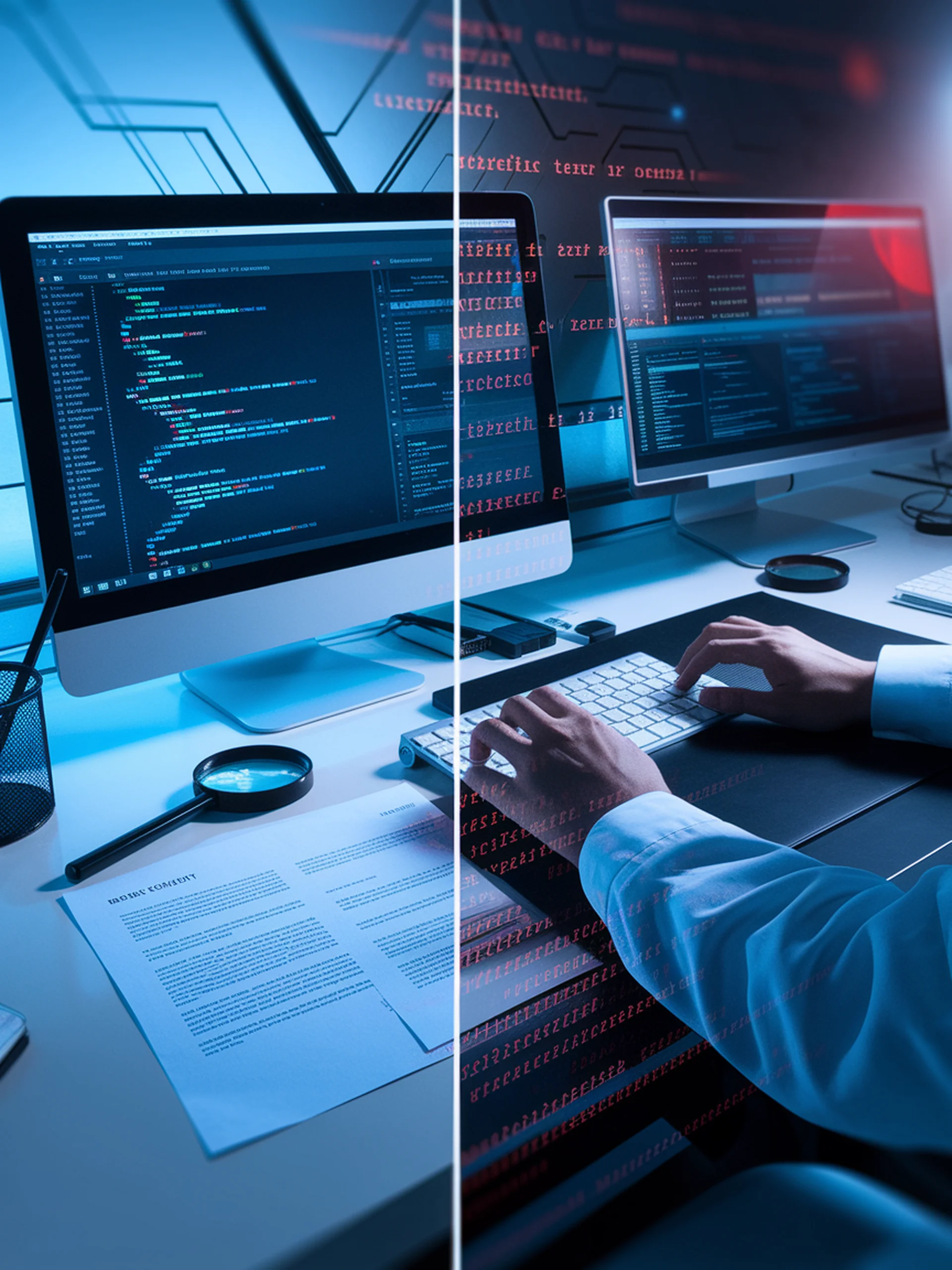
Uncovering Prompt Vulnerabilities in Style Transfer
A benchmark dataset for reconstructing LLM style transformation prompts
This research introduces StyleRec, the first benchmark dataset focused on recovering prompts used for writing style transformation in LLMs, revealing potential security vulnerabilities.
- Presents a novel approach to prompt recovery focused on style transfer rather than Q&A contexts
- Demonstrates how prompts can be reconstructed from LLM outputs without access to internal model weights
- Establishes evaluation metrics and baselines for this security-critical task
- Highlights implications for API-based LLM services where users lack access to model internals
This research has significant security implications for organizations using LLM APIs, revealing potential privacy vulnerabilities where third parties might reverse-engineer proprietary prompts used in style transformation applications.
StyleRec: A Benchmark Dataset for Prompt Recovery in Writing Style Transformation