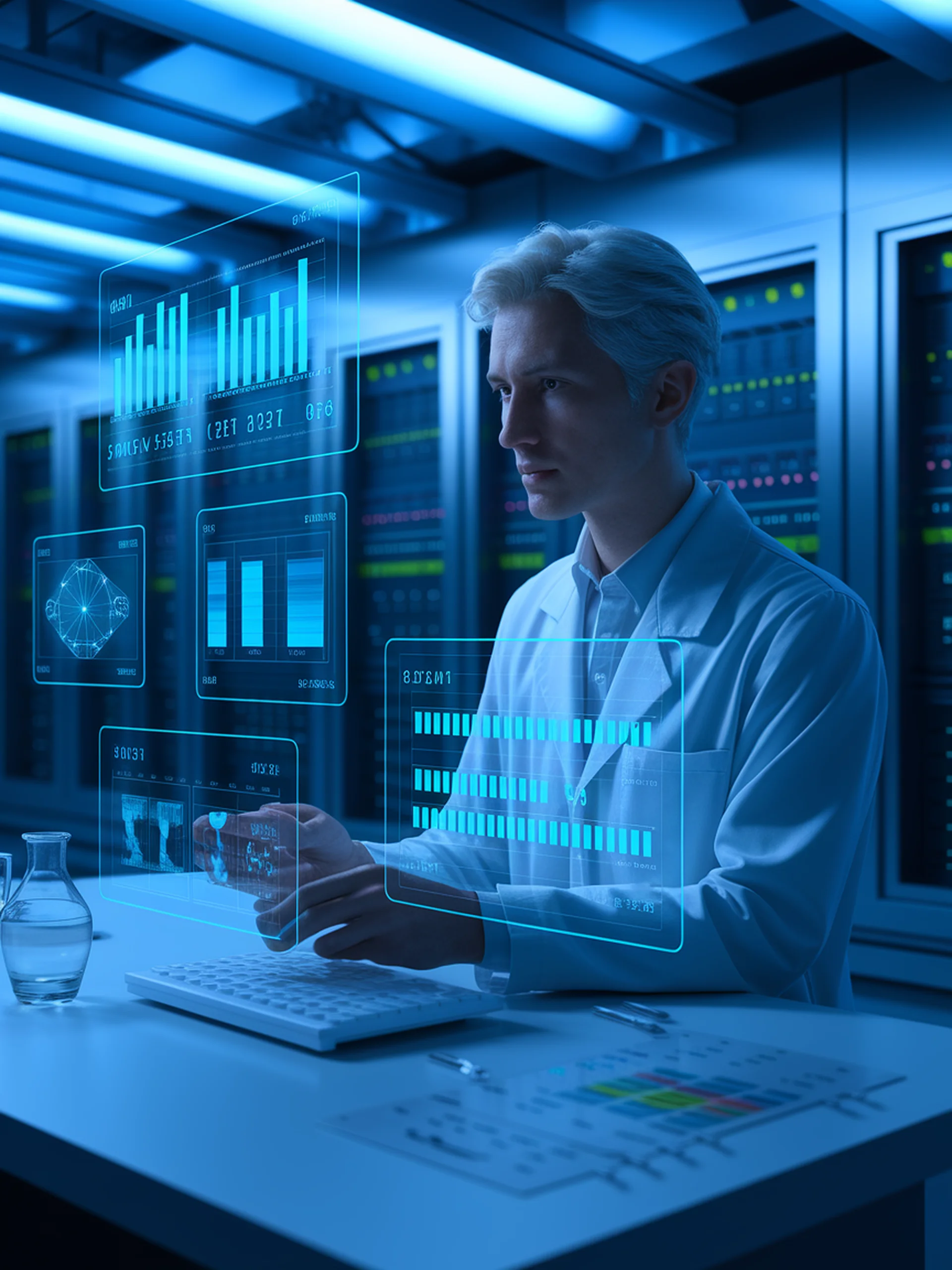
Better Confidence in AI Outputs
A new framework for evaluating how LLMs assess their own reliability
MCQA-Eval introduces a robust methodology to evaluate confidence estimation in Large Language Models, addressing critical concerns in high-stakes domains.
- Replaces noisy heuristic-based correctness functions with gold-standard correctness labels
- Transforms natural language generation tasks into multiple-choice question answering for more reliable evaluation
- Provides a fairer comparison framework for confidence estimation methods
- Reduces evaluation costs while increasing reliability
For medical applications, this research enables more trustworthy AI systems by ensuring LLMs accurately assess when their outputs might be incorrect—critical for patient safety and clinical decision support.
MCQA-Eval: Efficient Confidence Evaluation in NLG with Gold-Standard Correctness Labels