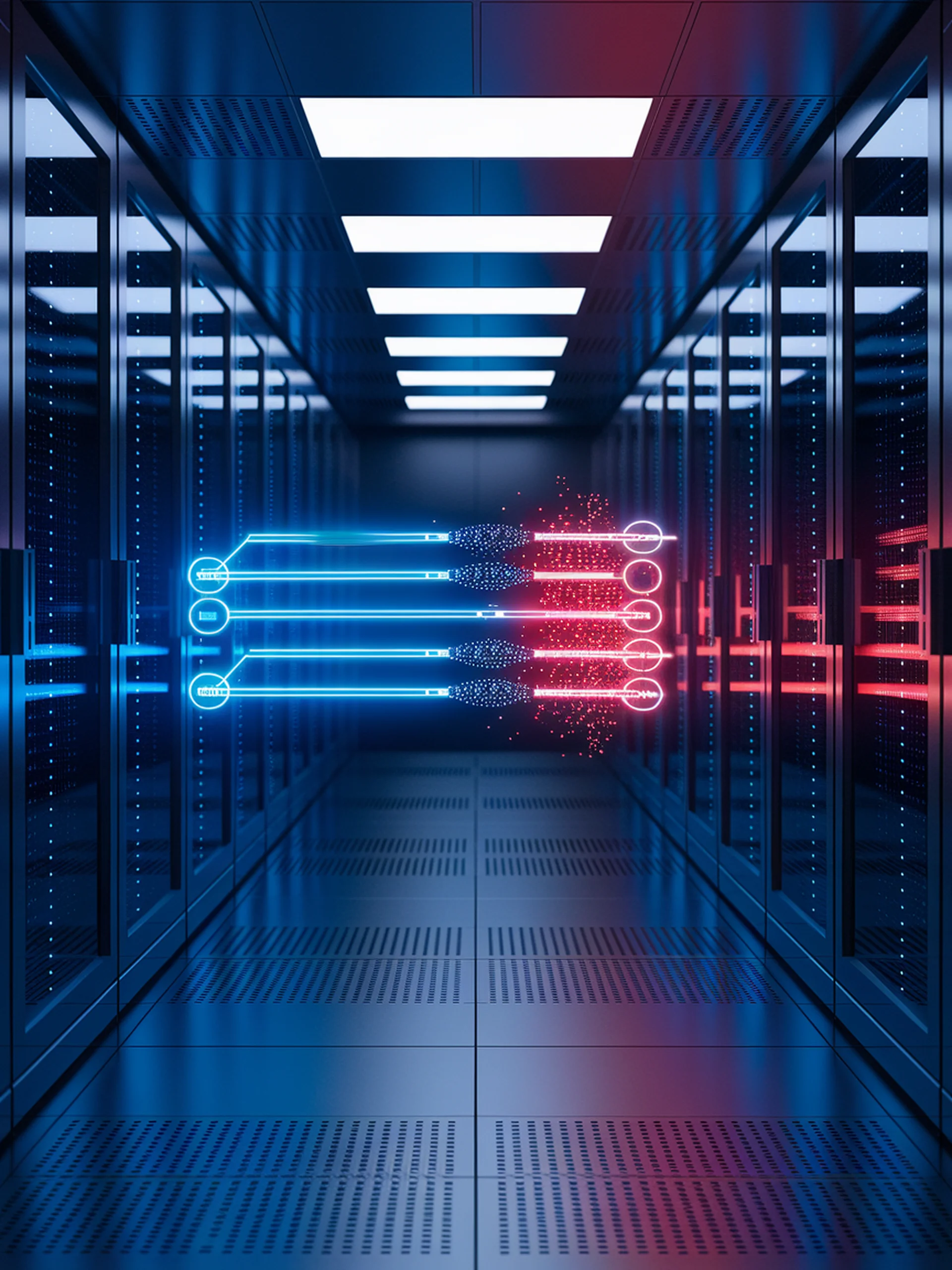
Dynamic Command Attacks in LLM Tool Systems
How AutoCMD exploits tool-learning vulnerabilities for information theft
This research reveals how adversaries can dynamically generate malicious commands that adapt to user interactions with LLM tool-learning systems, creating serious privacy risks.
- Introduces AutoCMD, a novel attack approach that generates adaptive commands to extract sensitive information
- Demonstrates how compromised tools can manipulate LLMs into sending private data to malicious endpoints
- Shows these attacks can bypass traditional static detection methods by continuously evolving commands
- Proposes defense mechanisms including input sanitization and restricting tool execution privileges
As organizations increasingly adopt LLMs with tool capabilities, understanding these security vulnerabilities becomes critical for protecting sensitive information and maintaining user trust.