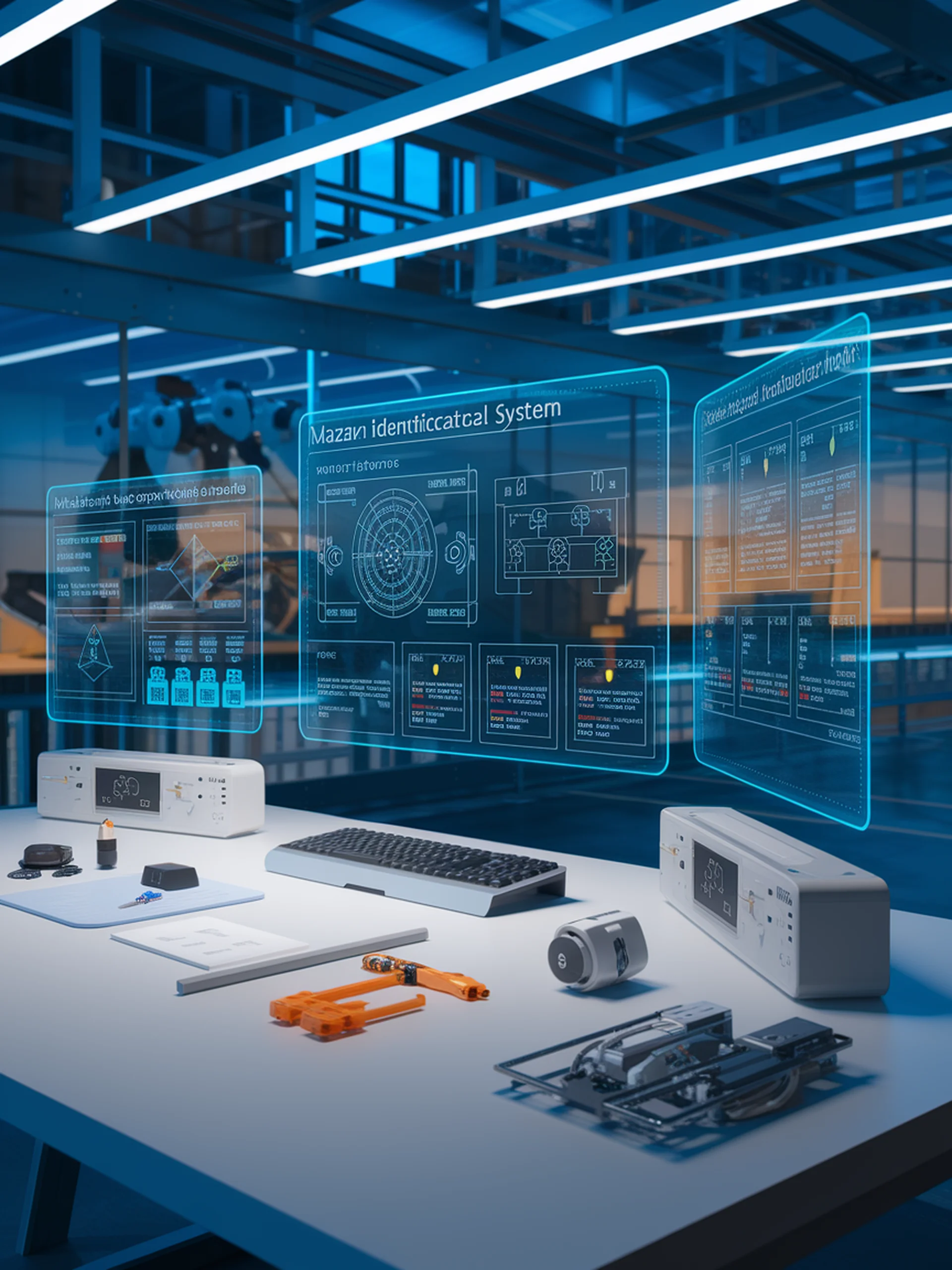
Proactive Safety for ML Systems
Using LLMs to enhance safety engineering for machine learning applications
This research introduces a framework for proactive hazard identification and mitigation in ML-powered systems using LLM assistance.
- Adapts traditional safety engineering methods (FMEA, STPA) specifically for ML applications
- Leverages LLMs to support hazard identification, causal analysis, and safety controller design
- Evaluates the approach through real-world case studies with ML practitioners
- Demonstrates how LLMs can enhance safety engineering by generating diverse hazard scenarios
By integrating safety engineering early in ML system development, organizations can identify potential risks before deployment, reducing harmful consequences and improving regulatory compliance.