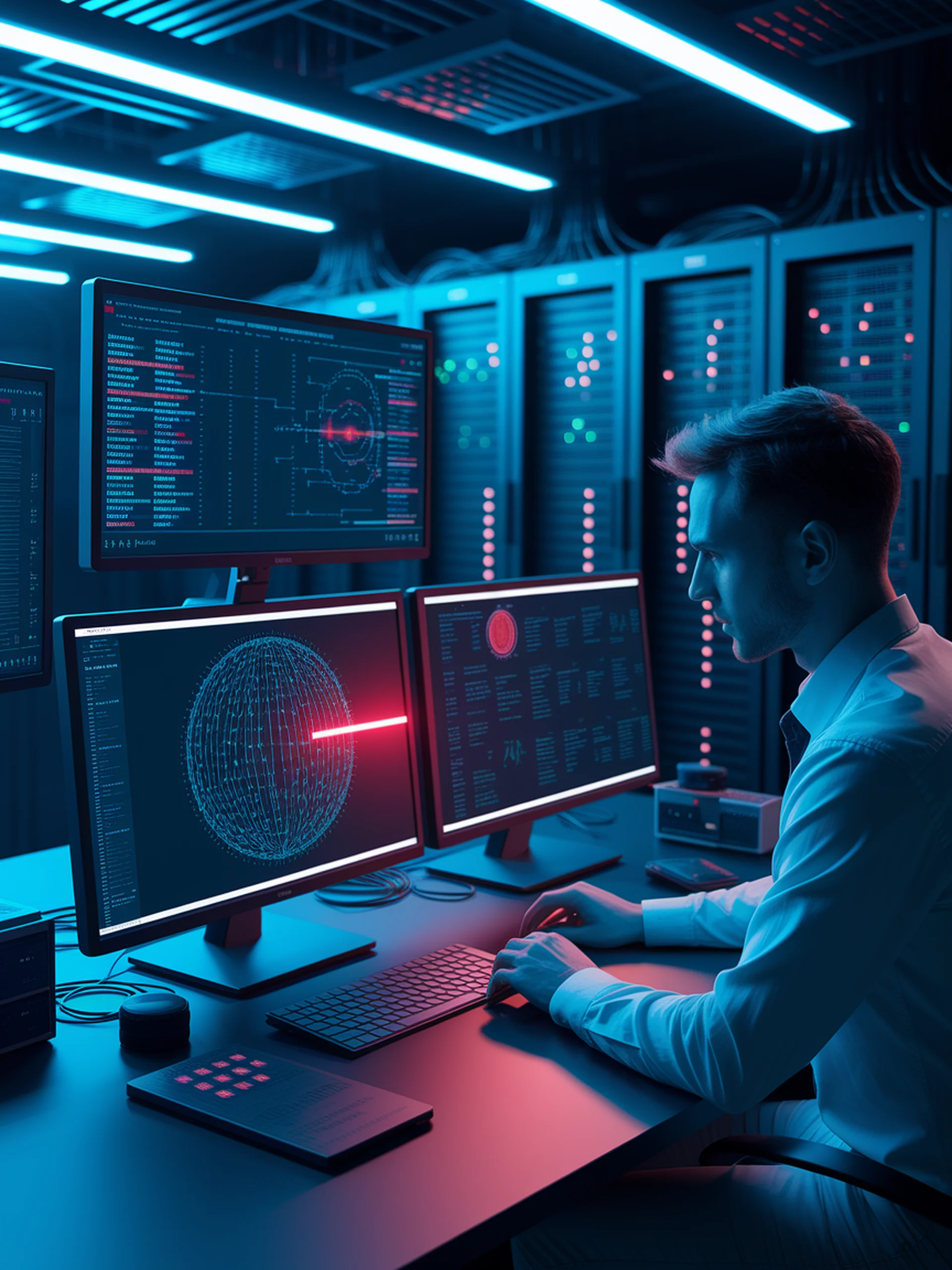
Blind Spots in AI Safety Judges
Evaluating the reliability of LLM safety evaluation systems
This research examines the robustness vulnerabilities in LLM-based safety judges that are critical for AI safety evaluation processes.
- In real-world conditions, LLM judges show significant prompt sensitivity and performance degradation under distribution shifts
- Researchers identified adversarial attacks that can manipulate safety judges, causing up to 90% false negative rates
- Safety judges can be fooled by specific prompting strategies that bypass harmful content detection
- Even with fine-tuning, safety judges remain vulnerable to relatively simple attacks
This research is crucial for security professionals as it reveals fundamental weaknesses in current AI safety mechanisms and highlights the need for more robust evaluation systems before deployment.
Know Thy Judge: On the Robustness Meta-Evaluation of LLM Safety Judges