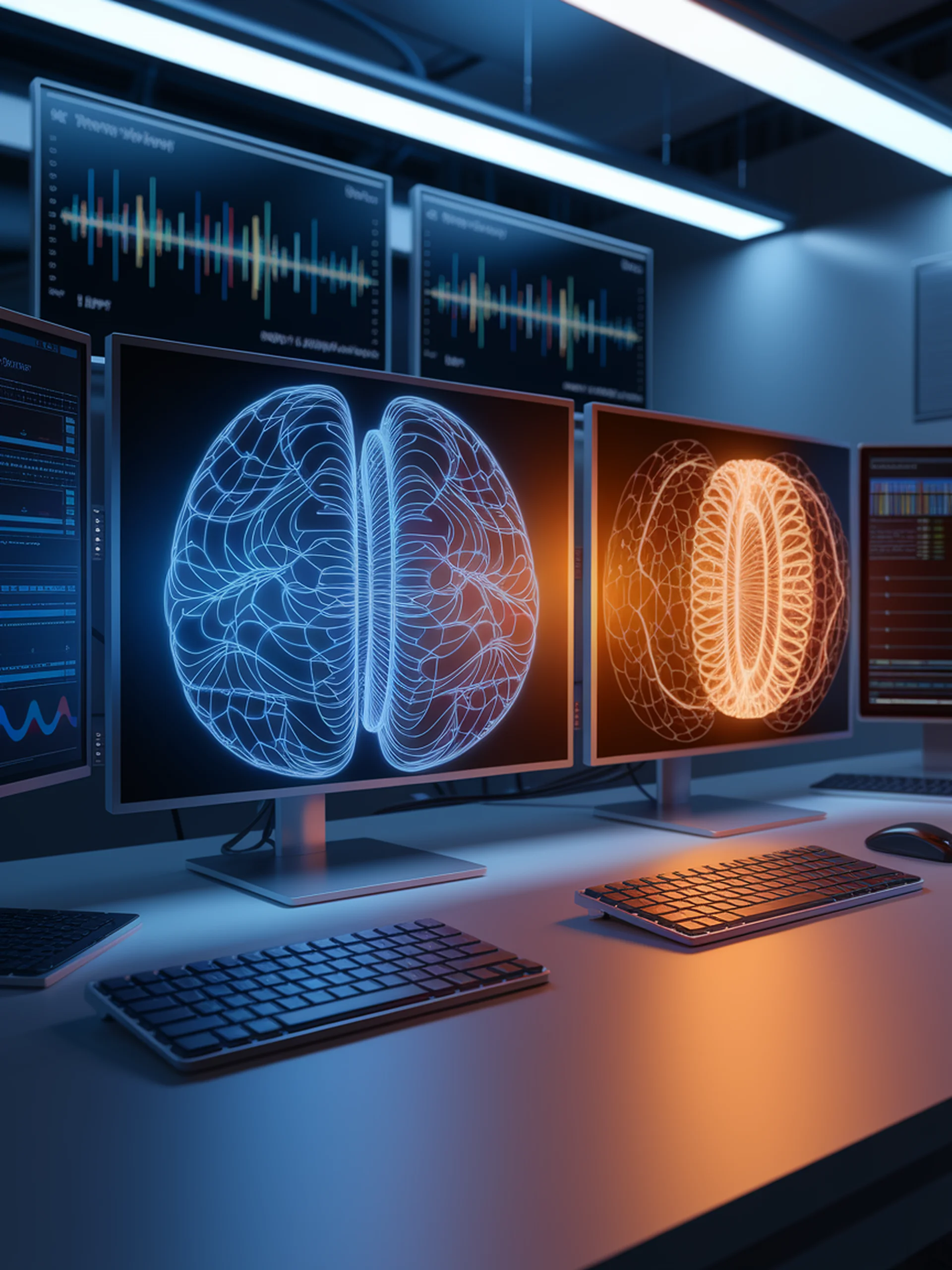
Architectural Influences on LLM Hallucinations
Comparing self-attention vs. recurrent architectures for reliability
This research examines how different LLM architectural designs impact hallucination behaviors, with important implications for security and reliability.
- Self-attention models and recurrent neural networks exhibit different hallucination patterns
- Architecture choices significantly affect the types and frequency of model hallucinations
- Different inductive biases in model design create varying vulnerability patterns
- Understanding these patterns can lead to more robust LLM implementations
Security Significance: As LLMs become integrated into critical systems, architectural choices directly impact reliability and the potential for generating harmful misinformation. This research provides insights for designing safer AI systems with reduced hallucination risks.