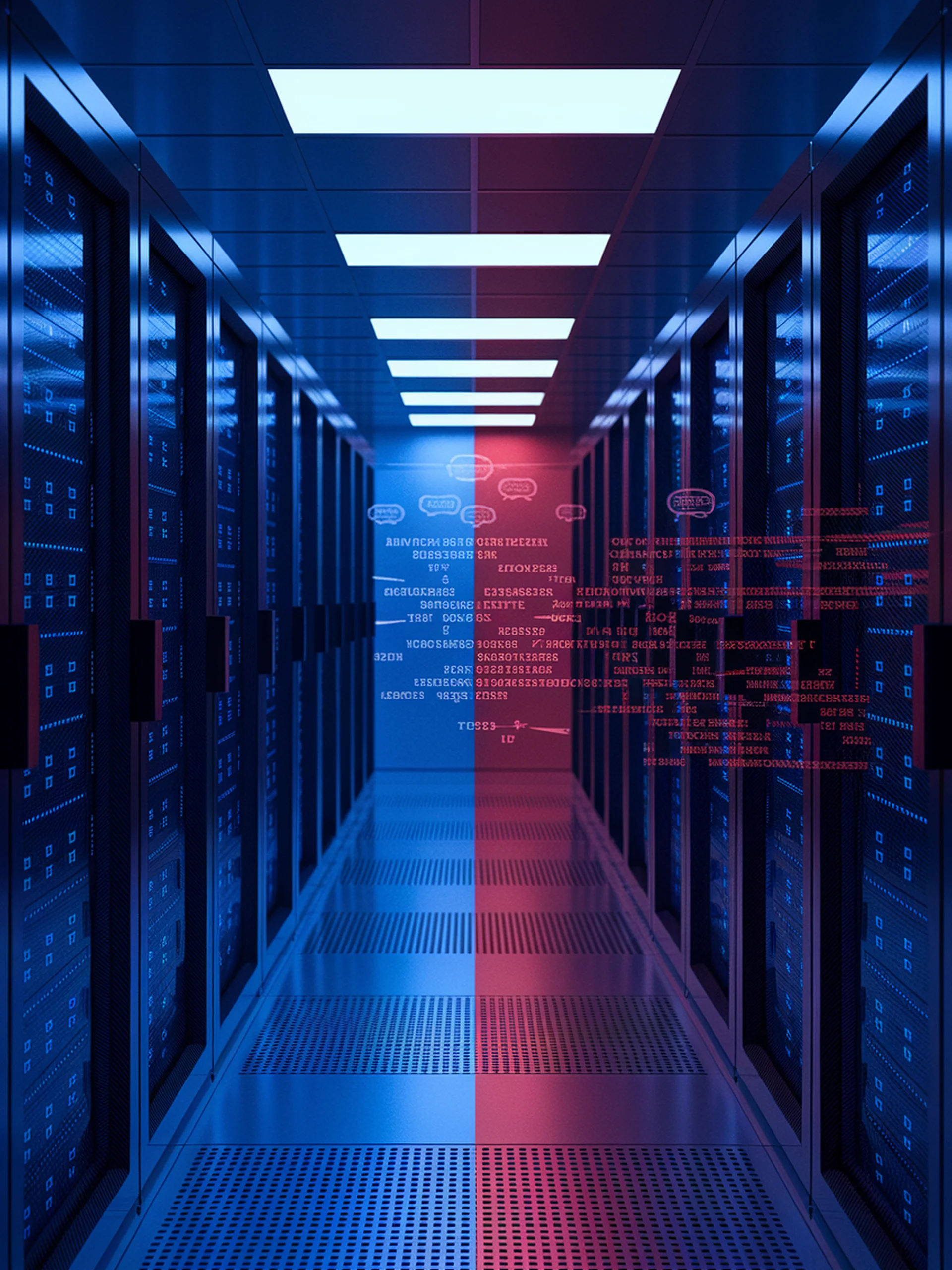
Separator Injection Attacks in LLMs
Uncovering security vulnerabilities in conversational AI
This research reveals how conversational LLMs can be manipulated through their role separator mechanisms, exposing critical security vulnerabilities.
- Role separators (used to distinguish participants in LLM conversations) create exploitable weaknesses
- Attackers can misuse these separators to override instructions, causing the model to deviate from intended behavior
- These vulnerabilities can lead to prompt injection attacks that bypass safety guardrails
- Simple changes in separator handling can significantly improve model security
This research highlights the importance of robust security design in conversational AI systems, as exploitation of these vulnerabilities could lead to misaligned AI behavior and potential harm.