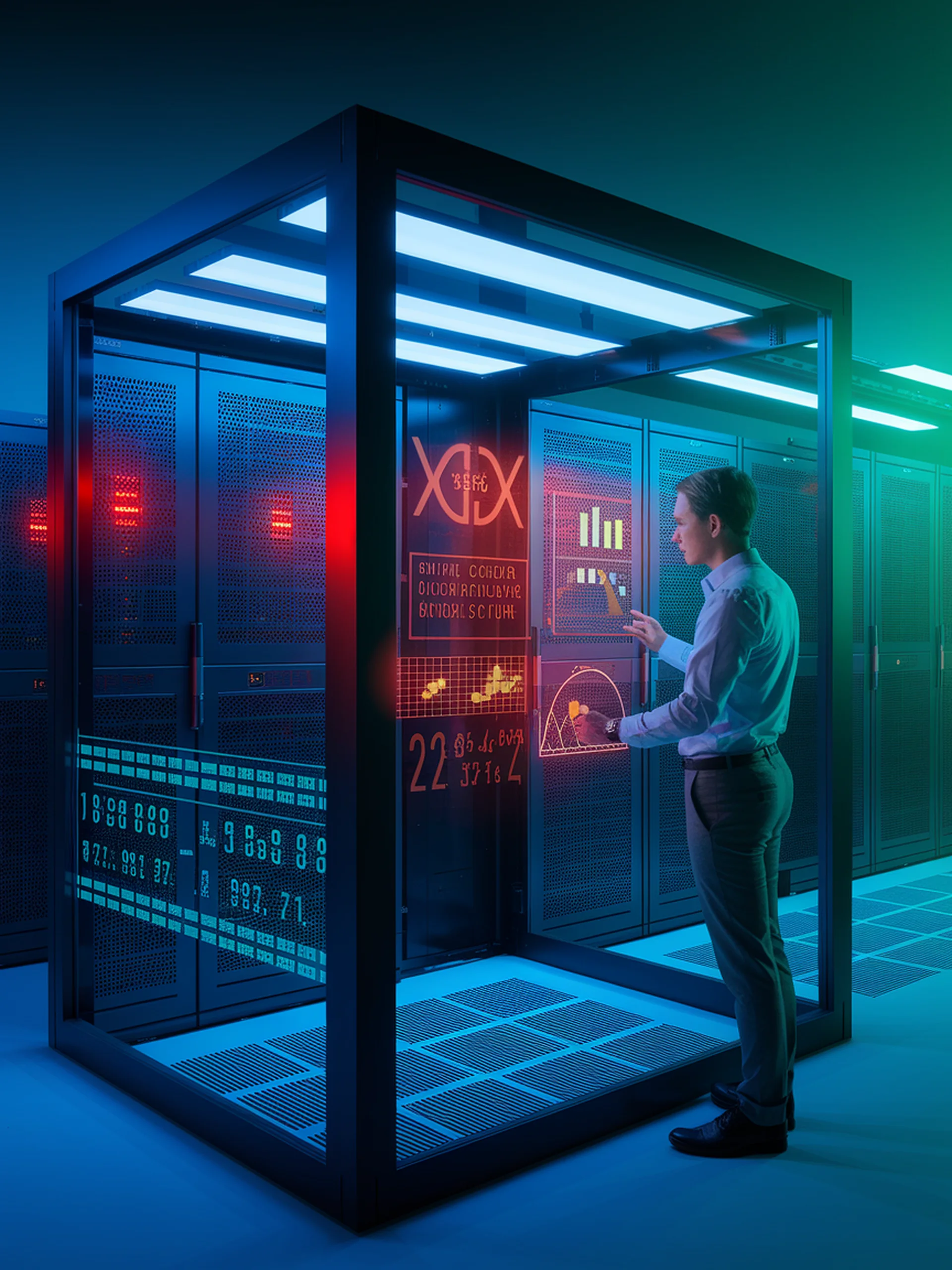
Building Trust in Black-Box LLMs
A Framework for Confidence Estimation Without Model Access
This research develops a practical framework for estimating the reliability of large language model outputs without requiring access to model internals.
- Leverages engineered features to train simple, interpretable models that predict LLM response confidence
- Achieves high accuracy (up to 80%) in identifying correct vs. incorrect responses
- Demonstrates effectiveness across multiple domains including code generation and reasoning tasks
- Provides actionable trust signals while maintaining model privacy
Security Relevance: This approach enables organizations to deploy LLMs with greater confidence by identifying potentially unreliable outputs, reducing risk without requiring model providers to expose proprietary information.
Large Language Model Confidence Estimation via Black-Box Access