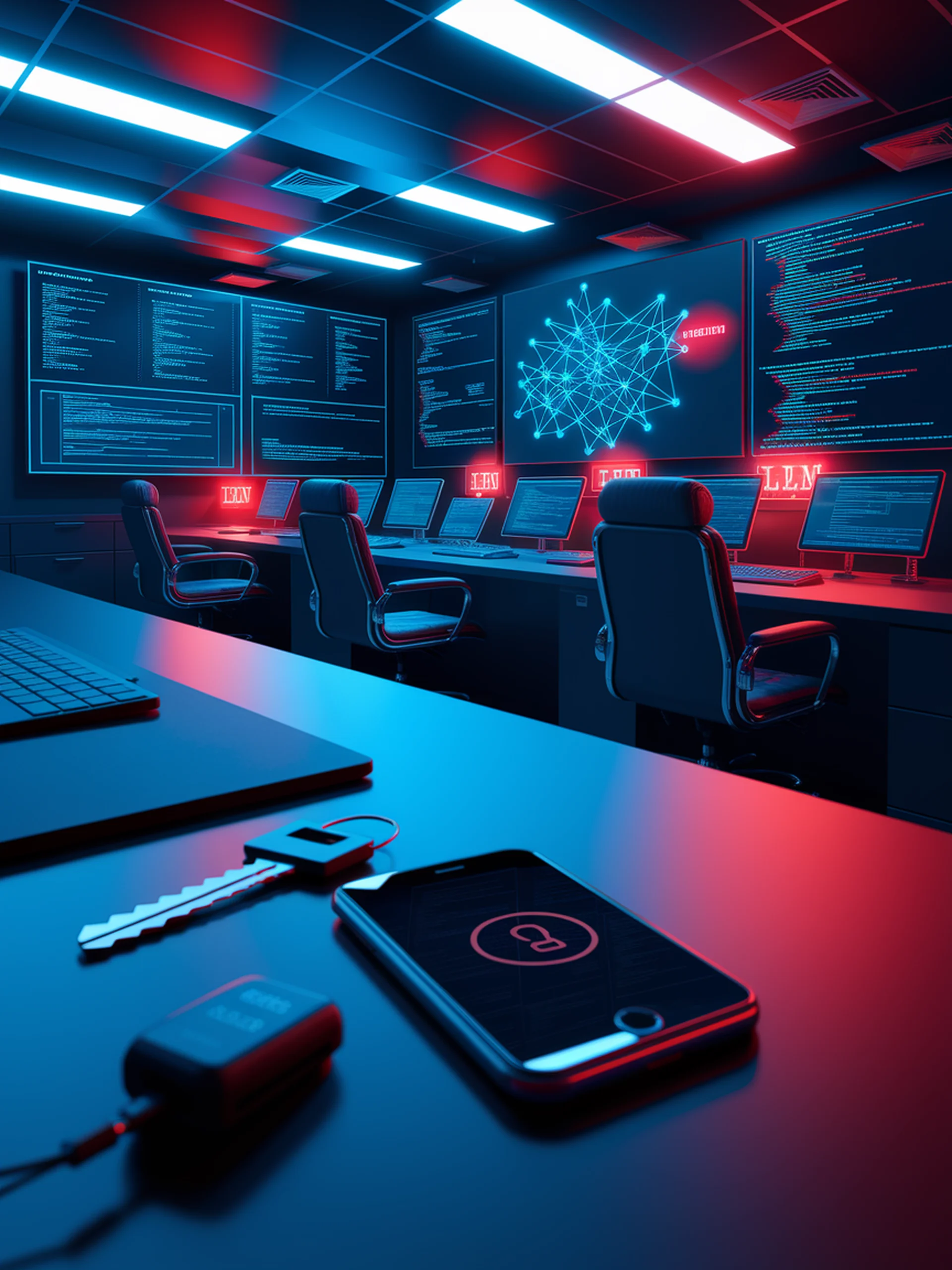
Vulnerabilities in LLM Tool Selection
New attack method exploits how LLMs choose tools
This research reveals critical security risks in how large language models select tools, demonstrating a practical attack method that manipulates tool selection decisions.
- Introduces a black-box text attack that can force LLMs to select specific tools regardless of appropriateness
- Shows that even leading models like GPT-4 and Claude are vulnerable to these manipulations
- Reveals how attackers can exploit tool selection without needing access to model parameters
- Highlights the urgent need for improved security measures in tool-augmented LLM systems
This security research matters because as LLMs increasingly rely on external tools for specialized tasks, vulnerabilities in tool selection could enable attackers to trigger malicious tools or bypass safety mechanisms.
Select Me! When You Need a Tool: A Black-box Text Attack on Tool Selection