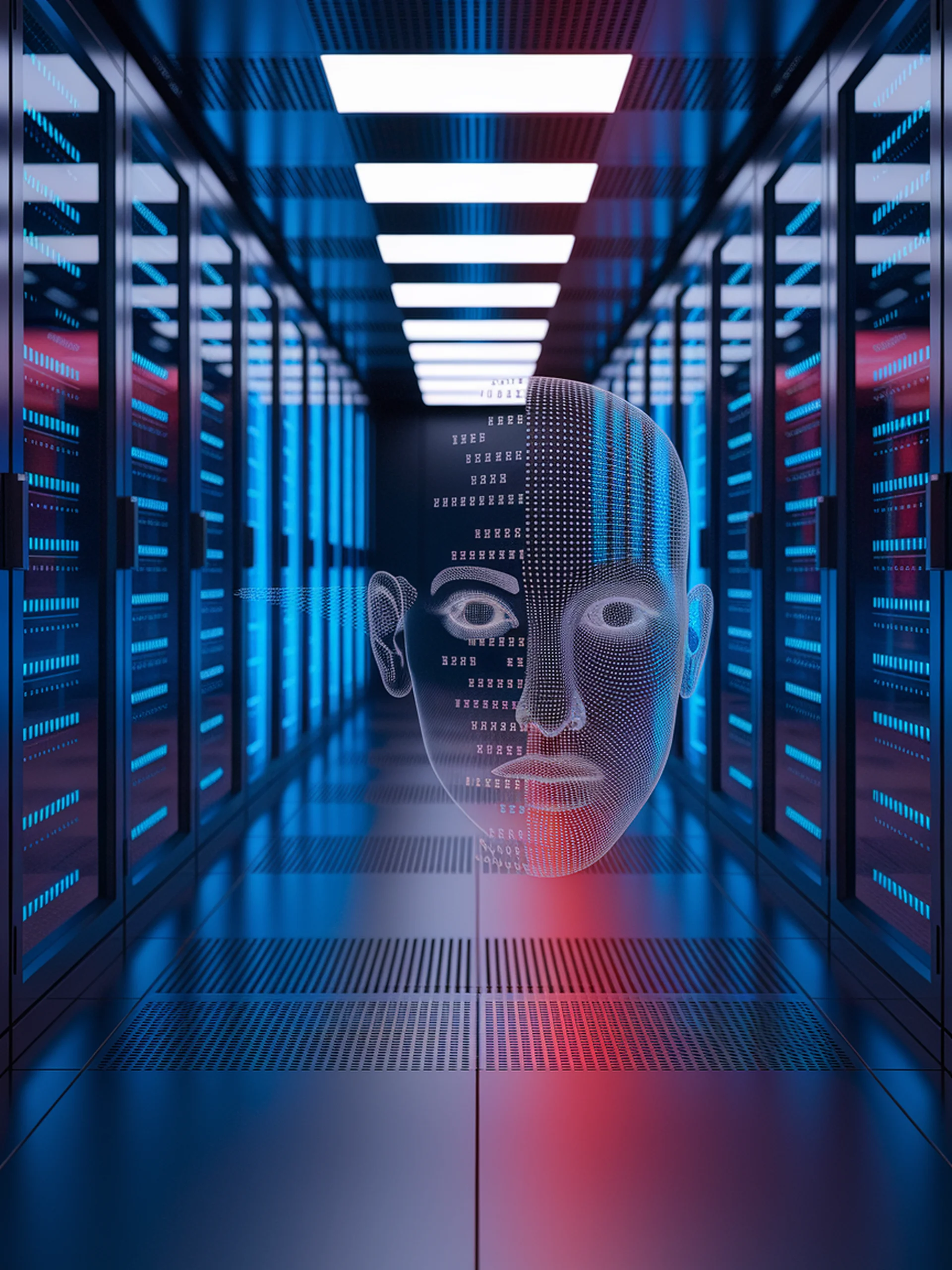
Privacy Vulnerabilities in VLMs
Detecting Data Leakage in Vision-Language Models
This research reveals how membership inference attacks can expose private information in Vision-Language Models (VLMs), posing significant security risks for multi-modal AI systems.
- Successfully demonstrates how attackers can determine if specific data was used in training
- Evaluates vulnerability across different VLM architectures and configurations
- Identifies factors that increase security risks in multi-modal models
- Highlights the urgent need for privacy-preserving techniques in VLM development
As VLMs become increasingly integrated into critical applications, these findings underscore the importance of implementing robust security measures to protect sensitive data from exploitation while maintaining model performance.