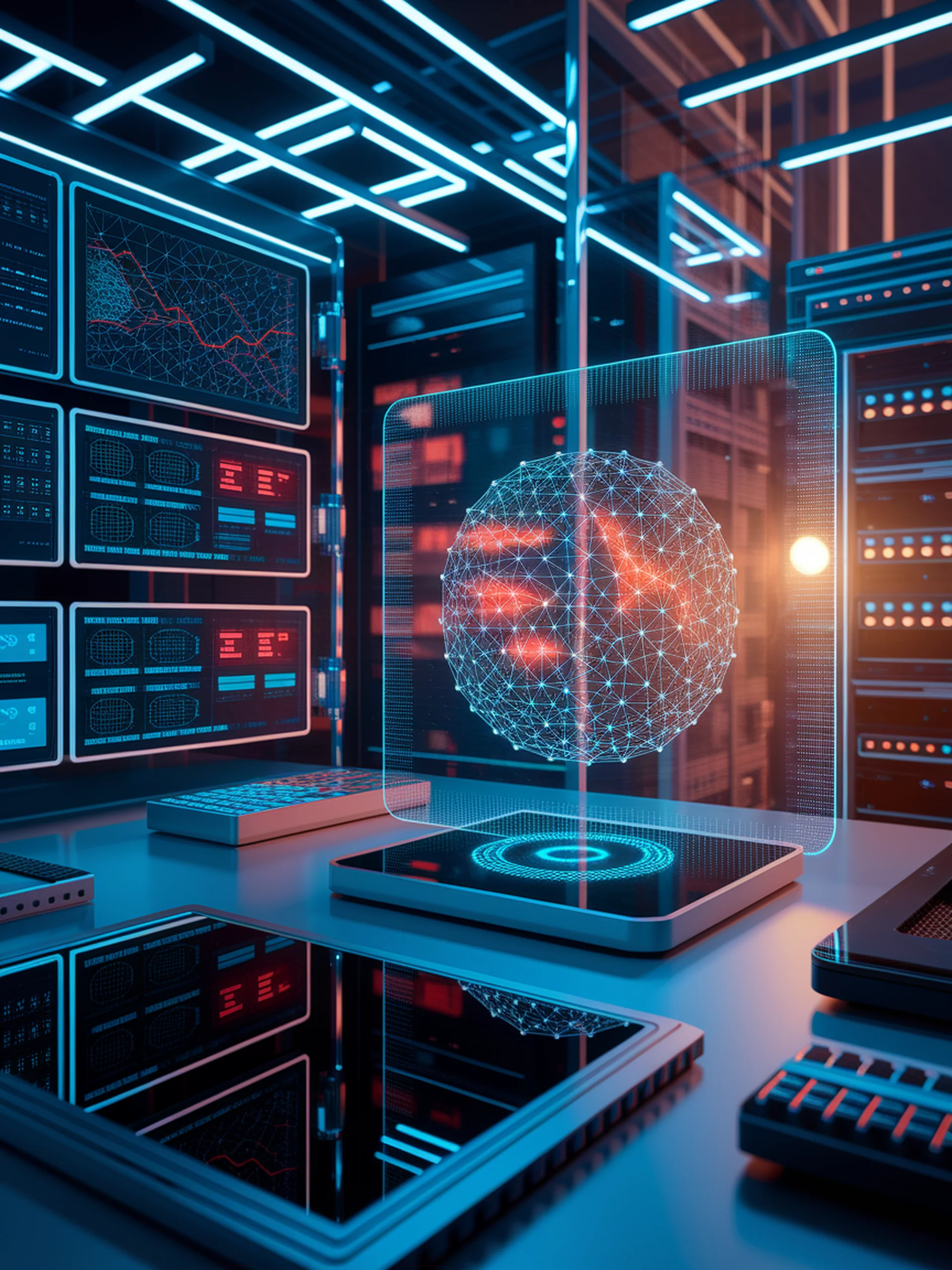
Detecting LLM Contamination
Safeguarding model integrity and evaluation reliability
This survey addresses the critical challenge of data contamination in Large Language Models where exposure to test data can artificially inflate performance metrics.
- Contamination detection is becoming increasingly important as benchmarks directly impact business valuations
- The paper systematically categorizes detection methods to help researchers identify when models have been exposed to test data
- Highlights the security implications of contaminated models in high-stakes applications
- Provides a framework for reliable model evaluation in an era of massive pretraining datasets
For security professionals, this research offers vital tools to verify model integrity, ensure fair comparisons, and prevent deceptive performance claims in the competitive AI landscape.
A Comprehensive Survey of Contamination Detection Methods in Large Language Models