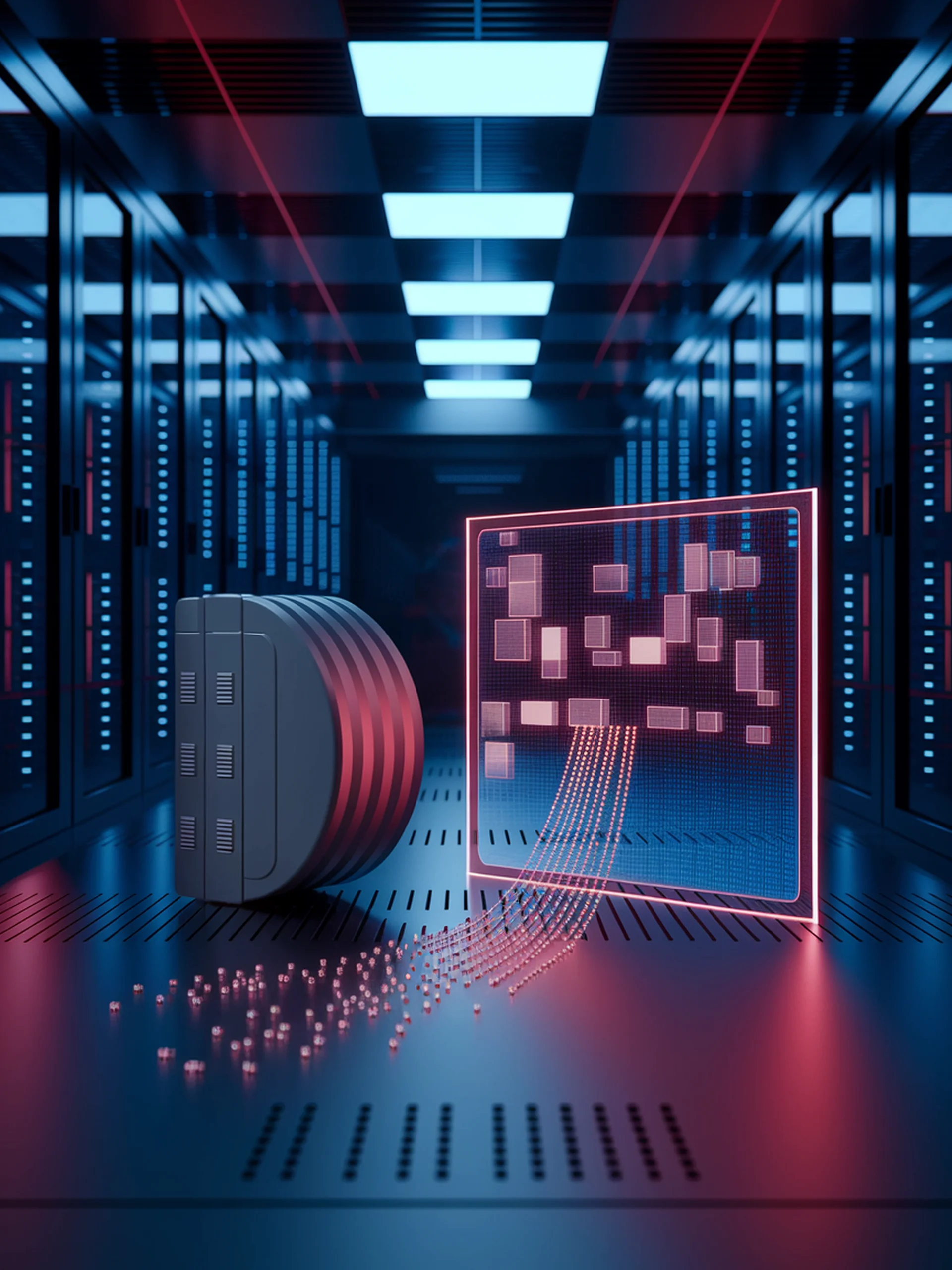
Exposing Privacy Vulnerabilities in LLMs
Advanced techniques to audit and measure privacy leakage in language models
This research introduces significantly more effective privacy auditing techniques for large language models, revealing concerning vulnerabilities in current systems.
- Develops sophisticated canary methods that outperform previous approaches for detecting training data memorization
- Demonstrates stronger membership inference attacks across multiple fine-tuned LLM families
- Provides more accurate lower bounds on privacy leakage in real-world settings
- Highlights urgent security implications for organizations deploying LLMs with sensitive data
For security professionals, this work reveals critical weaknesses in how we measure and protect against privacy breaches in AI systems, emphasizing the need for robust auditing frameworks before deployment.