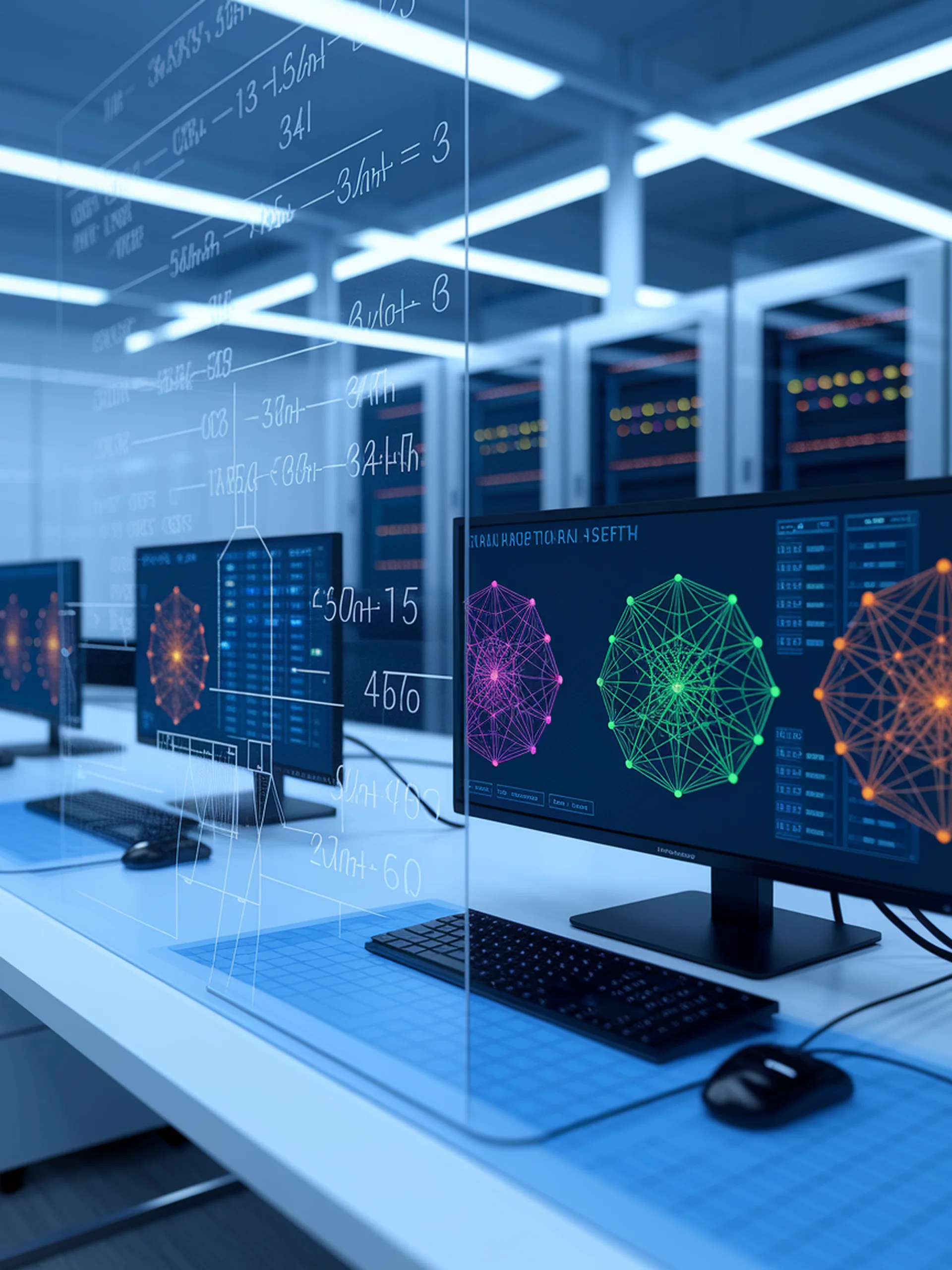
Smarter Safety Alignment for LLMs
Using entropy to improve multi-criteria safety evaluations
This research presents a novel entropy-guided approach for combining multiple safety criteria when evaluating and aligning large language models.
- Identifies that safety rules with high rating entropy (high disagreement) are less reliable in evaluations
- Proposes a multi-head reward aggregation method that uses entropy to adaptively weight different safety criteria
- Demonstrates improved performance on safety benchmarks compared to traditional weighting approaches
- Shows how to create more robust safety guardrails by intelligently combining human feedback signals
For security teams, this means more reliable safety alignment techniques that can better prioritize consistent, high-quality safety signals while minimizing the impact of noisy or subjective criteria.