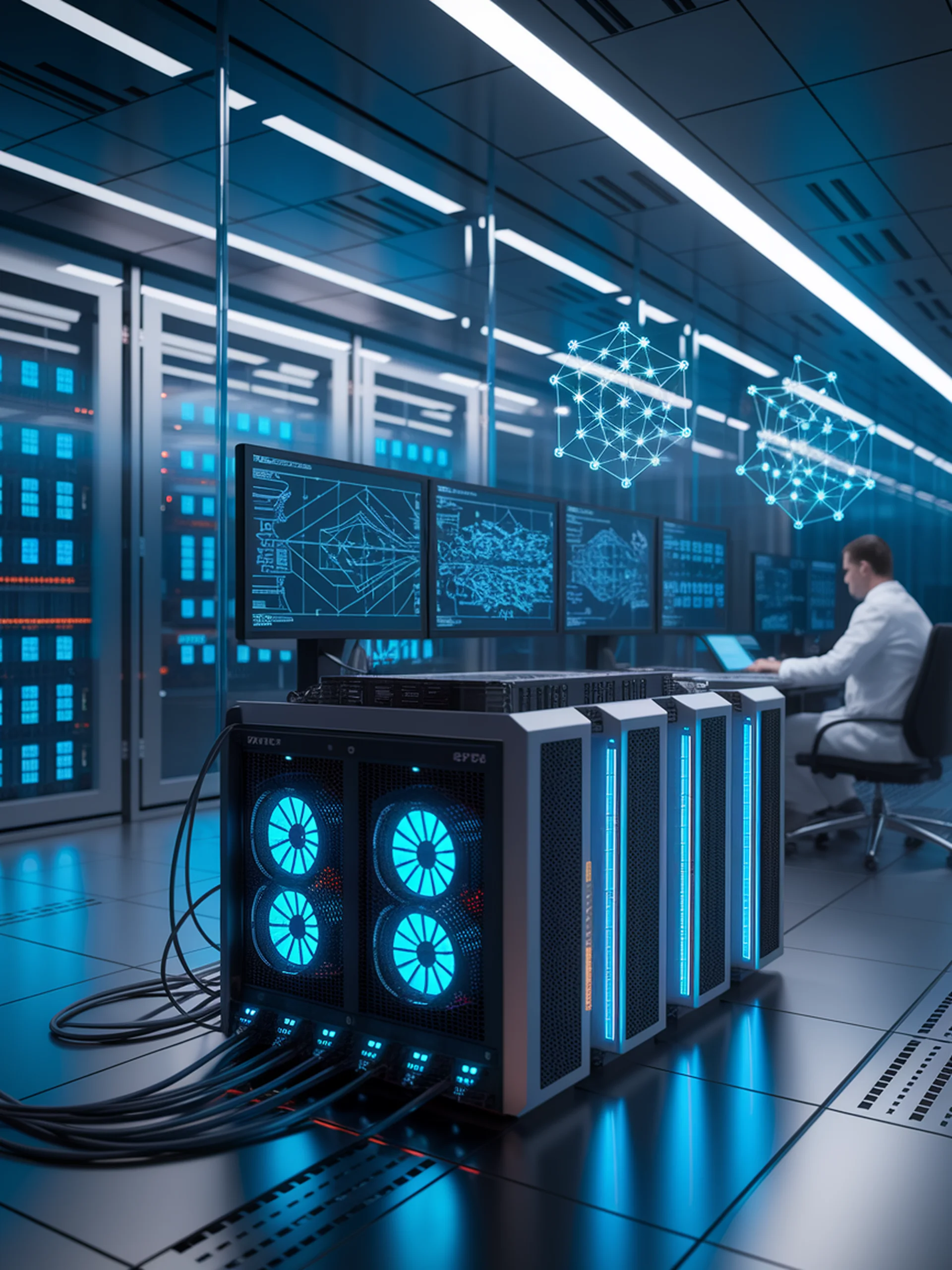
Accelerating Homomorphic Encryption with AI
GPU-powered algorithms and LLM coding for faster privacy-preserving computation
This research introduces a novel approach to dramatically speed up Number Theoretic Transform (NTT) implementation—a critical bottleneck in privacy-preserving machine learning systems.
- GPU-accelerated algorithms that enhance homomorphic encryption performance
- LLM-powered code generation to automate complex cryptographic implementations
- System-agnostic approach that works across different GPUs and operating systems
- Practical security gains for privacy-preserving machine learning applications
The innovation addresses a critical challenge in security: making homomorphic encryption efficient enough for real-world adoption in privacy-sensitive applications, accelerating the path to truly secure AI systems.
Ramp Up NTT in Record Time using GPU-Accelerated Algorithms and LLM-based Code Generation