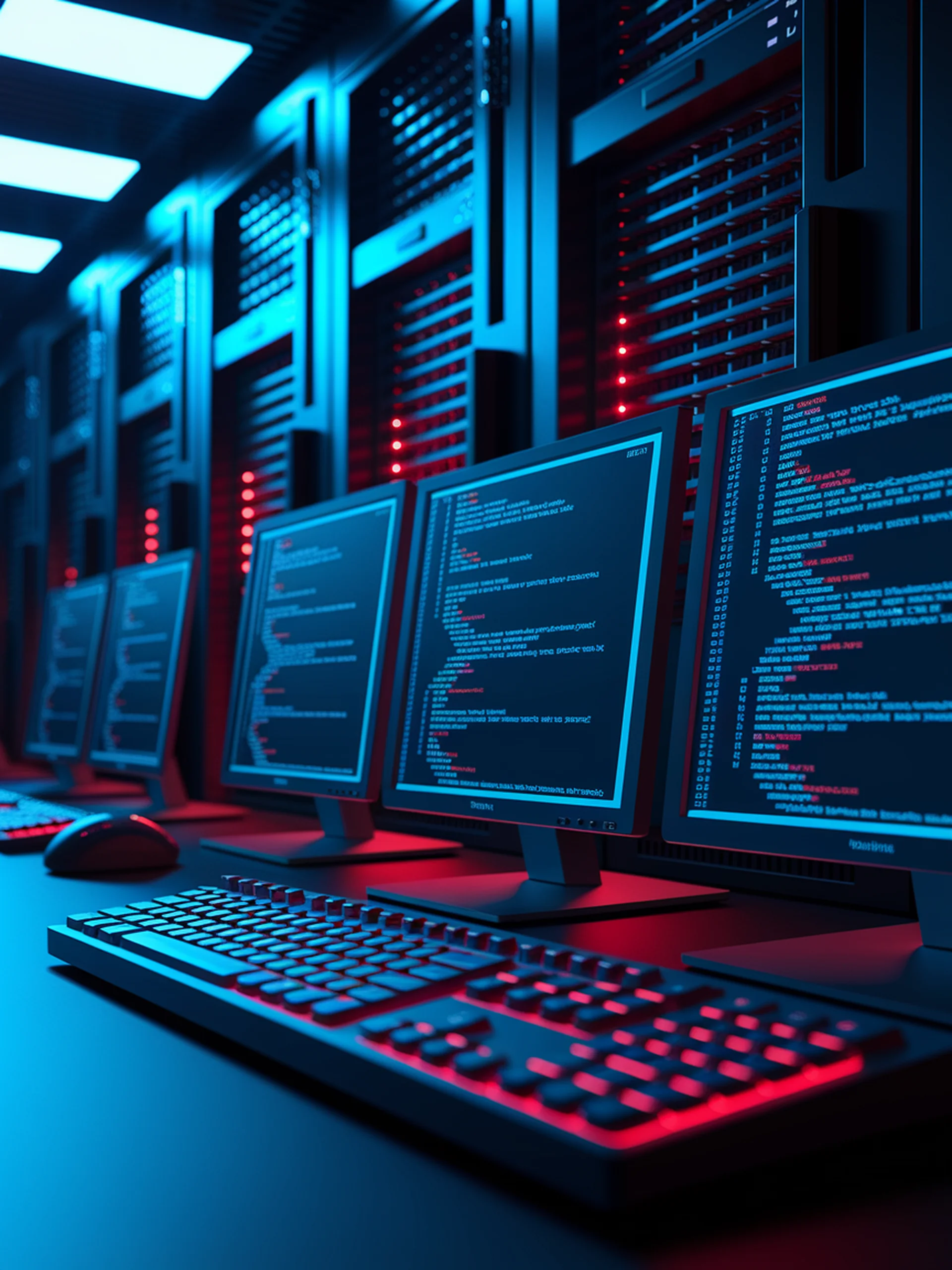
Hidden Threats in Text-to-SQL Models
Uncovering backdoor vulnerabilities in language models
This research reveals how backdoor attacks can inject SQL injection vulnerabilities into LLM-based Text-to-SQL models through poisoned training data.
- Demonstrates how attackers can create persistent SQL injection threats triggered by specific inputs
- Presents ToxicSQL, a framework for generating poisoned examples that maintain high utility while embedding malicious behavior
- Finds Text-to-SQL models are highly susceptible to backdoor attacks with attack success rates over 90%
- Proposes initial detection and defense methods against these security threats
This research highlights critical security gaps as organizations increasingly adopt LLM-based database interfaces, emphasizing the need for robust security measures before deployment.
ToxicSQL: Migrating SQL Injection Threats into Text-to-SQL Models via Backdoor Attack