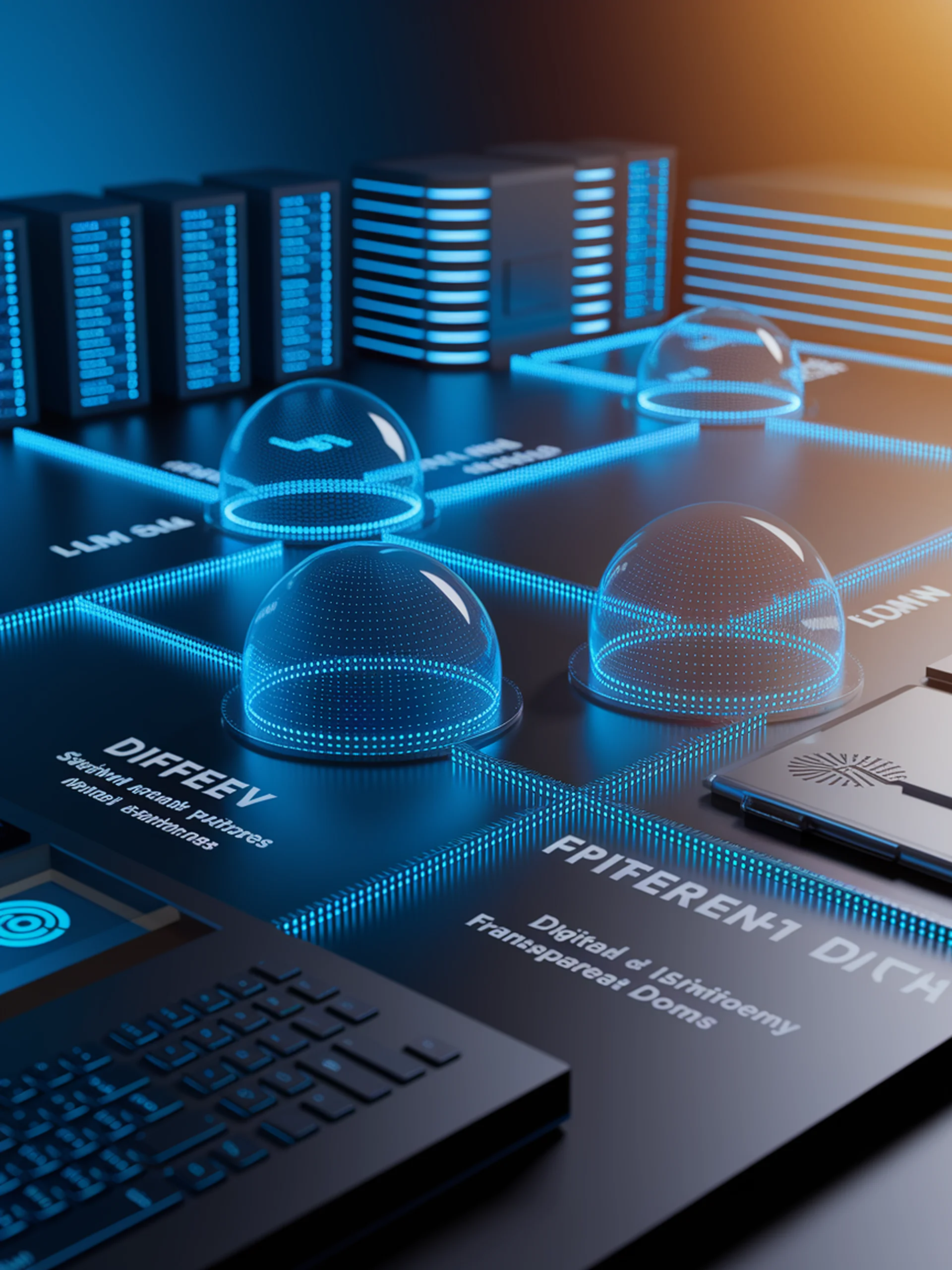
Securing Federated Learning for LLMs
Privacy-Preserving Framework Balances Security and Performance
This research introduces an interactive framework that implements Differential Privacy in federated learning for LLMs while addressing the tradeoff between privacy and model utility.
- Enables organizations to train robust AI models while keeping user data local
- Tackles emerging privacy threats in federated learning environments
- Provides practical implementation beyond theoretical worst-case privacy guarantees
- Demonstrates effectiveness specifically with Large Language Models
The work addresses critical security concerns for enterprises developing AI solutions with sensitive data, offering a pathway to maintain privacy compliance without severely compromising performance.