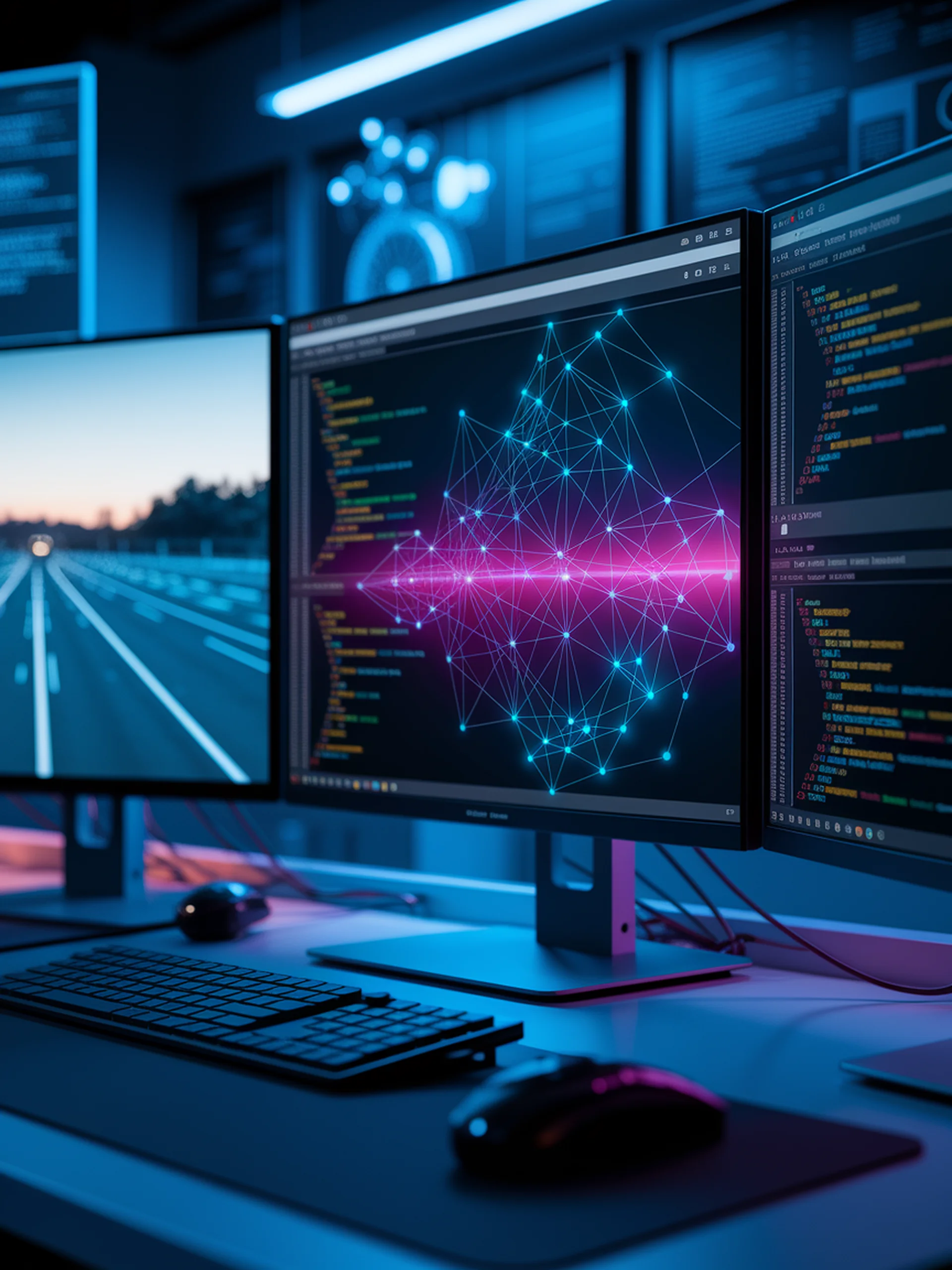
Testing DNNs Without Ground Truth
Using GANs to enable simulator-based testing for safety-critical systems
This research tackles a critical challenge in DNN testing: how to effectively test computer vision systems when simulators cannot provide ground-truth data for verification.
- Introduces a GAN-enhanced approach that generates synthetic inputs for testing computer vision DNNs
- Enables cost-effective testing of DNNs in safety-critical applications
- Creates a simulation-driven framework that works without ground-truth data
- Bridges the gap between simulation capabilities and verification requirements
For security professionals, this research provides a novel solution for verifying neural networks in autonomous systems where traditional testing approaches fail due to ground-truth limitations, helping ensure system reliability in critical applications.
GAN-enhanced Simulation-driven DNN Testing in Absence of Ground Truth