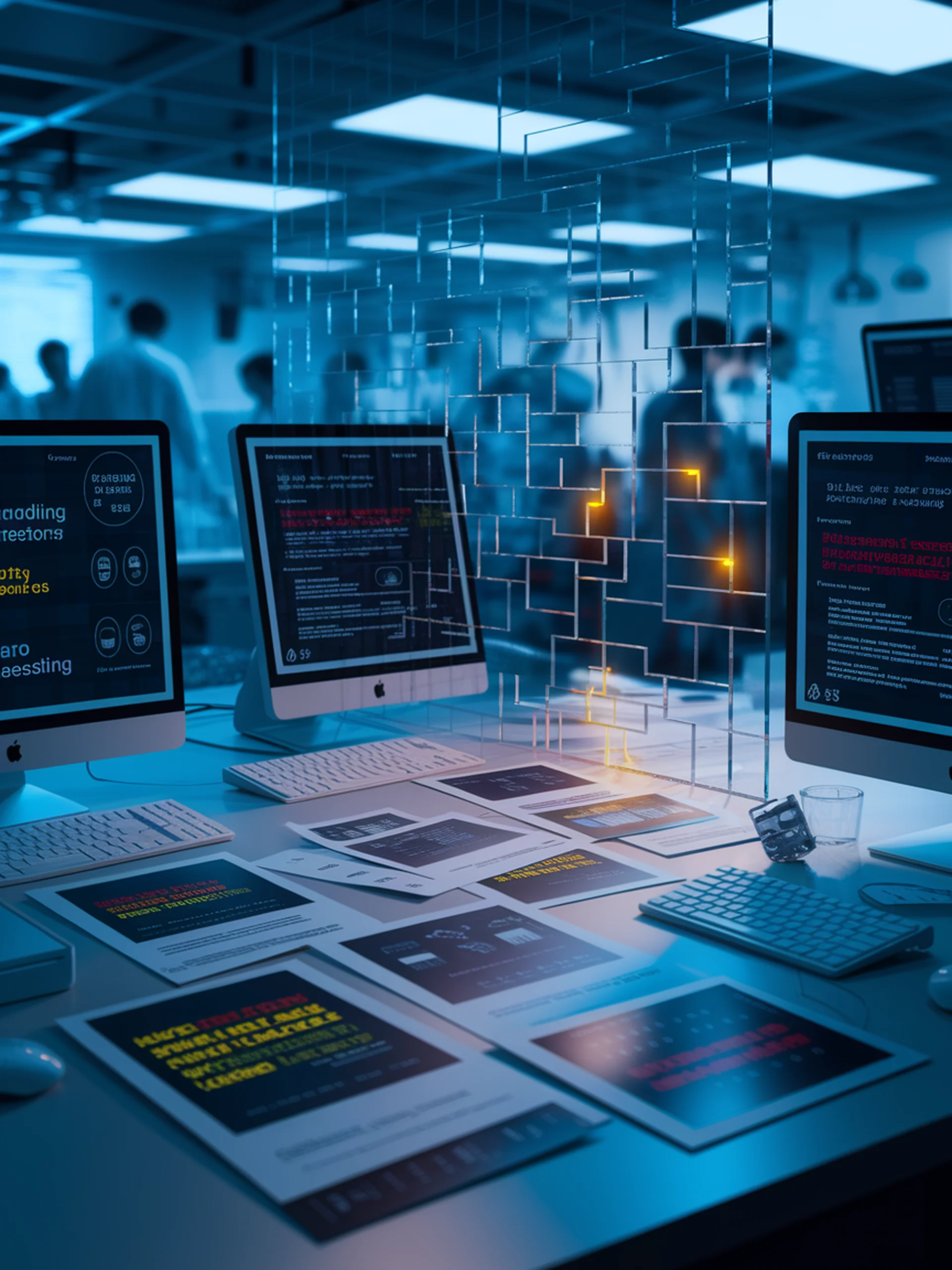
The Fragility of AI Safety Testing
Why Current LLM Safety Evaluations Need Improvement
This research reveals critical reliability issues in how we evaluate large language model safety, potentially undermining security efforts across the industry.
- Current evaluation methods suffer from multiple sources of noise, including small datasets and inconsistent methodologies
- These weaknesses make fair comparison between attacks and defenses nearly impossible
- The paper systematically analyzes the entire safety evaluation pipeline from dataset curation to red-teaming
- Improved evaluation robustness is essential for meaningful progress in AI security
For security professionals, this research highlights the urgent need to develop more standardized, robust evaluation frameworks before we can truly assess LLM vulnerability to attacks or the effectiveness of defensive measures.