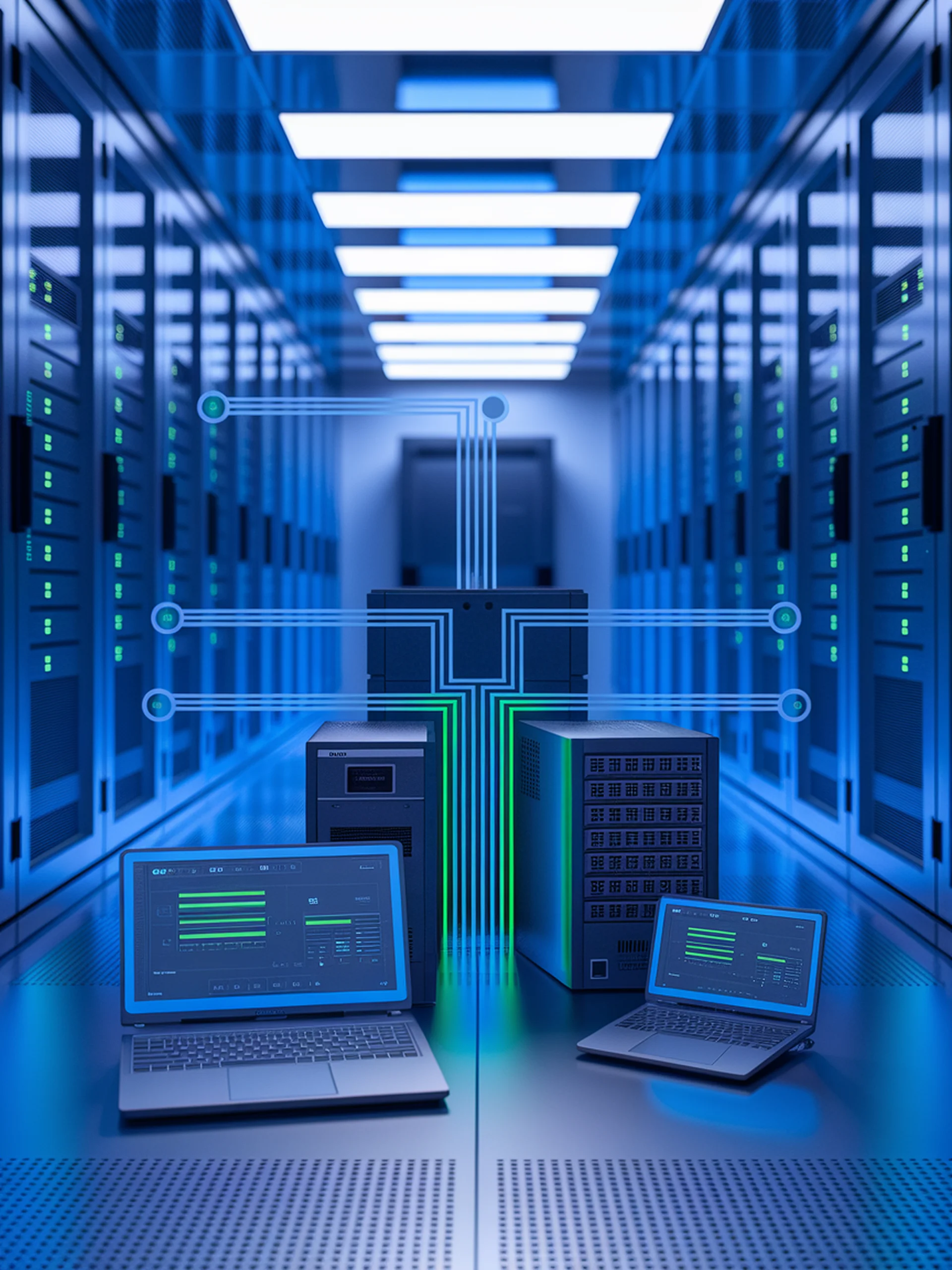
Efficient Federated LLM Fine-Tuning
Solving resource constraints and data heterogeneity across devices
HierFedLoRA introduces a hierarchical federated learning framework that enables resource-efficient fine-tuning of Large Language Models while preserving privacy.
- Combines Low-Rank Adaptation (LoRA) with federated learning to reduce computational demands
- Addresses data heterogeneity through a novel hierarchical approach
- Improves model performance while maintaining privacy of sensitive user data
- Enables deployment on resource-constrained devices through optimized parameter sharing
This research is critical for security teams deploying LLMs across distributed environments, ensuring sensitive data remains protected while still allowing models to learn from diverse user interactions without centralized data collection.
Resource-Efficient Federated Fine-Tuning Large Language Models for Heterogeneous Data