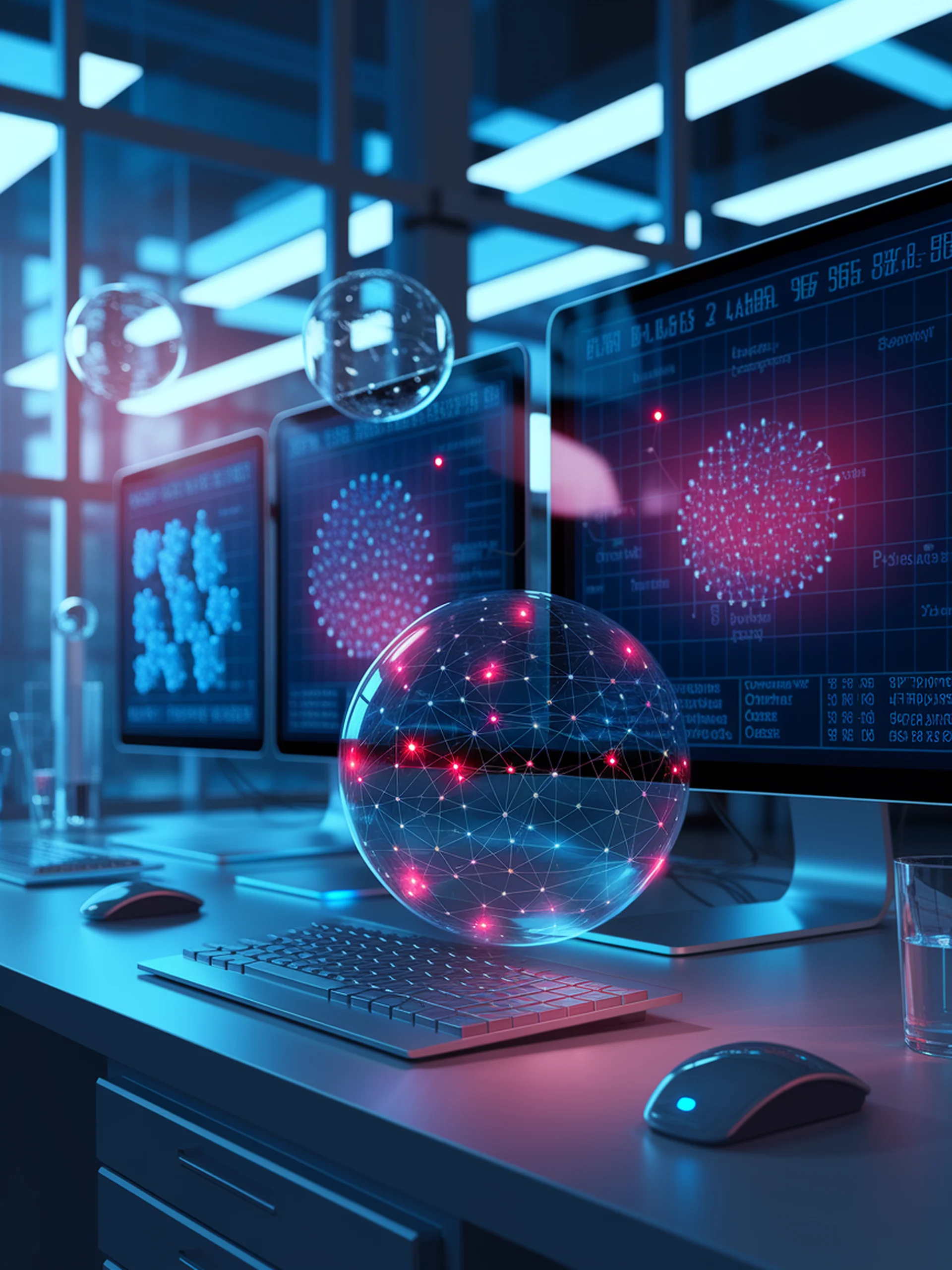
Improving LLM Truthfulness with Uncertainty Detection
Novel density-based approach outperforms existing uncertainty methods
This research introduces a token-level Mahalanobis Distance (MD) technique to detect when language models are uncertain, helping elicit more truthful responses from AI systems.
Key findings:
- Adapts density-based uncertainty methods from classification tasks to generative LLMs
- Achieves state-of-the-art performance in detecting factual errors compared to information-based methods
- Demonstrates effectiveness across multiple benchmarks and model architectures
- Can be efficiently implemented through batch processing and caching
Security implications: This approach significantly improves AI systems' ability to identify when they might be providing false information, a critical requirement for deploying trustworthy LLMs in high-stakes environments where accuracy matters.