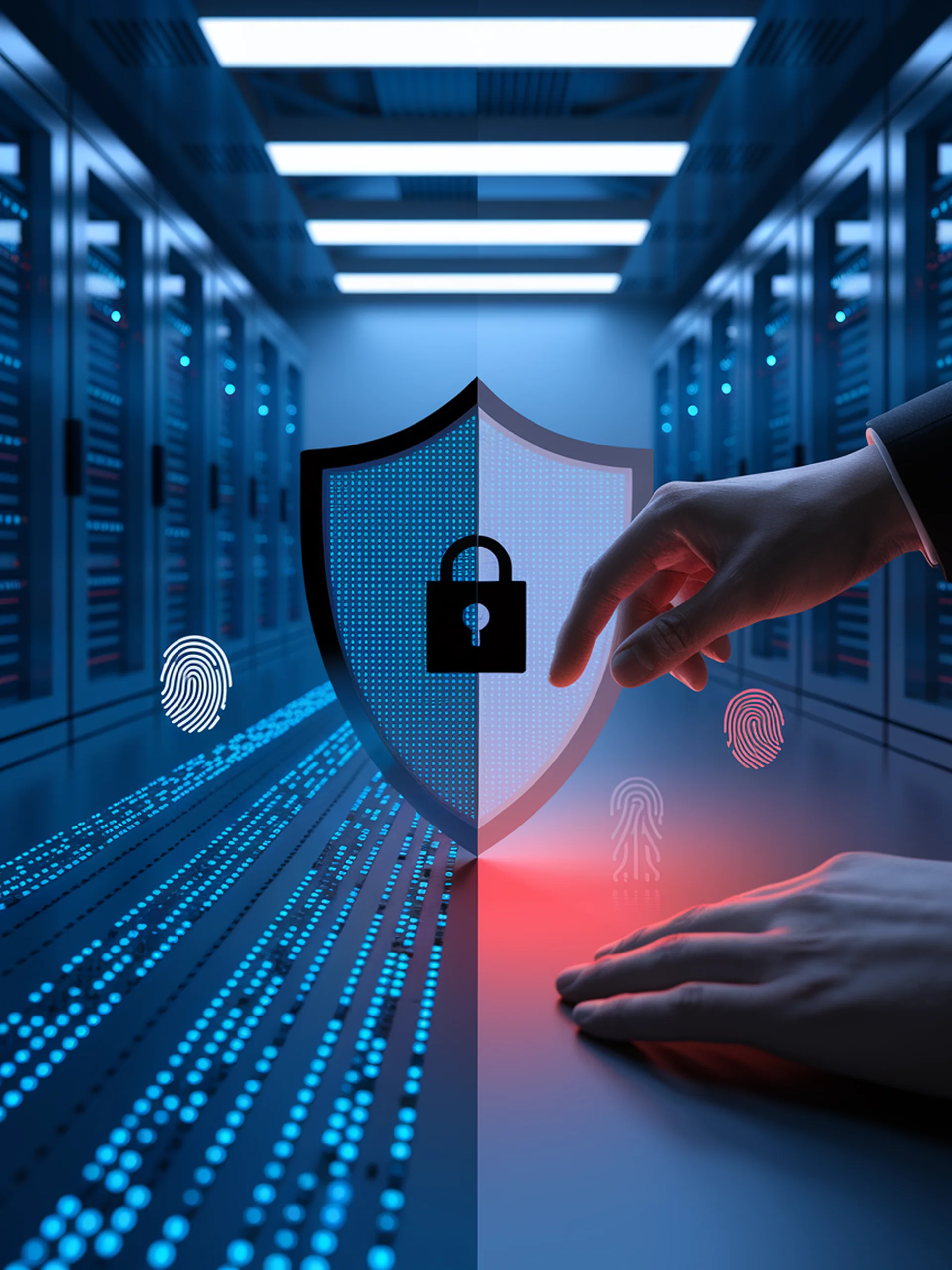
Safeguarding Privacy in LLM Interactions
A token-level approach to protect sensitive data
This research introduces a novel privacy-preserving mechanism for language model services that protects user data while maintaining functionality.
- Addresses the critical challenge of sharing sensitive data with untrusted LLM providers
- Proposes a token-level privacy approach that goes beyond semantic similarity methods
- Introduces the dchi-stencil technique to selectively protect private tokens
- Balances privacy protection with maintaining context for effective LLM responses
This work is significant for security professionals as it provides a practical method to mitigate privacy risks when organizations leverage external LLM services, enabling safer adoption of AI language technologies in sensitive domains.