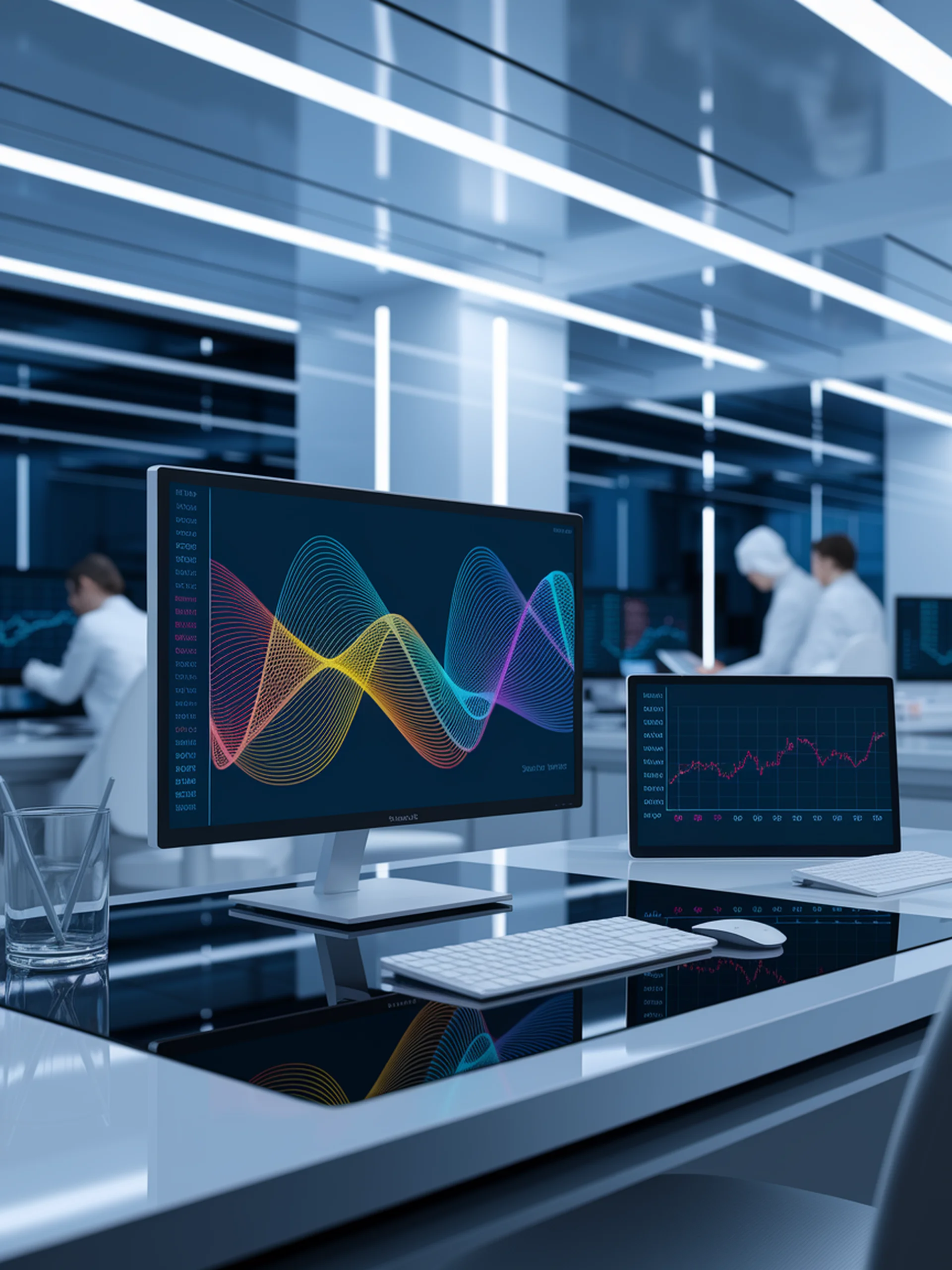
Detecting LLM Hallucinations Through Logit Analysis
A novel approach to measuring AI uncertainty and improving reliability
This research introduces a logit-based uncertainty estimation method that outperforms traditional probability-based approaches for identifying when language models might be hallucinating.
- Analyzes critical token reliability through direct examination of model logits
- Demonstrates superior performance in detecting uncertainty compared to probability-based methods
- Provides a practical framework for identifying potential AI hallucinations
- Enhances security by helping systems recognize when they lack sufficient knowledge
From a security perspective, this approach enables more robust AI deployments by allowing systems to flag potentially unreliable outputs, reducing risks of misinformation and improving user trust.