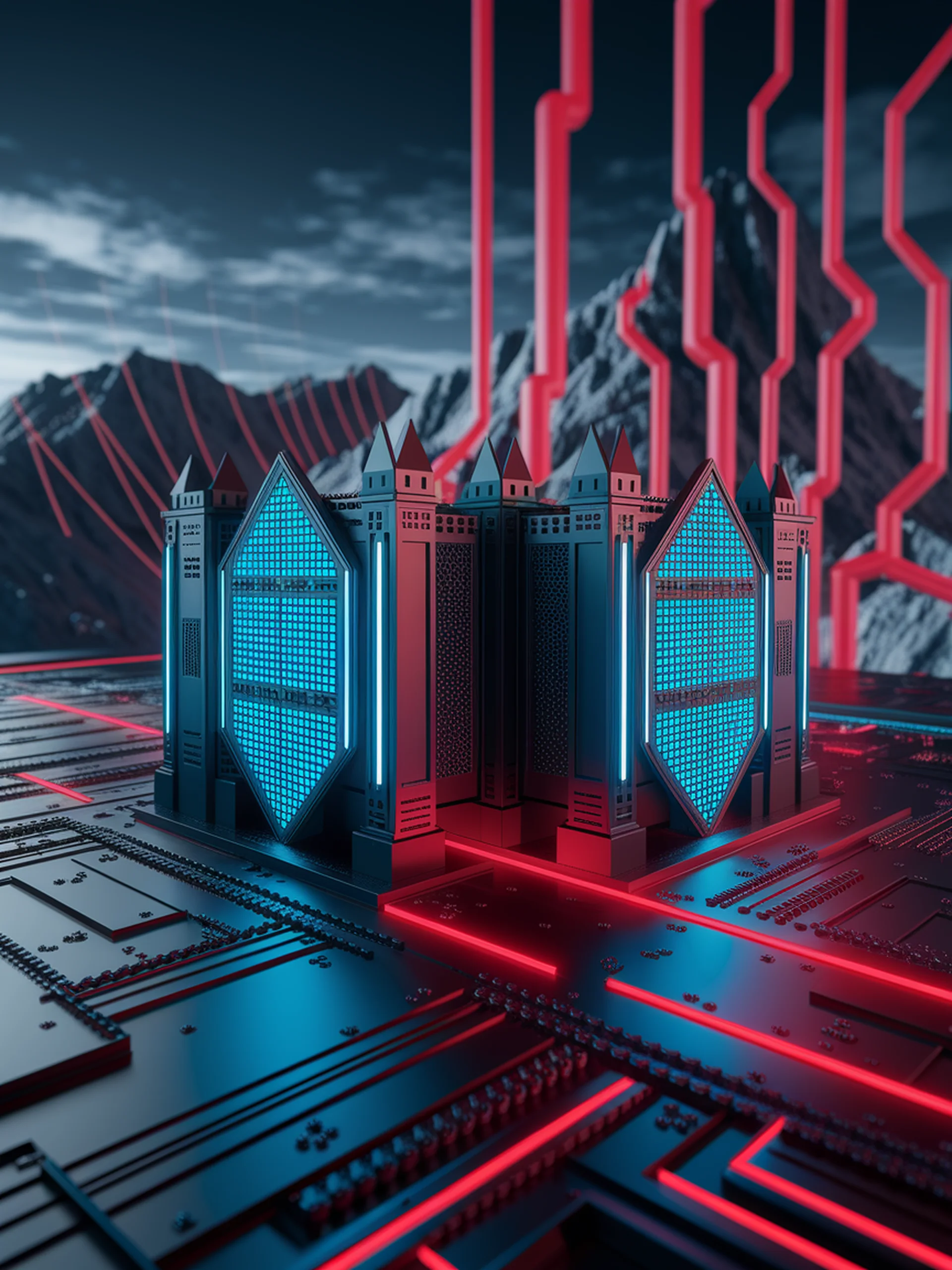
Defending LLMs Against Prompt Injection
First benchmark for evaluating and mitigating indirect prompt injection attacks
This research introduces BIPIA, the first comprehensive benchmark for assessing how vulnerable LLMs are to indirect prompt injection attacks when processing external content.
- Evaluates 16 popular LLMs, revealing widespread vulnerability to manipulated inputs from external sources
- Identifies that smaller, open-source models are generally more vulnerable than larger, proprietary models
- Proposes novel defense mechanisms that can reduce attack success rates by up to 49%
- Establishes a standardized evaluation framework for security researchers and LLM developers
This work addresses a critical security gap as organizations increasingly deploy LLMs that process external content, helping prevent attackers from hijacking model outputs through maliciously crafted content.
Benchmarking and Defending Against Indirect Prompt Injection Attacks on Large Language Models