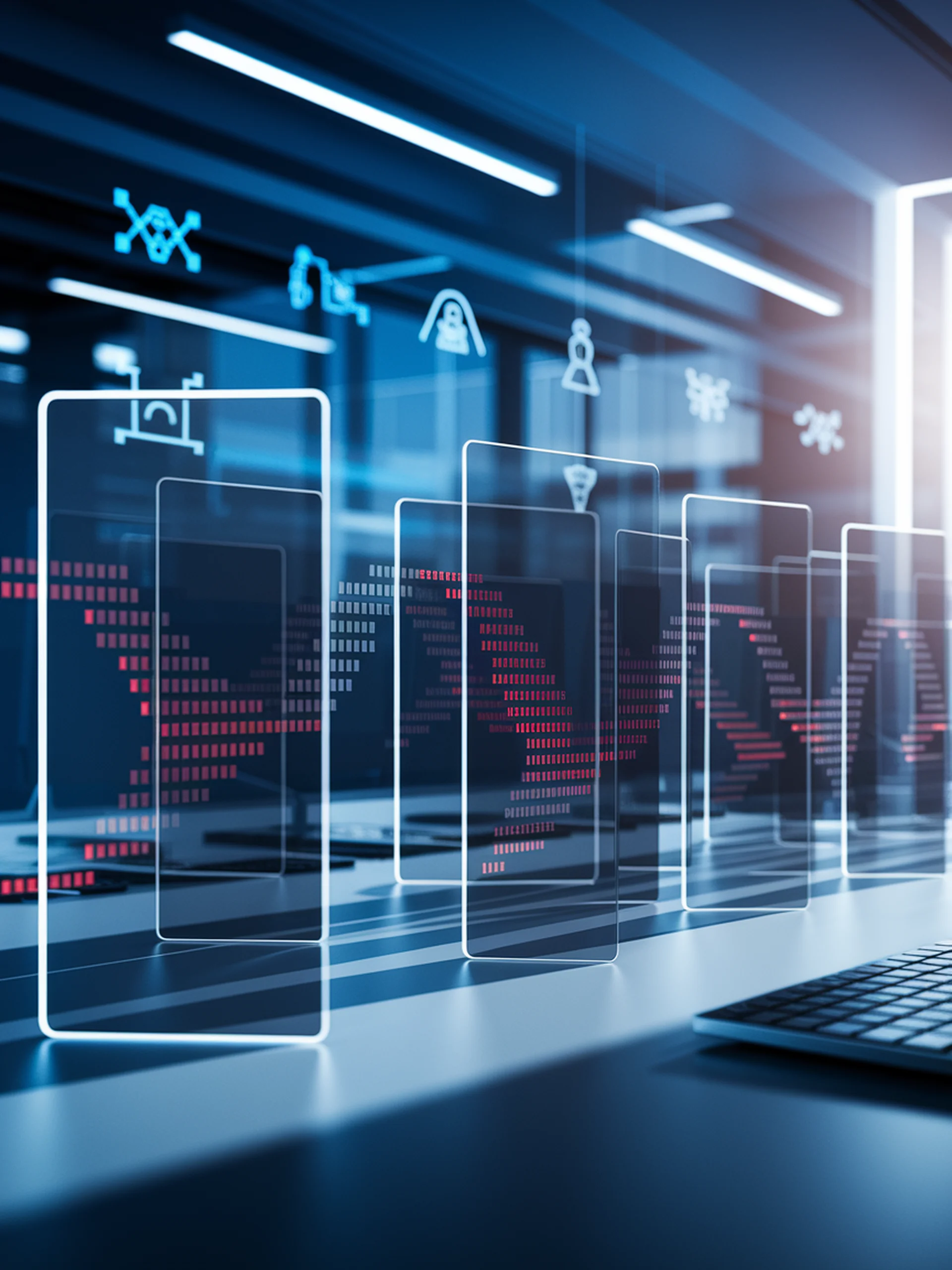
Redefining Privacy for AI Decision-Making
Why traditional privacy frameworks fail in the age of LLMs
This research introduces new privacy paradigms for sequential decision-making AI systems where sensitive information emerges from patterns over time, not just isolated data points.
- Identifies unique privacy challenges in reinforcement learning (RL) applications, especially in federated RL and RLHF for large language models
- Explains how temporal patterns and behavioral strategies create novel privacy vulnerabilities
- Proposes frameworks that better protect privacy in collaborative AI learning environments
- Highlights critical implications for high-stakes domains like healthcare and finance
For security professionals, this work addresses fundamental gaps in current privacy protection approaches as AI systems become increasingly deployed in sensitive contexts.
Position Paper: Rethinking Privacy in RL for Sequential Decision-making in the Age of LLMs