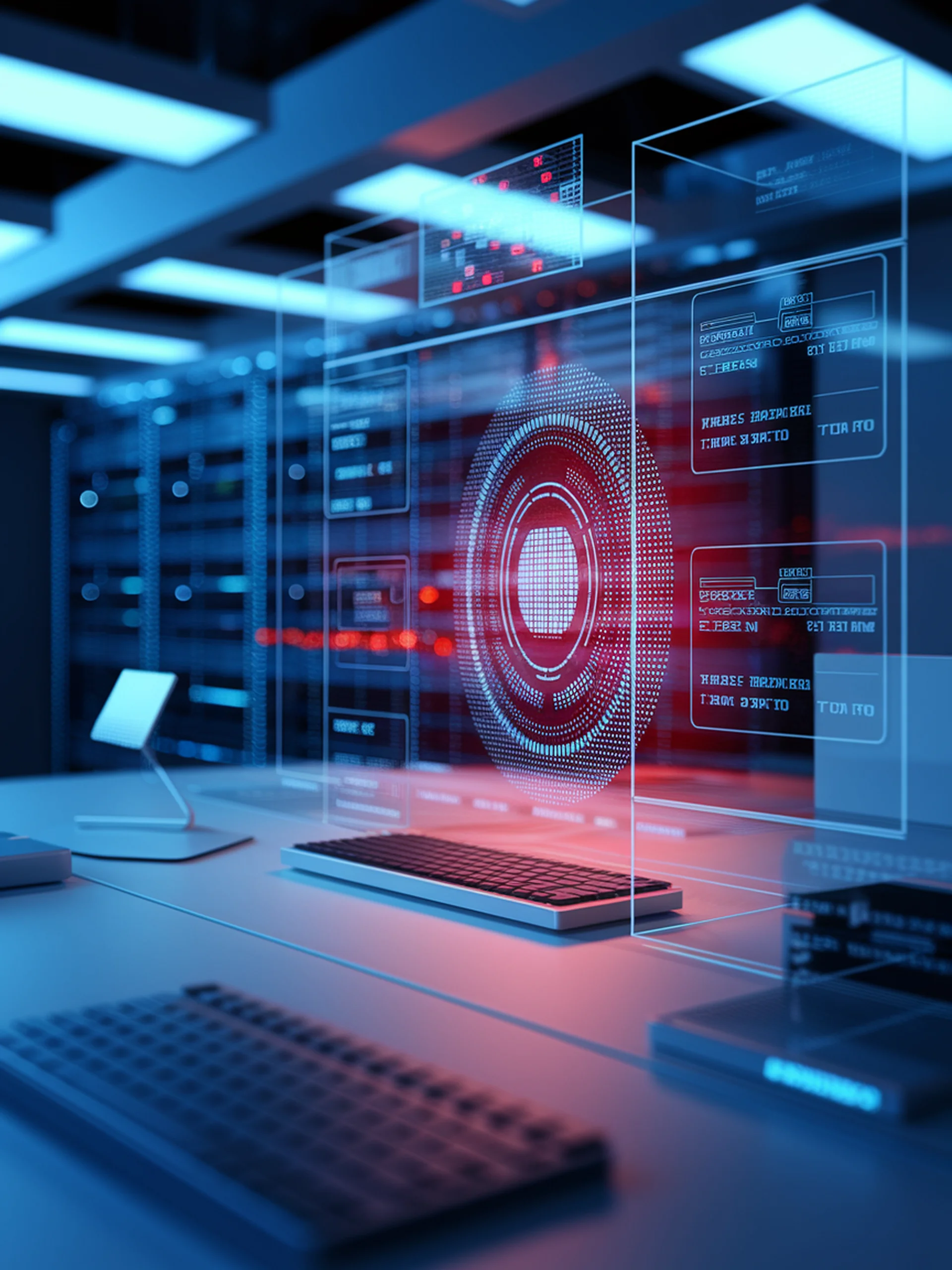
Securing LLM Agents Against Prompt Injections
CaMeL: A Novel Defense System for LLM-Based Applications
CaMeL introduces a protective system layer around Large Language Models that secures them from prompt injection attacks when handling untrusted data.
- Creates a clear separation between control flow and data flow in LLM queries
- Provides security even when underlying models remain vulnerable to attacks
- Demonstrated effectiveness through the AgentDojo security benchmark
- Offers a practical defense-by-design approach for LLM-based applications
This research addresses critical security vulnerabilities in agentic LLM systems, enabling safer deployment of AI assistants and automated systems that interact with external environments and untrusted data sources.