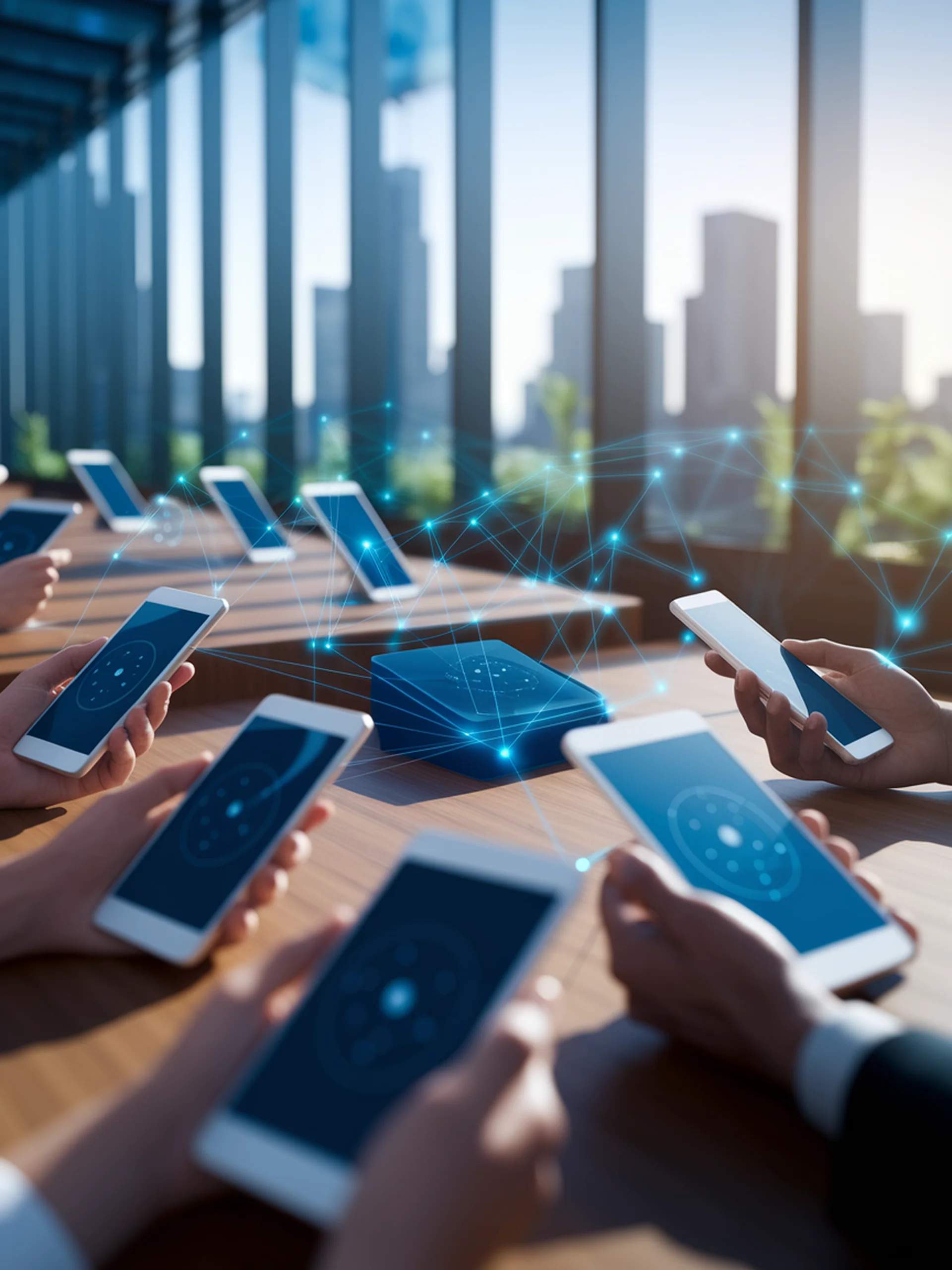
Decentralized Learning at the Edge
Privacy-Preserving Collaborative ML for Mobile Devices
ML Mule introduces a mobile-driven approach to collaborative learning that protects privacy while improving model performance through contextual awareness.
- Decentralized Architecture - Shifts ML processing from centralized data centers to edge devices, reducing privacy risks and infrastructure costs
- Context-Aware Collaboration - Intelligently shares model updates between devices based on user context and relevance
- Privacy Preservation - Keeps sensitive user data on local devices while still benefiting from collaborative learning
- Efficient Resource Usage - Optimizes when and how model updates are shared based on device capabilities
Security Impact: By keeping personal data on users' devices and implementing context-based sharing, ML Mule addresses critical privacy concerns in AI deployment while maintaining high model performance.