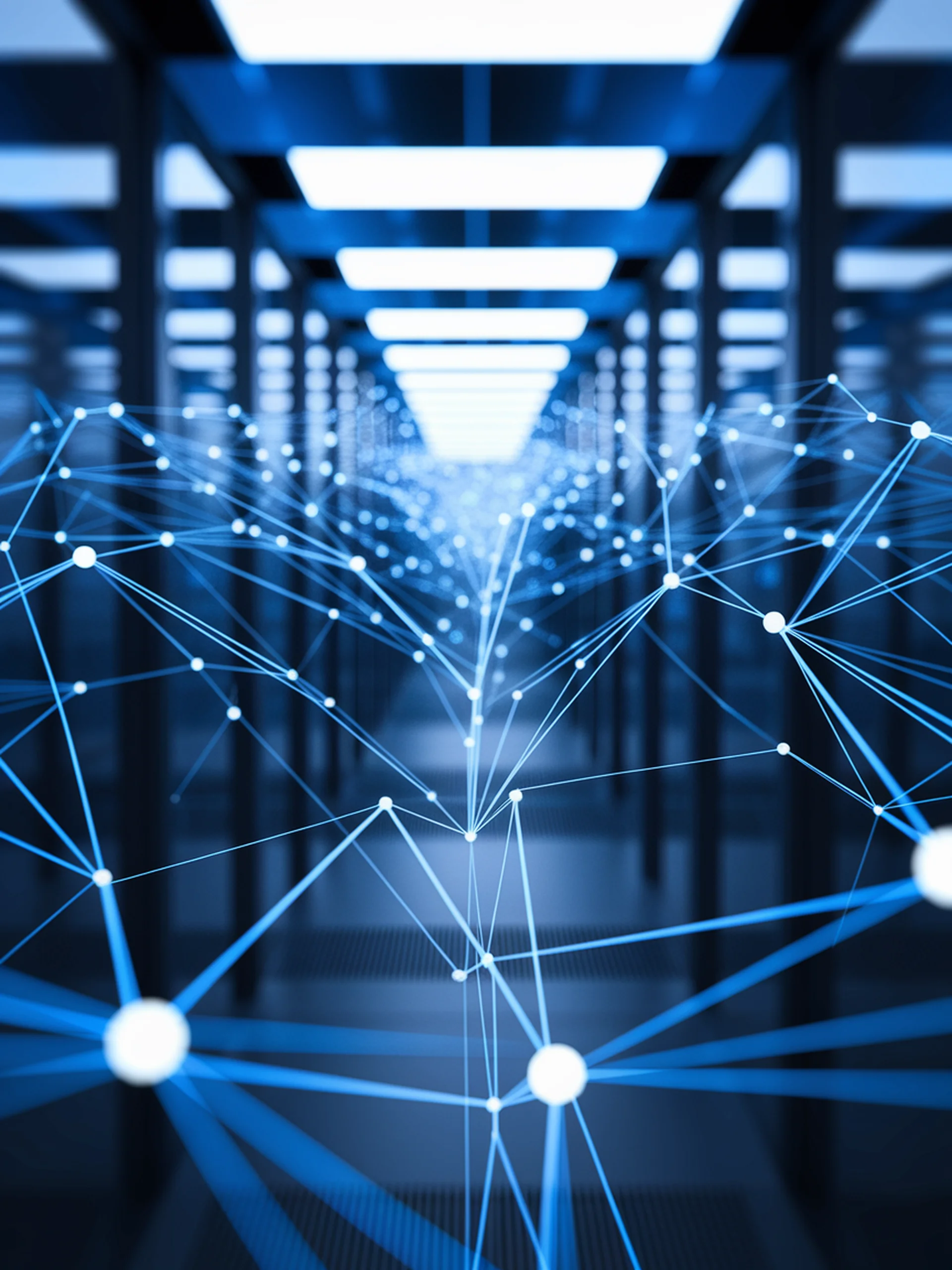
Reducing Hallucinations in LLMs
Zero-shot detection through attention-guided self-reflection
The AGSER approach leverages attention patterns within LLMs to detect and reduce hallucinations without requiring additional training or external models.
- Separates input queries into attentive and non-attentive categories based on attention contribution
- Processes each query type separately to compute consistency scores between responses
- Achieves superior performance compared to existing methods, particularly for challenging queries
- Demonstrates effectiveness across multiple LLM architectures including GPT and Llama models
This research addresses a critical security challenge for enterprise LLM deployment by improving the reliability of AI-generated content without additional computational overhead.
Attention-guided Self-reflection for Zero-shot Hallucination Detection in Large Language Models